Takeover quality prediction based on driver physiological state of different cognitive tasks in conditionally automated driving
ADVANCED ENGINEERING INFORMATICS(2023)
摘要
In conditionally automated driving, traffic safety problems would occur if the driver does not properly take over the control authority when the request of automated system arises. Therefore, this study proposes XGBoost learning method considering risk potential field to predict the takeover quality in conditionally automated driving under different levels of cognitive non-driving related tasks (NDRTs). Thirty participants drive on two experimental conditions: manual driving is following an automated driving during which the driver is asked to perform NDRTs. Drivers' physiological features of different cognitive states are exploited to model multi-level takeover quality prediction. This investigation also gives an insight into the main effects of the selected prediction variables on the takeover quality. The proposed model performance within different time windows is assessed using multiple evaluation metrics and compared with other methods. Results show that the prediction accuracy within the time windows of 7-10 s, 5-7 s, 3-5 s and 1-3 s is 0.87, 0.85, 0.85 and 0.90, respectively. The XGBoost model has the best performance of different time windows under each level of takeover quality compared to the other three machine learning models. Our findings can effectively predict the takeover quality and assist automated driving safely in interactions between drivers and automated vehicles.
更多查看译文
关键词
Conditionally automated driving,Takeover quality prediction,Cognitive tasks,Multimodal physiological features,XGBoost and risk potential field
AI 理解论文
溯源树
样例
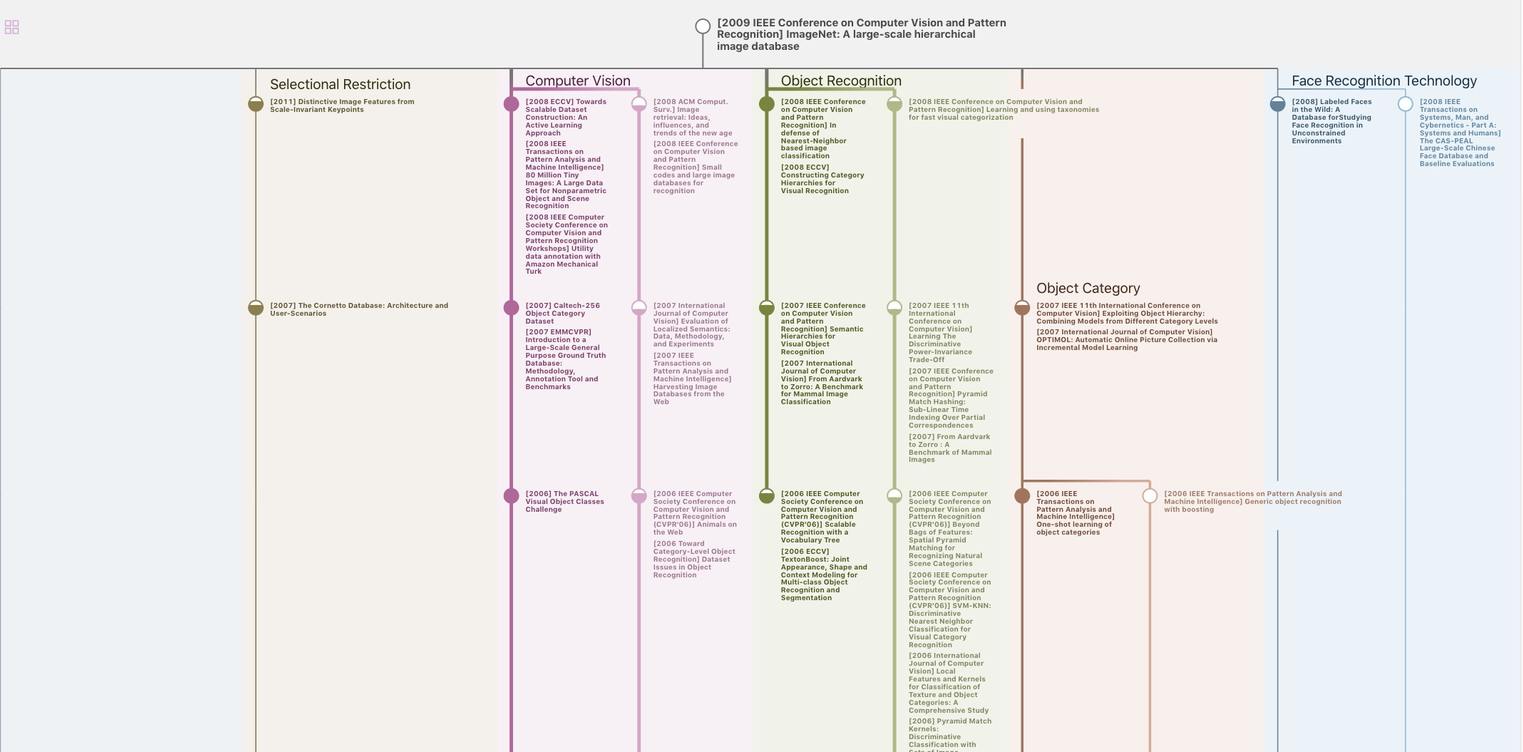
生成溯源树,研究论文发展脉络
Chat Paper
正在生成论文摘要