Cascading and Ensemble Techniques in Deep Learning
ELECTRONICS(2023)
摘要
In this study, we explore the integration of cascading and ensemble techniques in Deep Learning (DL) to improve prediction accuracy on diabetes data. The primary approach involves creating multiple Neural Networks (NNs), each predicting the outcome independently, and then feeding these initial predictions into another set of NN. Our exploration starts from an initial preliminary study and extends to various ensemble techniques including bagging, stacking, and finally cascading. The cascading ensemble involves training a second layer of models on the predictions of the first. This cascading structure, combined with ensemble voting for the final prediction, aims to exploit the strengths of multiple models while mitigating their individual weaknesses. Our results demonstrate significant improvement in prediction accuracy, providing a compelling case for the potential utility of these techniques in healthcare applications, specifically for prediction of diabetes where we achieve compelling model accuracy of 91.5% on the test set on a particular challenging dataset, where we compare thoroughly against many other methodologies.
更多查看译文
关键词
neural networks,cascading,ensemble,diabetes
AI 理解论文
溯源树
样例
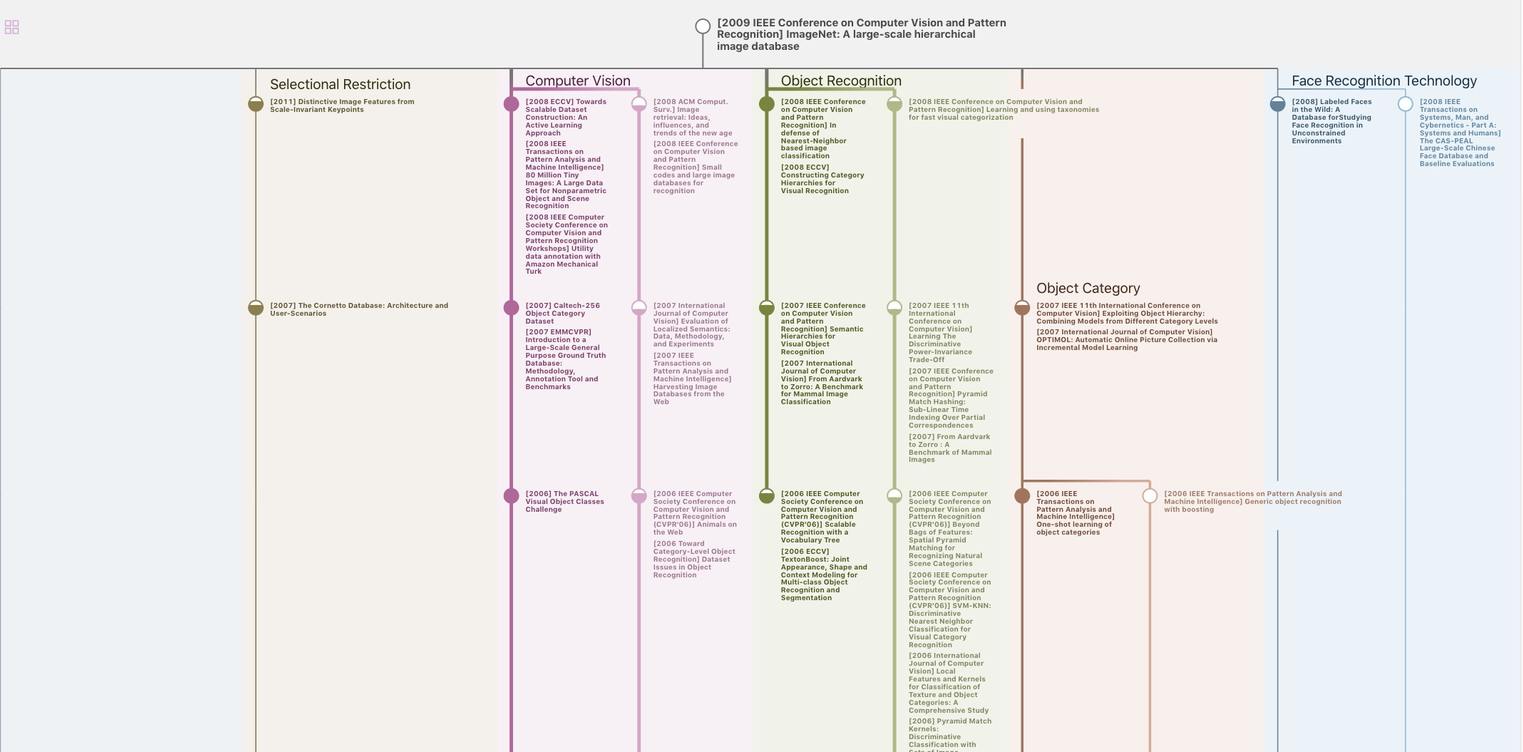
生成溯源树,研究论文发展脉络
Chat Paper
正在生成论文摘要