Mutually Adaptable Learning
IEEE TRANSACTIONS ON EMERGING TOPICS IN COMPUTATIONAL INTELLIGENCE(2024)
摘要
In real-world applications that involve complex data dependencies, it would be essential to proceed with machine learning tasks in an adaptable manner. This article presents a novel Mutually Adaptable Learning (MAL) approach that allows for, on the one hand, extracting the most crucial information from data, and on the other hand, maximally utilizing it through model learning, in a mutually adaptable manner. We elaborate our MAL approach by explaining how it determines the necessity for the adaptation of both features and the learning model, integratively adapts between feature selection and model learning, and optimally achieves the learning objective. To systematically validate the effectiveness of MAL, we conduct comprehensive experiments on challenging learning tasks from two representative domains: spatiotemporal prediction and chaotic behavioral prediction, where the complex data dependencies are general encountered. Results demonstrate that MAL outperforms existing learning methods. Moreover, we show that the formulated objective can be attained under an information-theoretic guarantee. With both empirical and theoretical supports, MAL offers an effective solution to the problem of feature and model adaptation to achieve desired learning objective for given complex tasks.
更多查看译文
关键词
Adaptation models,Task analysis,Data models,Feature extraction,Predictive models,Spatiotemporal phenomena,Data mining,Complex data dependency,Mutually Adaptable Learning (MAL),feature and model adaptation,information-theoretic analysis
AI 理解论文
溯源树
样例
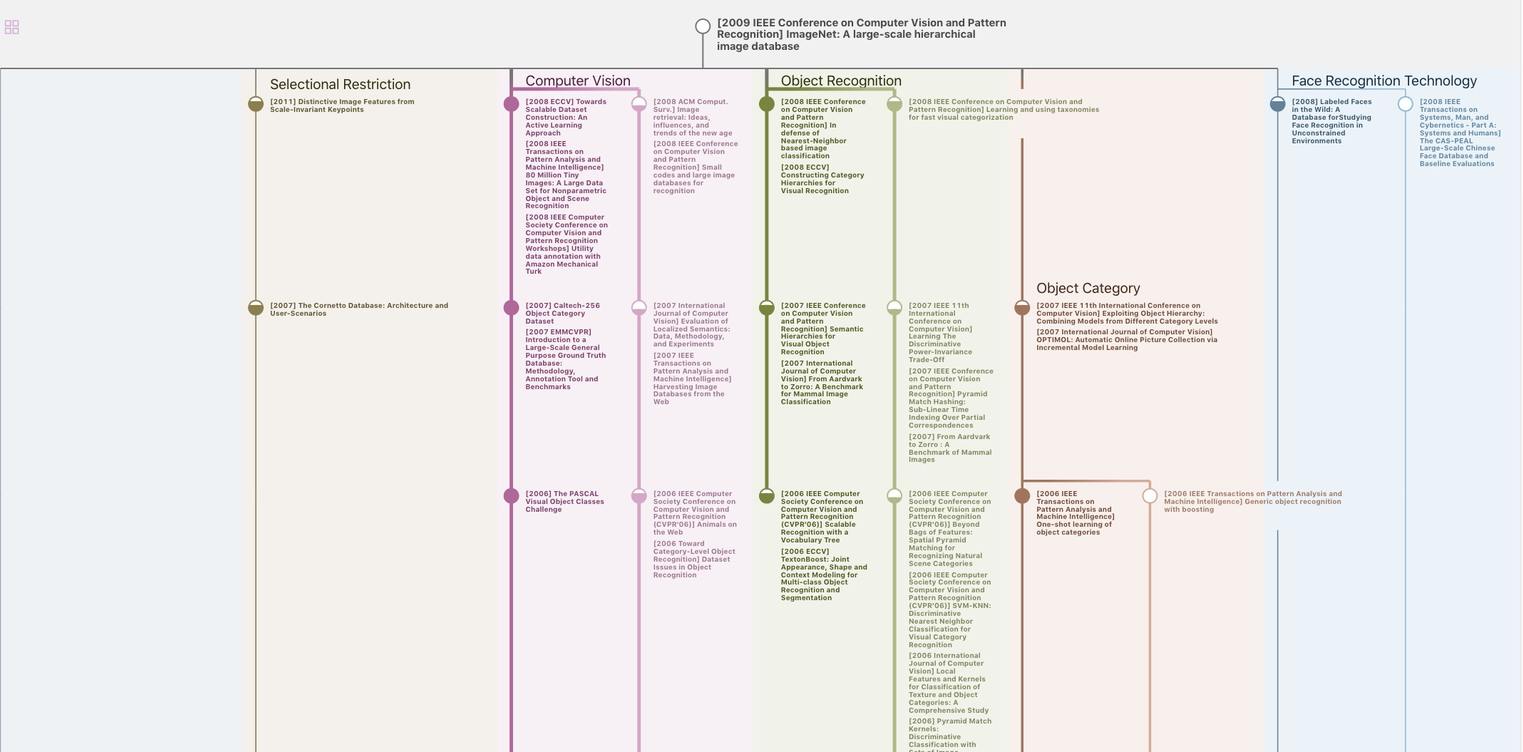
生成溯源树,研究论文发展脉络
Chat Paper
正在生成论文摘要