CGFNet: 3D Convolution Guided and Multi-scale Volume Fusion Network for fast and robust stereo matching
Pattern Recognit. Lett.(2023)
摘要
Nowadays, although significant progress has been made by convolutional neural network, it is still difficult to realize accurate and robust stereo matching in real time. In this article, we study how to achieve more accurate and robust disparity estimation based on real-time requirement. For this reason, a Multi-scale Volume Fusion (MVF) module was proposed and embedded to improve the matching accuracy. To achieve real-time performance, an innovative way to use 3D convolution is proposed. The 3D convolution is used during training for guidance and supervision, making the inference lightweight. Based on these two structures, we designed an end-to-end stereo matching method called 3D Convolution Guided and Multi-scale Cost Volume Fusion Network (CGFNet). Experimental results showed that our CGFNet has better generalization performance on cross-domain datasets, which achieves more accurate disparity estimation without additional fine tuning process in challenging regions. On KITTI benchmark, CGFNet reached D1-all=1.98% with substantial improvement among the State-Of-The-Art (SOTA) real-time models and runs a pair of images within 38 ms (26 fps). The results are notable when considering both matching accuracy and real-time performance.
更多查看译文
关键词
Robotic vision,Stereo matching,Disparity estimation,Deep learning,Textureless regions
AI 理解论文
溯源树
样例
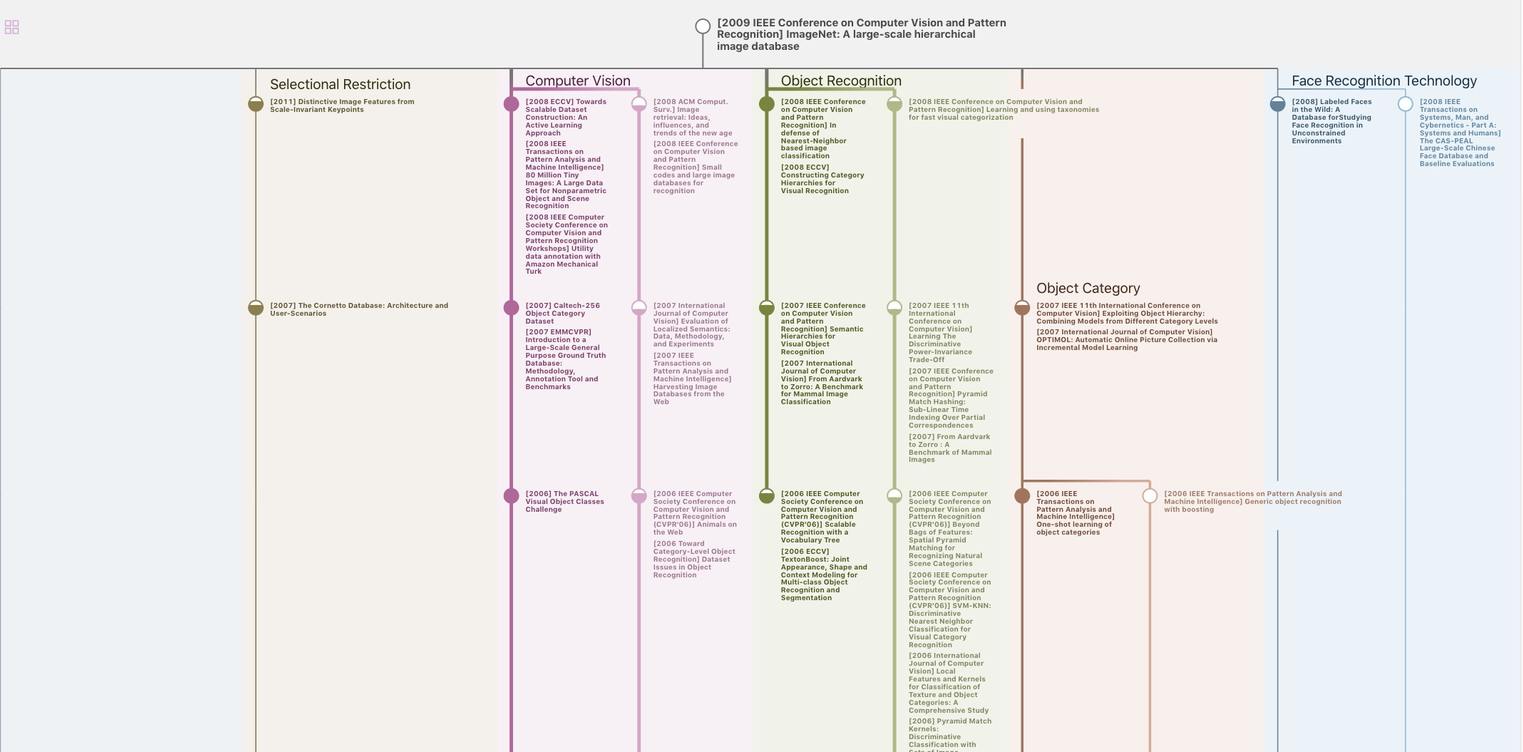
生成溯源树,研究论文发展脉络
Chat Paper
正在生成论文摘要