Improving object detection by enhancing the effect of localisation quality evaluation on detection confidence
IET COMPUTER VISION(2024)
摘要
The one-stage object detector has been widely applied in many computer vision applications due to its high detection efficiency and simple framework. However, one-stage detectors heavily rely on Non-maximum Suppression to remove the duplicated predictions for the same objects, and the detectors produce detection confidence to measure the quality of those predictions. The localisation quality is an important factor to evaluate the predicted bounding boxes, but its role has not been fully utilised in previous works. To alleviate the problem, the Quality Prediction Block (QPB), a lightweight sub-network, is designed by the authors, which strengthens the effect of localisation quality evaluation on detection confidence by leveraging the features of predicted bounding boxes. The QPB is simple in structure and applies to different forms of detection confidence. Extensive experiments are conducted on the public benchmarks, MS COCO, PASCAL VOC and Berkeley DeepDrive. The results demonstrate the effectiveness of our method in the detectors with various forms of detection confidence. The proposed approach also achieves better performance in the stronger one-stage detectors.
更多查看译文
关键词
object detection,object recognition
AI 理解论文
溯源树
样例
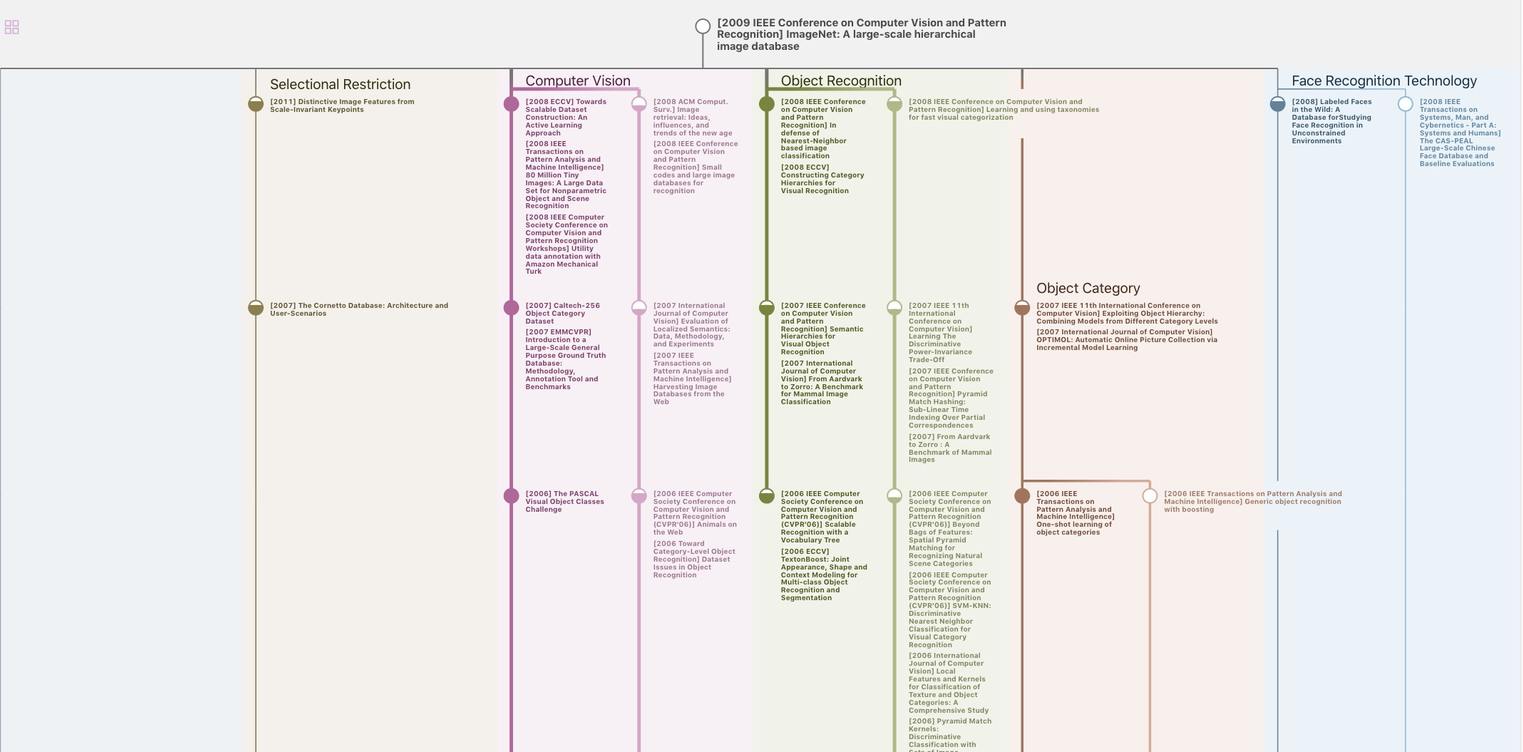
生成溯源树,研究论文发展脉络
Chat Paper
正在生成论文摘要