Reinforcement Learning of Action and Query Policies With LTL Instructions Under Uncertain Event Detector
IEEE ROBOTICS AND AUTOMATION LETTERS(2023)
摘要
Reinforcement learning (RL) with linear temporal logic (LTL) objectives can allow robots to carry out symbolic event plans in unknown environments. Most existing methods assume that the event detector can accurately map environmental states to symbolic events; however, uncertainty is inevitable for real-world event detectors. Such uncertainty in an event detector generates multiple branching possibilities on LTL instructions, confusing action decisions. Moreover, the queries to the uncertain event detector, necessary for the task's progress, may increase the uncertainty further. To cope with those issues, we propose an RL framework, Learning Action and Query over Belief LTL (LAQBL), to learn an agent that can consider the diversity of LTL instructions due to uncertain event detection while avoiding task failure due to the unnecessary event-detection query. Our framework simultaneously learns 1) an embedding of belief LTL, which is multiple branching possibilities on LTL instructions using a graph neural network, 2) an action policy, and 3) a query policy that decides whether or not to query for the event detector. Simulations in a 2D grid world and image-input robotic inspection environments show that our method successfully learns actions to follow LTL instructions even with uncertain event detectors.
更多查看译文
关键词
Reinforcement learning,Proposals,planning under uncertainty
AI 理解论文
溯源树
样例
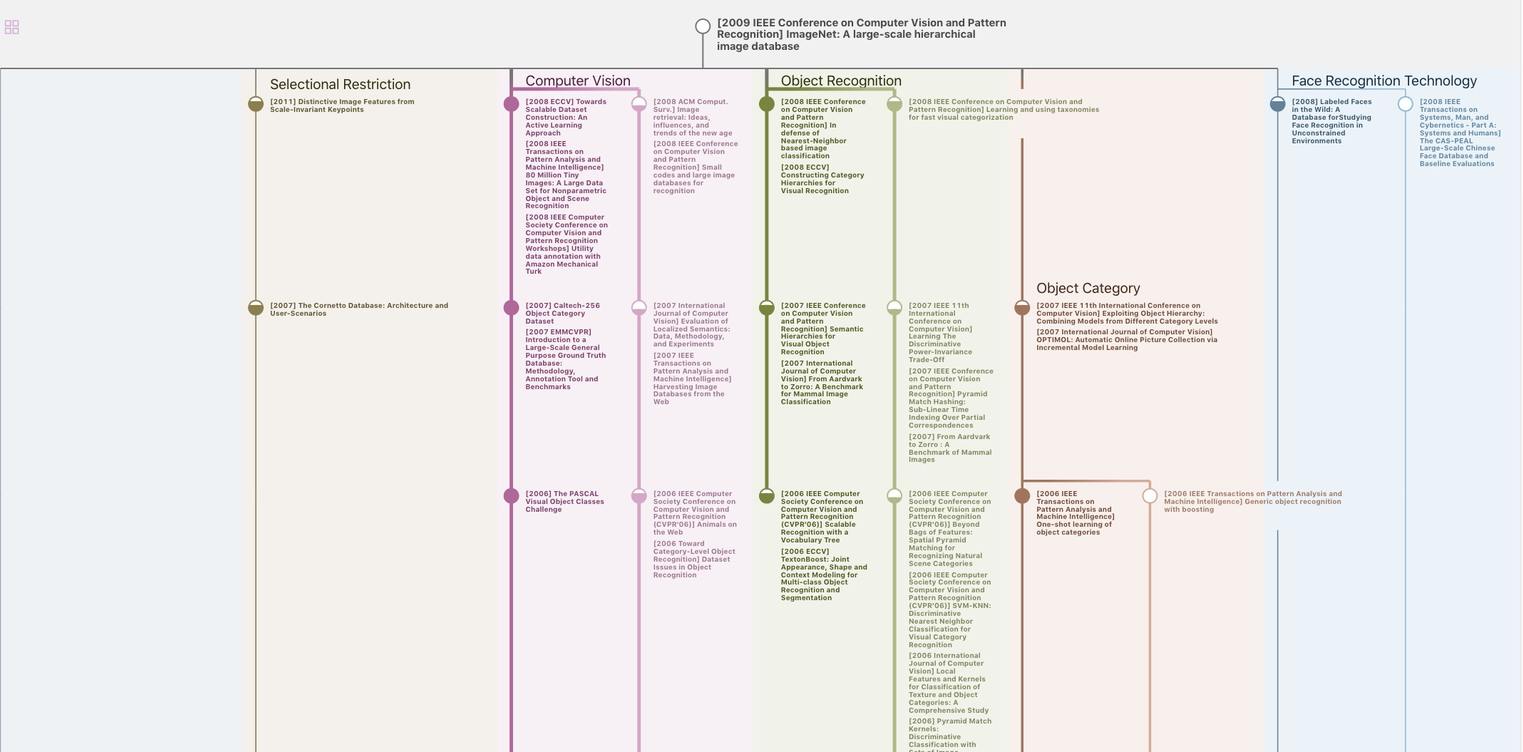
生成溯源树,研究论文发展脉络
Chat Paper
正在生成论文摘要