DMKD: Improving Feature-based Knowledge Distillation for Object Detection Via Dual Masking Augmentation
ICASSP 2024 - 2024 IEEE International Conference on Acoustics, Speech and Signal Processing (ICASSP)(2023)
摘要
Recent mainstream masked distillation methods function by reconstructing selectively masked areas of a student network from the feature map of its teacher counterpart. In these methods, the masked regions need to be properly selected, such that reconstructed features encode sufficient discrimination and representation capability like the teacher feature. However, previous masked distillation methods only focus on spatial masking, making the resulting masked areas biased towards spatial importance without encoding informative channel clues. In this study, we devise a Dual Masked Knowledge Distillation (DMKD) framework which can capture both spatially important and channel-wise informative clues for comprehensive masked feature reconstruction. More specifically, we employ dual attention mechanism for guiding the respective masking branches, leading to reconstructed feature encoding dual significance. Furthermore, fusing the reconstructed features is achieved by self-adjustable weighting strategy for effective feature distillation. Our experiments on object detection task demonstrate that the student networks achieve performance gains of 4.1% and 4.3% with the help of our method when RetinaNet and Cascade Mask R-CNN are respectively used as the teacher networks, while outperforming the other state-of-the-art distillation methods.
更多查看译文
关键词
Masked Knowledge Distillation,Dual Attention,Masked Feature Reconstruction,Object Detection
AI 理解论文
溯源树
样例
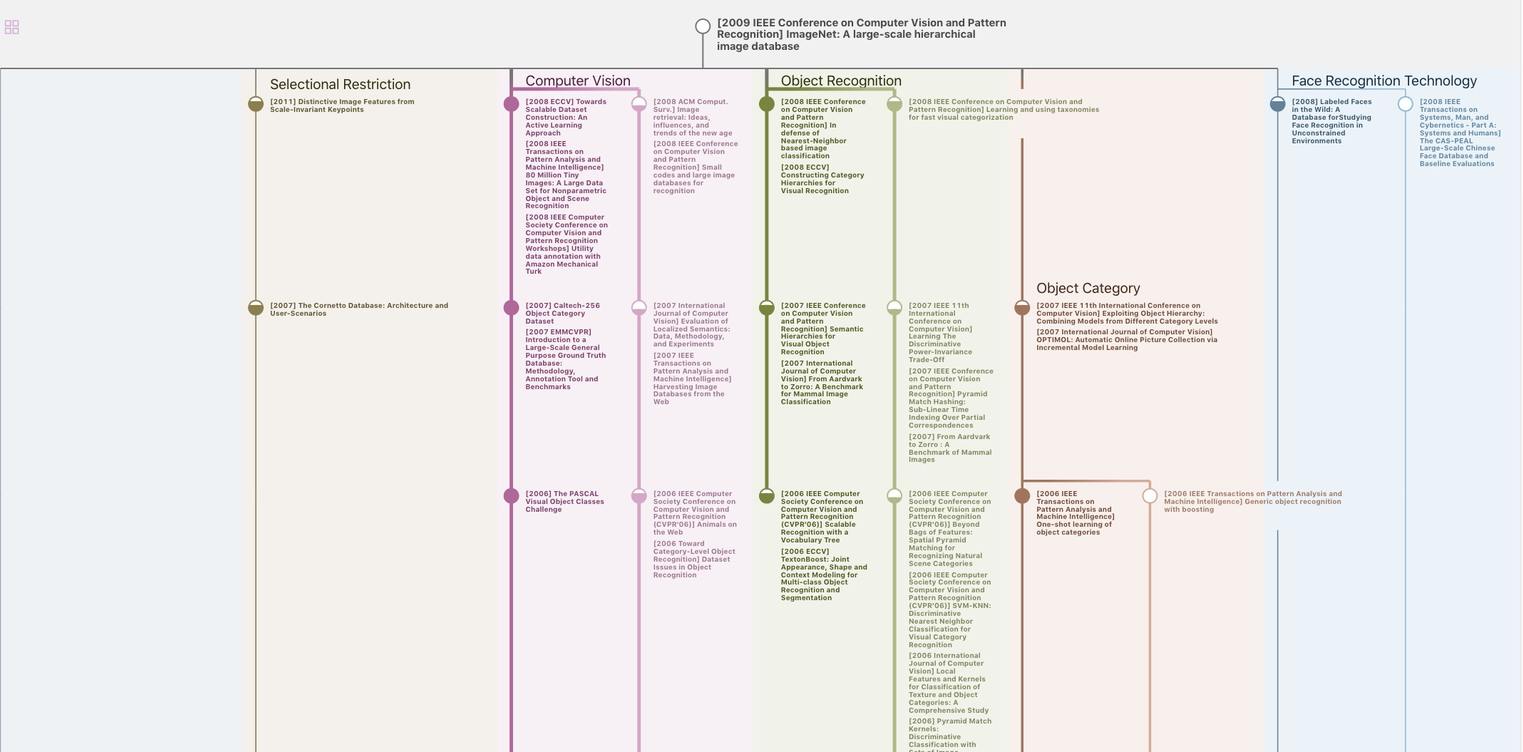
生成溯源树,研究论文发展脉络
Chat Paper
正在生成论文摘要