Using machine learning to predict bleeding after cardiac surgery
EUROPEAN JOURNAL OF CARDIO-THORACIC SURGERY(2023)
摘要
OBJECTIVES The primary objective was to predict bleeding after cardiac surgery with machine learning using the data from the Australia New Zealand Society of Cardiac and Thoracic Surgeons Cardiac Surgery Database, cardiopulmonary bypass perfusion database, intensive care unit database and laboratory results.METHODS We obtained surgical, perfusion, intensive care unit and laboratory data from a single Australian tertiary cardiac surgical hospital from February 2015 to March 2022 and included 2000 patients undergoing cardiac surgery. We trained our models to predict either the Papworth definition or Dyke et al.'s universal definition of perioperative bleeding. Our primary outcome was the performance of our machine learning algorithms using sensitivity, specificity, positive and negative predictive values, accuracy, area under receiver operating characteristics curve (AUROC) and area under precision-recall curve (AUPRC).RESULTS Of the 2000 patients undergoing cardiac surgery, 13.3% (226/2000) had bleeding using the Papworth definition and 17.2% (343/2000) had moderate to massive bleeding using Dyke et al.'s definition. The best-performing model based on AUPRC was the Ensemble Voting Classifier model for both Papworth (AUPRC 0.310, AUROC 0.738) and Dyke definitions of bleeding (AUPRC 0.452, AUROC 0.797).CONCLUSIONS Machine learning can incorporate routinely collected data from various datasets to predict bleeding after cardiac surgery. Severe post-surgical cardiac bleeding is common and contributes to increased morbidity and mortality for patients undergoing cardiac surgery [1, 2].
更多查看译文
关键词
cardiac surgery,machine learning,bleeding
AI 理解论文
溯源树
样例
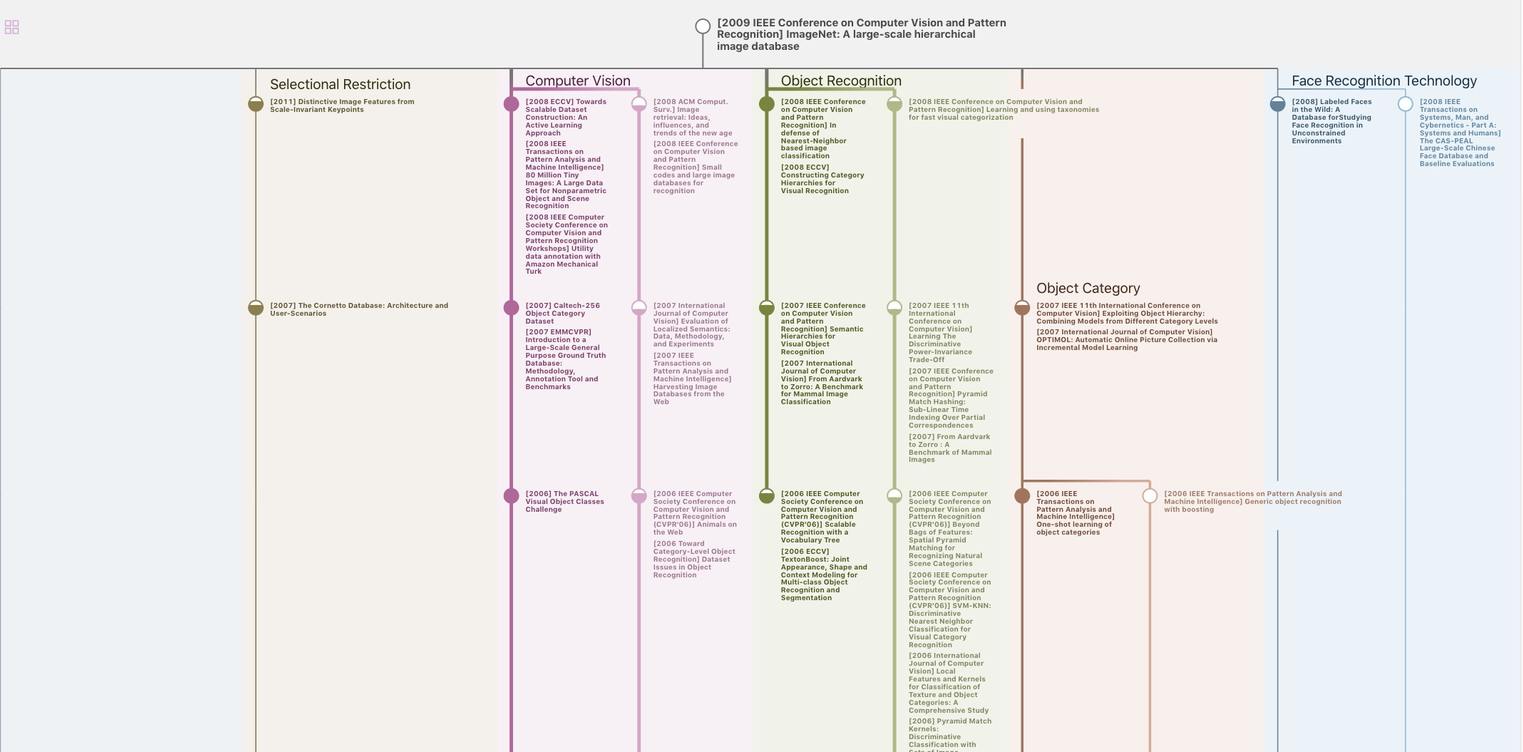
生成溯源树,研究论文发展脉络
Chat Paper
正在生成论文摘要