A fast nondominated sorting-based MOEA with convergence and diversity adjusted adaptively
JOURNAL OF SUPERCOMPUTING(2024)
摘要
In the past few decades, to solve the multi-objective optimization problems, many multi-objective evolutionary algorithms (MOEAs) have been proposed. However, MOEAs have a common difficulty: because the diversity and convergence of solutions are often two conflicting conditions, the balance between the diversity and convergence directly determines the quality of the solutions obtained by the algorithms. Meanwhile, the nondominated sorting method is a costly operation in part Pareto-based MOEAs and needs to be optimized. In this article, we propose a multi-objective evolutionary algorithm framework with convergence and diversity adjusted adaptively. Our contribution is mainly reflected in the following aspects: firstly, we propose a nondominated sorting-based MOEA framework with convergence and diversity adjusted adaptively; secondly, we propose a novel fast nondominated sorting algorithm; thirdly, we propose a convergence improvement strategy and a diversity improvement strategy. In the experiments, we compare our method with several popular MOEAs based on two widely used performance indicators in several multi-objective problem test instances, and the empirical results manifest the proposed method performs the best on most test instances, which further demonstrates that it outperforms all the comparison algorithms.
更多查看译文
关键词
Nondominated sorting,Multi-objective evolutionary algorithm,Convergence,Diversity,Adjusted adaptively
AI 理解论文
溯源树
样例
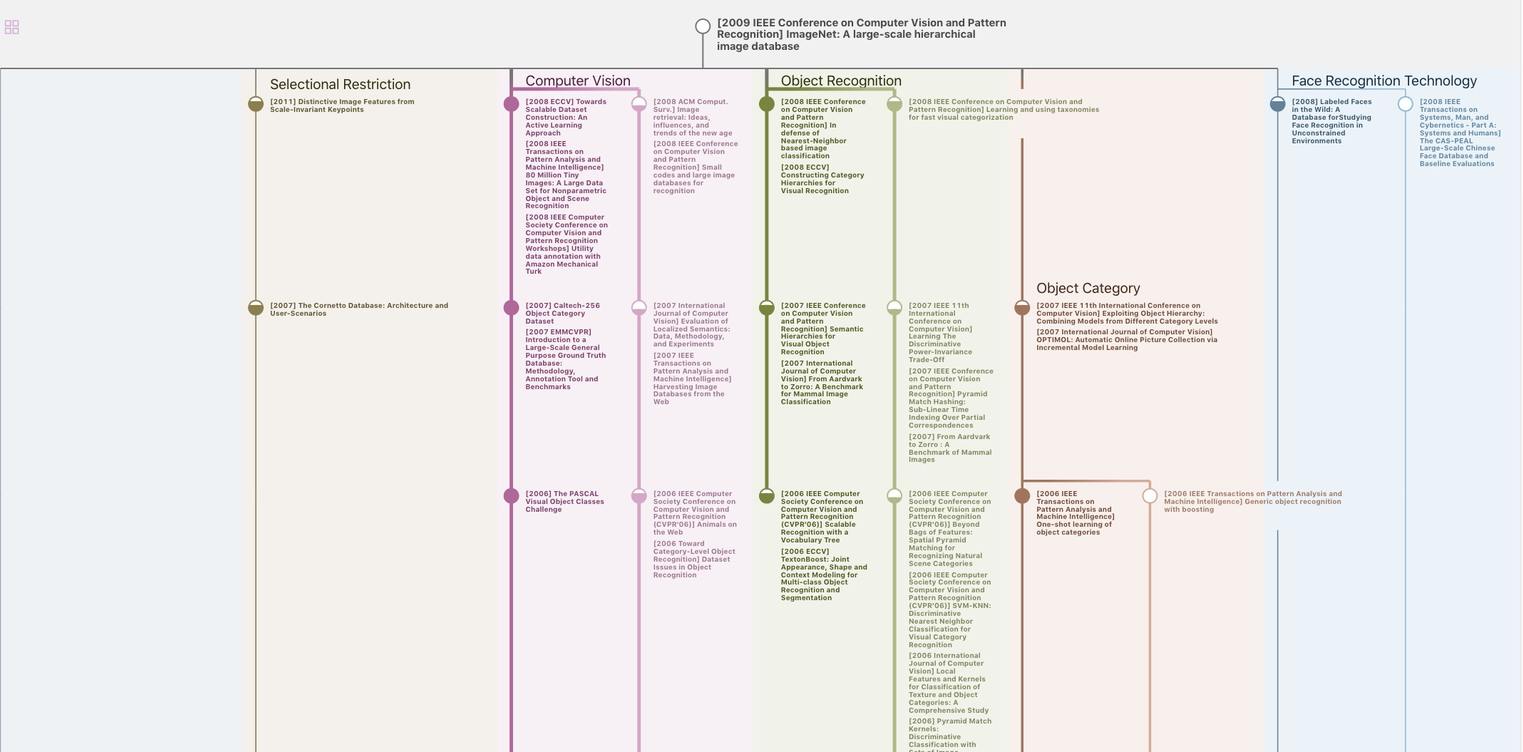
生成溯源树,研究论文发展脉络
Chat Paper
正在生成论文摘要