Potential Game Based Task Offloading in the High-Speed Railway With Reinforcement Learning
IEEE TRANSACTIONS ON INTELLIGENT TRANSPORTATION SYSTEMS(2023)
摘要
The advanced 5G and mobile edge computing promote the development of the intelligent high-speed railway and enable computing-intensive and latency-sensitive tasks. Mobile edge computing can effectively release the pressure of tasks on on-board computing resources by migrating communication, computing, and storage to edge servers. However, the fluctuation of data transmission and task processing duration exists in practical projects. The current work assumes that the traditional communication model is ideal and that the latency can be derived as a fixed value, which is the most conservative bound. Obviously, there will be some system performance loss because the transmission latency is usually much shorter than this conservative bound. Therefore, an offloading strategy is proposed to maximize the task completion rate, considering the fluctuation of transmission latency and processing time in this paper. First, the stochastic network calculus is adopted to evaluate the fluctuation and probability of the transmission latency. Additionally, the task processing duration is regarded as an exponential distribution that is affected by the computing resource. Then, the task offloading model is generated based on the potential game to maximize the task completion rate. Moreover, the Nash Equilibrium and best response are derived. A reinforcement learning algorithm combined with a game is proposed to reach the Nash Equilibrium and obtain the task offloading strategy. Finally, extensive theoretical analysis and simulations are illustrated to prove the effectiveness of the model.
更多查看译文
关键词
Mobile edge computing,task offloading,high-speed railway,fluctuation of transmission and processing,potential game,stochastic network calculus
AI 理解论文
溯源树
样例
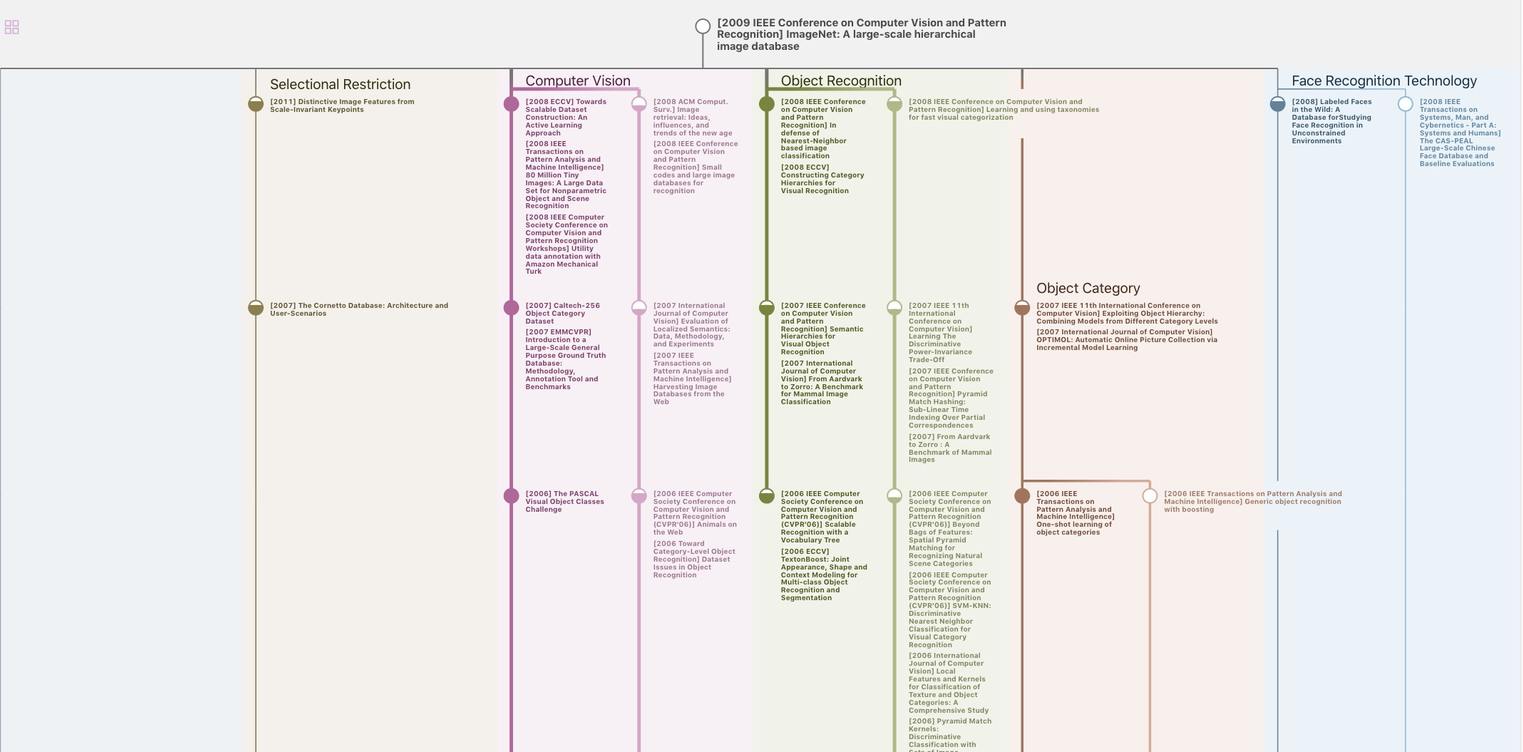
生成溯源树,研究论文发展脉络
Chat Paper
正在生成论文摘要