Experimental analysis of coal gangue identification using visible-near infrared spectroscopy under different working conditions with local and global model
INTERNATIONAL JOURNAL OF COAL PREPARATION AND UTILIZATION(2023)
Abstract
In response to the deficiencies in visible-near infrared spectral identification of coal gangue under different working conditions, this paper employs multiple algorithms to construct local and global recognition models and conducts analysis. Simulating five different working conditions in the laboratory, including different detection angles, illumination angles, detection distances, coal powder content, and moisture content. Each working condition is further divided into four levels. Collecting spectral data of coal and gangue samples. Constructing global and local datasets, which are respectively derived from all levels and single levels under certain working conditions. Training local and global models to identify samples based on machine learning algorithms such as Lightgbm and 1DCNN. The results of the experiment indicate that there are variations in the sample spectra and recognition accuracy based on different working conditions. Furthermore, the local model exhibits lower recognition accuracy and inadequate robustness for other level samples under the same working condition. The global model is more robust and practical, as it achieves higher or equal recognition accuracy for local data compared to the local model. Among the global models, Lightgbm has the best overall performance, with an average F1-score of 99.34% and 97.9% for local and global datasets, respectively. It also has fast training time of 0.75s and recognition time of 1 ms. The Lightgbm global model can accurately identify coal and gangue in various working conditions, making it a reliable solution.
MoreTranslated text
Key words
Coal and gangue, different working conditions, Lightgbm, global model
AI Read Science
Must-Reading Tree
Example
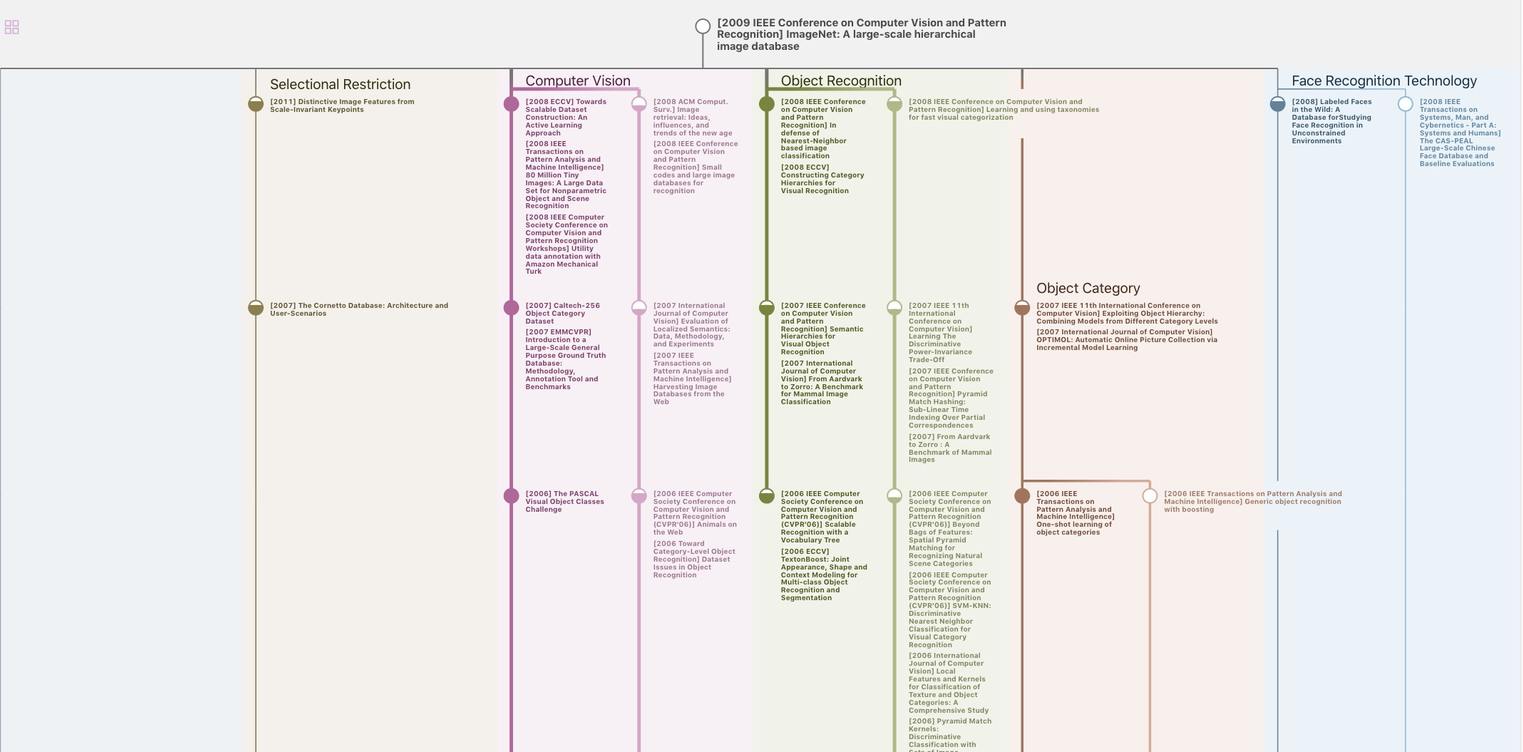
Generate MRT to find the research sequence of this paper
Chat Paper
Summary is being generated by the instructions you defined