Reliable and Scalable Routing Under Hybrid SDVN Architecture: A Graph Learning Based Method
IEEE TRANSACTIONS ON INTELLIGENT TRANSPORTATION SYSTEMS(2023)
摘要
Greedy routing efficiently achieves routing solutions for vehicular networks due to its simplicity and reliability. However, the existing greedy routing algorithms have mainly considered simple routing metrics only, e.g., distance based on the local view of an individual vehicle. This consideration is insufficient for analysing dynamic and complicated vehicular communication scenarios which inevitably degrades the overall routing performance. Software-Defined Vehicular Network (SDVN) and Graph Convolutional Network (GCN) can overcome these limitations. Thus, this paper presents a novel GCN-based greedy routing algorithm (NGGRA) in the hybrid SDVN. The SDVN control plane trains the GCN decision model based on the globally collected data. Vehicles with transmission requirements can adopt this model for inferring and making the routing decisions. The proposed node-importance-based graph convolutional network (NiGCN) model analyses multiple correlated metrics to accurately evaluate the dynamic vehicular network is available at: https://github.com/a824899245/NiGCN. Meanwhile, the SDVN architecture offers a global view for model training and routing computation. Extensive simulation results demonstrate that NiGCN outperforms popular GCN models in training efficiency and accuracy. In addition, NGGRA can improve the packet delivery ratio and substantially reduce delay compared with its counterparts.
更多查看译文
关键词
~Software-defined vehicular networks,vehicular ad-hoc networks,routing,deep learning,graph convolutional networks
AI 理解论文
溯源树
样例
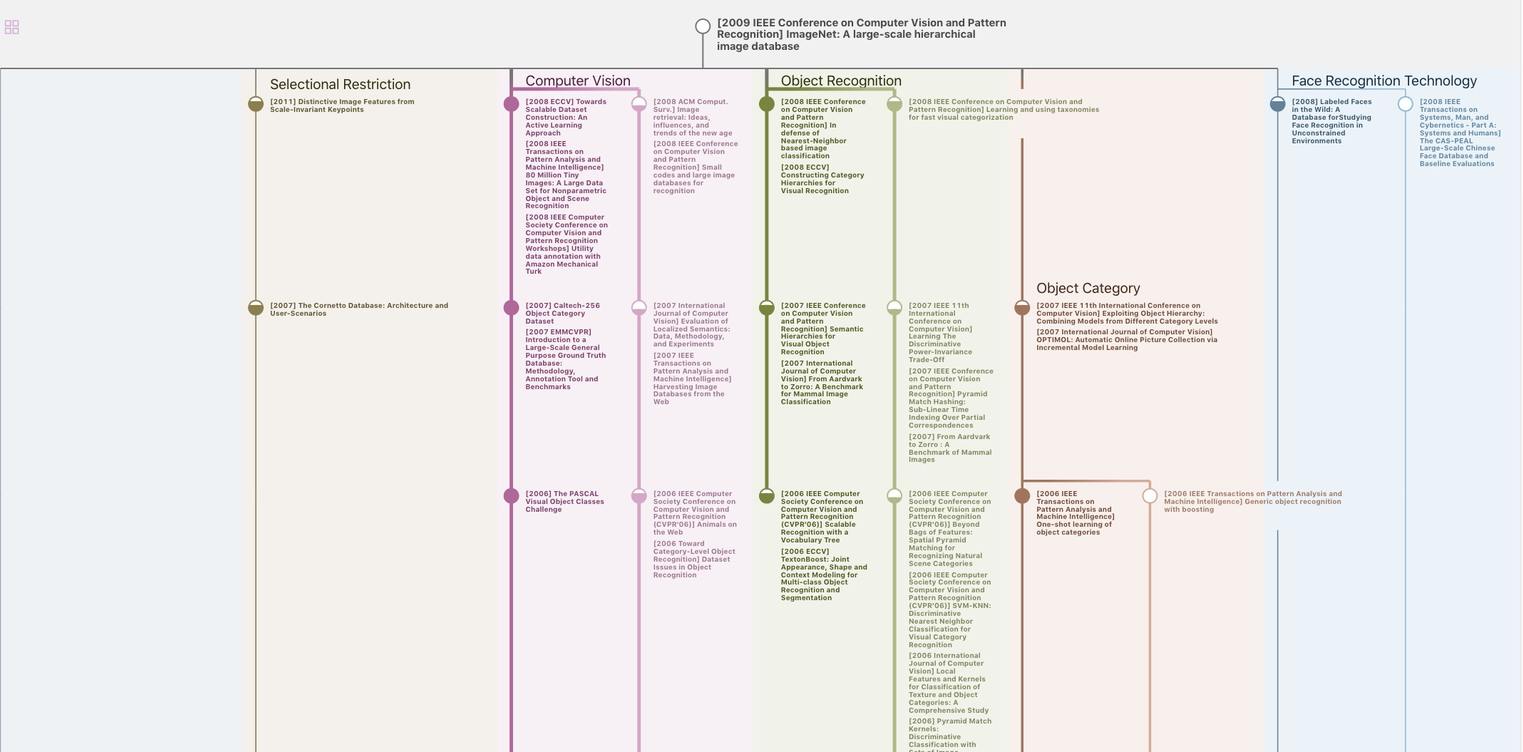
生成溯源树,研究论文发展脉络
Chat Paper
正在生成论文摘要