Image quality improvement in bowtie-filter-equipped cone-beam CT using a dual-domain neural network
Medical physics(2023)
摘要
BackgroundThe bowtie-filter in cone-beam CT (CBCT) causes spatially nonuniform x-ray beam often leading to eclipse artifacts in the reconstructed image. The artifacts are further confounded by the patient scatter, which is therefore patient-dependent as well as system-specific.PurposeIn this study, we propose a dual-domain network for reducing the bowtie-filter-induced artifacts in CBCT images.MethodsIn the projection domain, the network compensates for the filter-induced beam-hardening that are highly related to the eclipse artifacts. The output of the projection-domain network was used for image reconstruction and the reconstructed images were fed into the image-domain network. In the image domain, the network further reduces the remaining cupping artifacts that are associated with the scatter. A single image-domain-only network was also implemented for comparison.ResultsThe proposed approach successfully enhanced soft-tissue contrast with much-reduced image artifacts. In the numerical study, the proposed method decreased perceptual loss and root-mean-square-error (RMSE) of the images by 84.5% and 84.9%, respectively, and increased the structure similarity index measure (SSIM) by 0.26 compared to the original input images on average. In the experimental study, the proposed method decreased perceptual loss and RMSE of the images by 87.2% and 92.1%, respectively, and increased SSIM by 0.58 compared to the original input images on average.ConclusionsWe have proposed a deep-learning-based dual-domain framework to reduce the bowtie-filter artifacts and to increase the soft-tissue contrast in CBCT images. The performance of the proposed method has been successfully demonstrated in both numerical and experimental studies.
更多查看译文
关键词
bowtie-filter,cone-beam CT,dual-domain network,eclipse artifacts
AI 理解论文
溯源树
样例
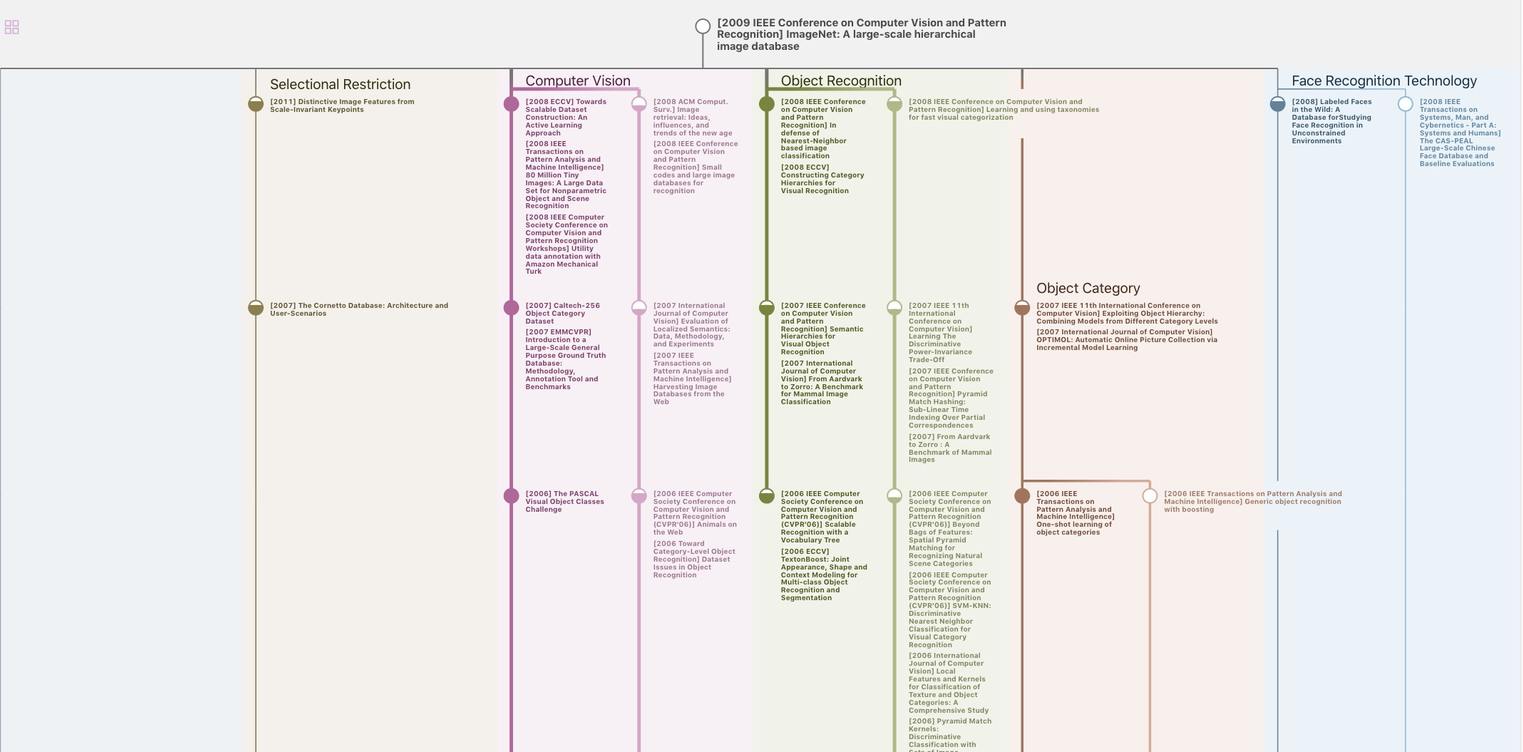
生成溯源树,研究论文发展脉络
Chat Paper
正在生成论文摘要