Uncertainty Quantification in Deep Learning Based Kalman Filters
ICASSP 2024 - 2024 IEEE International Conference on Acoustics, Speech and Signal Processing (ICASSP)(2023)
摘要
Various algorithms combine deep neural networks (DNNs) and Kalman filters (KFs) to learn from data to track in complex dynamics. Unlike classic KFs, DNN-based systems do not naturally provide the error covariance alongside their estimate, which is of great importance in some applications, e.g., navigation. To bridge this gap, in this work we study error covariance extraction in DNN-aided KFs. We examine three main approaches that are distinguished by the ability to associate internal features with meaningful KF quantities such as the Kalman gain (KG) and prior covariance. We identify the differences between these approaches in their requirements and their effect on the training of the system. Our numerical study demonstrates that the above approaches allow DNN-aided KFs to extract error covariance, with most accurate error prediction provided by model-based/data-driven designs.
更多查看译文
关键词
Kalman filter,deep learning,uncertainty
AI 理解论文
溯源树
样例
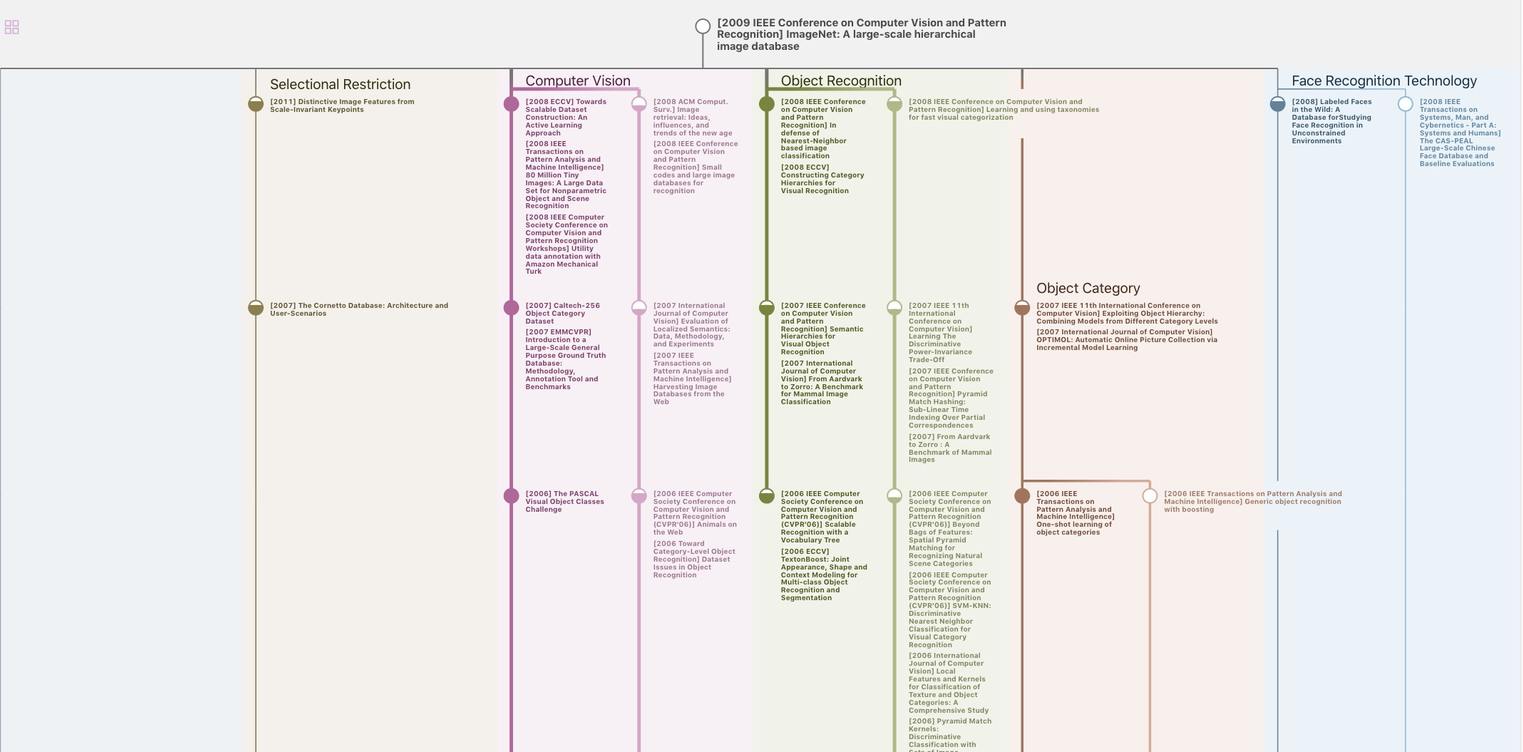
生成溯源树,研究论文发展脉络
Chat Paper
正在生成论文摘要