Evaluation via simulation of statistical corrections for network nonindependence
HEALTH SERVICES AND OUTCOMES RESEARCH METHODOLOGY(2024)
摘要
Social processes and social context are increasingly recognized as key factors shaping health-related behaviors and outcomes. One social process that may be acting within social networks is social influence, in which an individual's characteristic (e.g., specific health behavior) is potentially impacted by the corresponding characteristic of connected individuals in the network. In the health services context, healthcare providers who work together and share patients may influence each other through the knowledge transmission or development of clinical practice norms. Although many statistical techniques assume independence of data points, when analyzing data that may reflect social processes acting across a social network, it is imperative to account for the interdependencies (i.e., non-independence) across individuals. In practice, studies account for nonindependence in the context of estimating bivariate relations (correlations or linear regression) using a variety of analytic methods (some of which have previously been shown to yield biased results). To date, it is unclear which methods yield acceptable false positive rates, unbiased coefficient estimates, and acceptable statistical power, because there have been no systematic simulation studies comparing methods for addressing network nonindependence arising from social influence. To address this gap, we compared eight commonly used methods that purport to account for nonindependence using simulated network data. While results indicated that none of the techniques reduced false positive rates to the predicted (nominal) 0.05 level, random sampling of network nodes was the method that yielded the smallest false positive rates, yet came at a price of reduced statistical power. Further methodological development is needed.
更多查看译文
关键词
Network,Influence,Autocorrelation,Autoregression,Nonindependence,Sampling
AI 理解论文
溯源树
样例
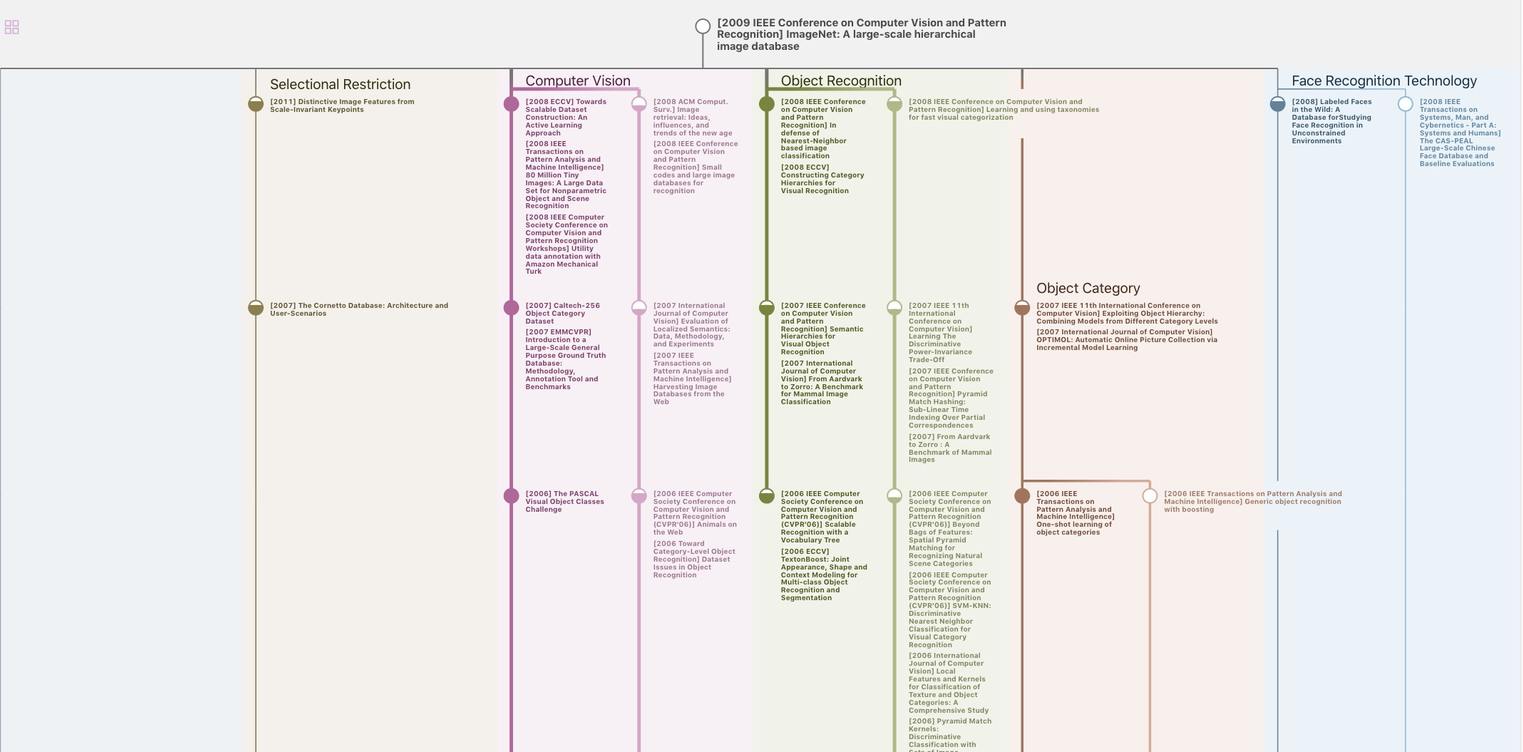
生成溯源树,研究论文发展脉络
Chat Paper
正在生成论文摘要