SPAHM(a,b): Encoding the Density Information from Guess Hamiltonian in Quantum Machine Learning Representations.
Journal of chemical theory and computation(2024)
摘要
Recently, we introduced a class of molecular representations for kernel-based regression methods─the spectrum of approximated Hamiltonian matrices (SPAHM)─that takes advantage of lightweight one-electron Hamiltonians traditionally used as a self-consistent field initial guess. The original SPAHM variant is built from occupied-orbital energies (i.e., eigenvalues) and naturally contains all of the information about nuclear charges, atomic positions, and symmetry requirements. Its advantages were demonstrated on data sets featuring a wide variation of charge and spin, for which traditional structure-based representations commonly fail. SPAHM(a,b), as introduced here, expand the eigenvalue SPAHM into local and transferable representations. They rely upon one-electron density matrices to build fingerprints from atomic and bond density overlap contributions inspired from preceding state-of-the-art representations. The performance and efficiency of SPAHM(a,b) is assessed on the predictions for data sets of prototypical organic molecules (QM7) of different charges and azoheteroarene dyes in an excited state. Overall, both SPAHM(a) and SPAHM(b) outperform state-of-the-art representations on difficult prediction tasks such as the atomic properties of charged open-shell species and of π-conjugated systems.
更多查看译文
关键词
quantum machine,density information,representations,machine learning
AI 理解论文
溯源树
样例
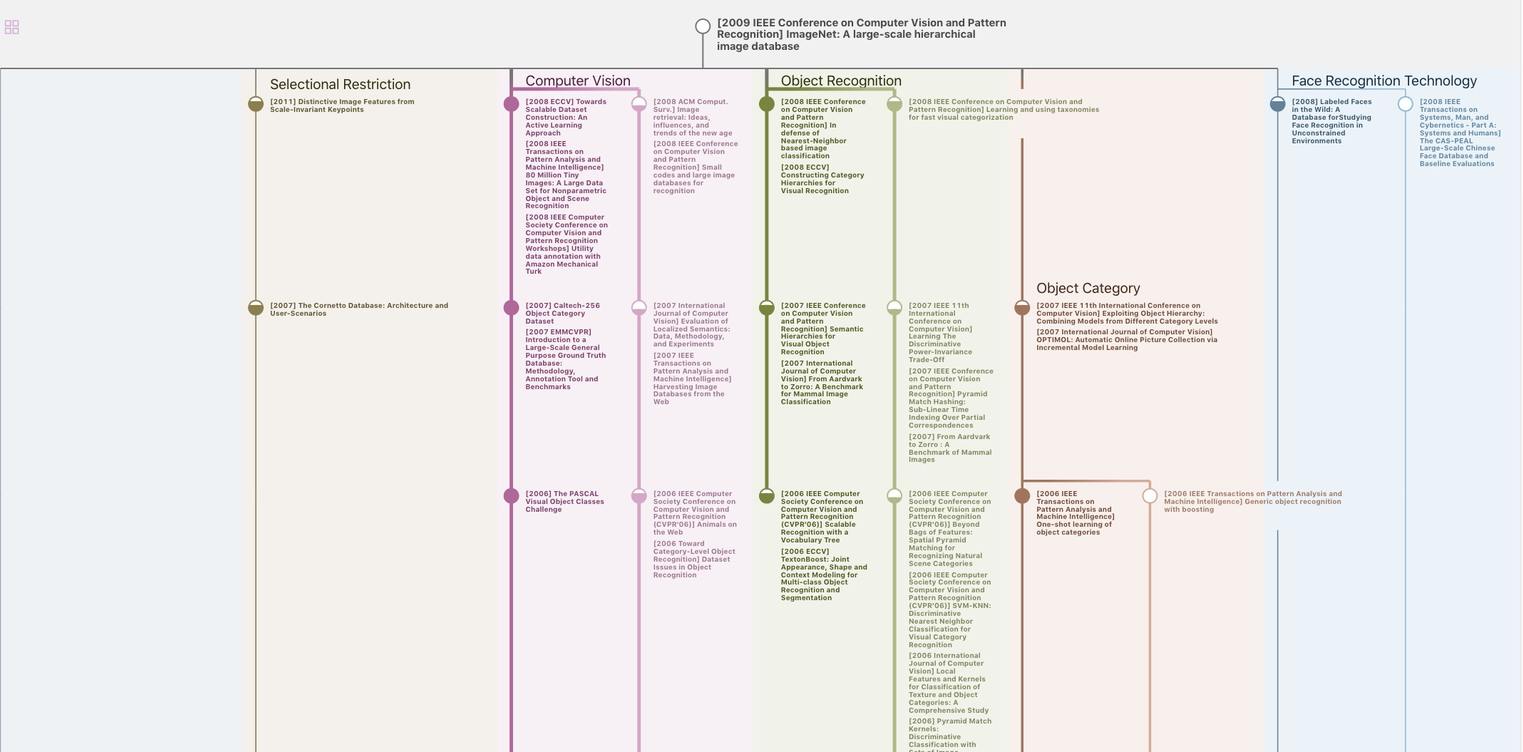
生成溯源树,研究论文发展脉络
Chat Paper
正在生成论文摘要