Modeling and optimization of Graphene Oxide (GO) membranes for nanofiltration with artificial neural networks
JOURNAL OF WATER PROCESS ENGINEERING(2023)
Abstract
It is important to understand the correlation between the nanostructure and membrane performances (water flux and rejection) in membrane separation technology, which is helpful to develop novel membranes. In this study, a back propagation artificial neural network model optimized with genetic algorithms was proposed to predict the nanofiltration performances (water flux and rejection) of GO membranes. The aim is to explore the feature importance of the membrane structure parameters (such as interlayer spacing, Zeta potential, water contact angle, roughness and thickness) and the operating condition (operation pressure) on the membrane properties, so as to provide clues for improving the membrane performances. The obtained results showed that genetic algorithm-back propagation artificial neural network (GABPANN) exhibited more accurate performance to predict the correlation between the parameters (including membrane structure and operation pressure) and the membrane performances according to the published experimental results. Moreover, the GABPANN results indicated that the water contact angle is the most powerful parameter determining the water flux, while the surface charge is the most powerful one determining the rejection of GO membranes. This work provided a novel strategy to efficiently optimize the nanofiltration performance of GO membranes, beneficial for better under-standing and controlling the structural design of GO membranes.
MoreTranslated text
Key words
nanofiltration,graphene oxide,membranes
AI Read Science
Must-Reading Tree
Example
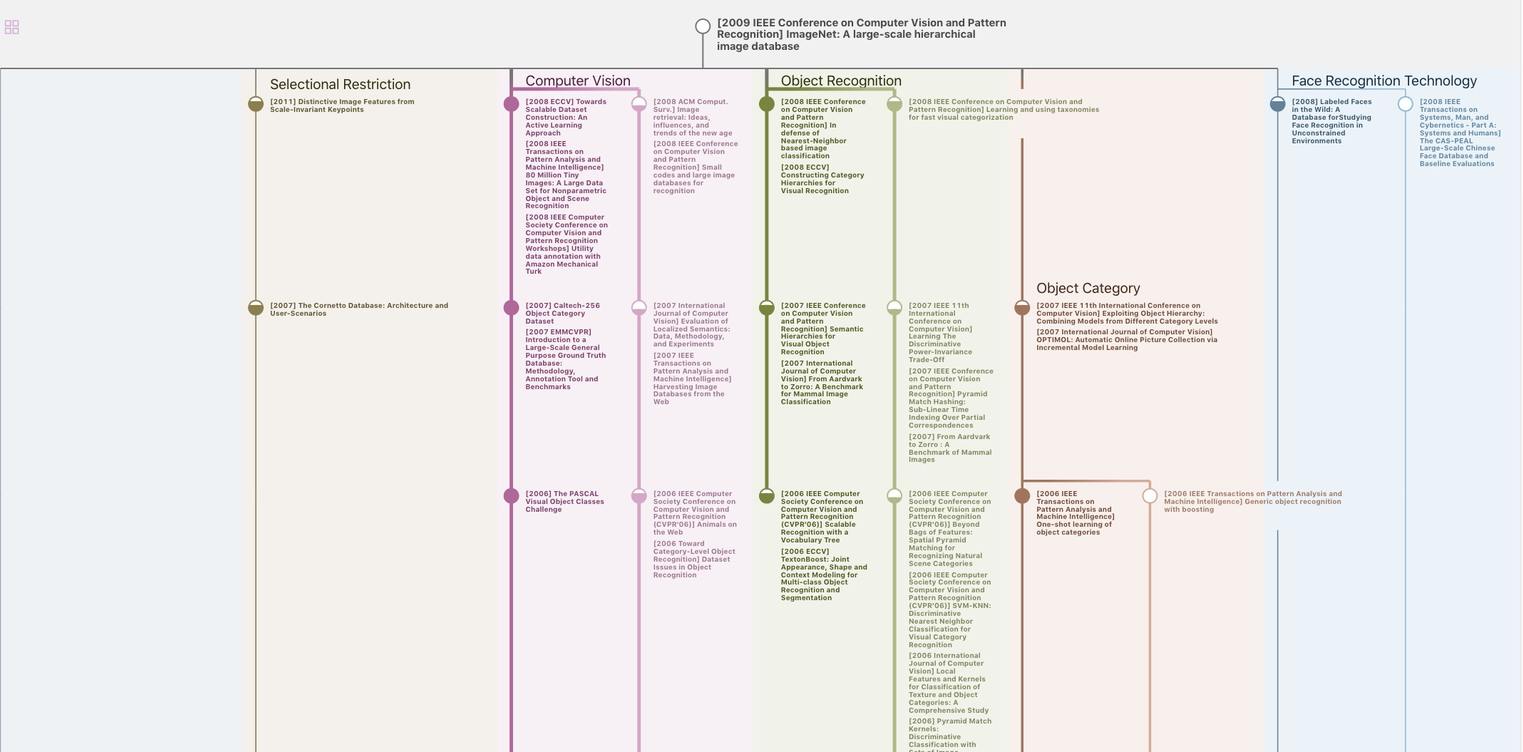
Generate MRT to find the research sequence of this paper
Chat Paper
Summary is being generated by the instructions you defined