Water Flow Modeling and Forecast in a Water Branch of Mexico City through ARIMA and Transfer Function Models for Anomaly Detection
WATER(2023)
摘要
Early identification of anomalies (such as leakages or sensor failures) in urban water distribution systems is critical to mitigating water scarcity in cities and is a challenge in water resource management. Several data-driven methods based on machine learning algorithms have been proposed in the literature for leakage detection in urban water distribution systems. Still, most of them are challenging to implement due to their complexity and requirements of vast amounts of reliable data for proper model generation. In addition, the required infrastructure and instrumentation to collect the data needed to train the models could be unaffordable. This paper presents the use and comparison of Autoregressive Integrated Moving Average models and Transfer Function models generated via the Box-Jenkins approach to modeling the water flow in water distribution systems for anomaly detection. The models were fit using water flow data from tanks operating in a branch of the water distribution system of Mexico City. The results showed that both methods helped select the best model type for each variable in the analyzed water branch, with Seasonal ARIMA models achieving a lower mean absolute percentage error than the fitted Transfer Function models. Furthermore, this methodology can be adjusted to different time windows to generate alerts at different rates and does not require a large sample size. The generated anomaly detection models could improve the efficiency of the water distribution system by detecting anomalies such as wrong measurements and water leakages.
更多查看译文
关键词
anomaly detection, ARIMA, transfer function model, urban water branch, leakage detection
AI 理解论文
溯源树
样例
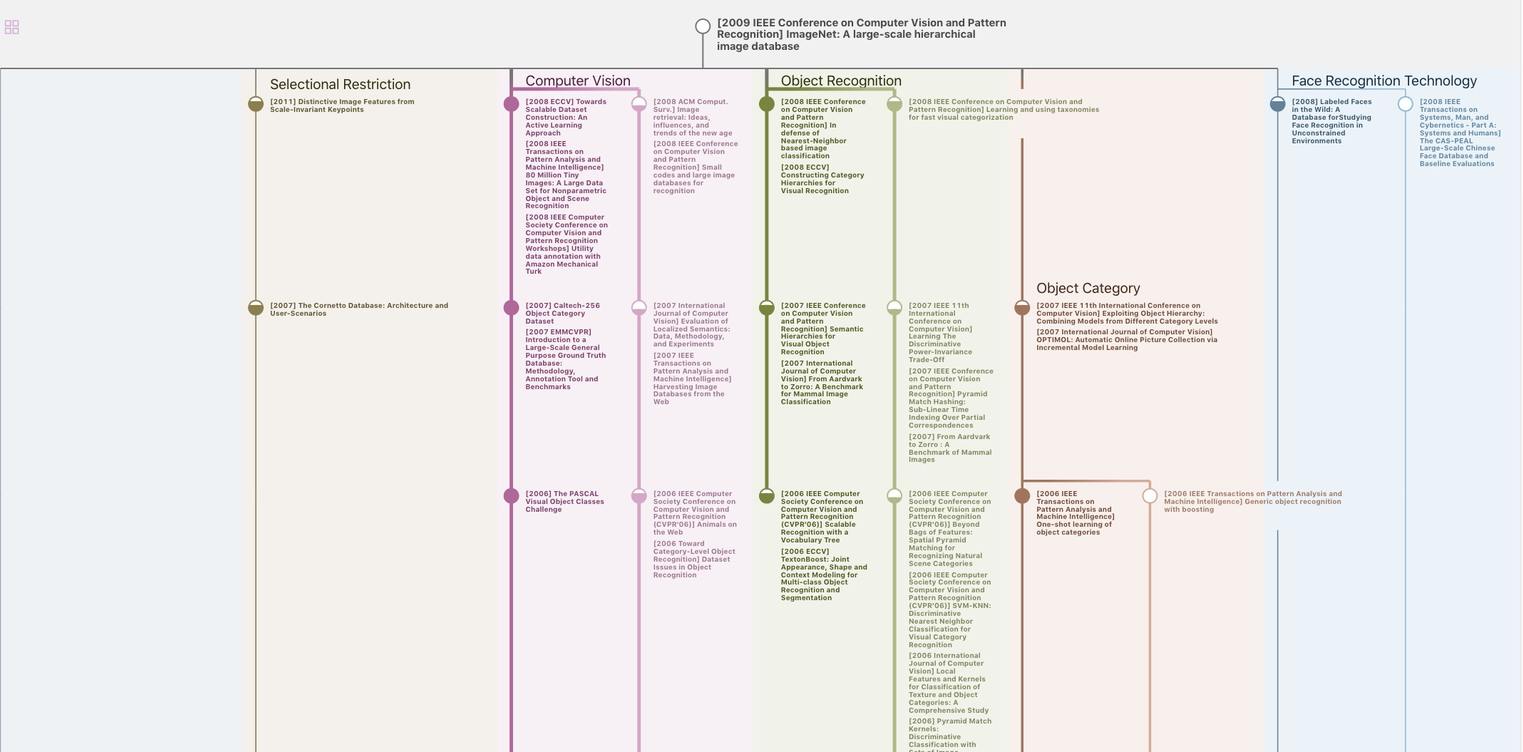
生成溯源树,研究论文发展脉络
Chat Paper
正在生成论文摘要