RLSynC: Offline-Online Reinforcement Learning for Synthon Completion
arxiv(2023)
摘要
Retrosynthesis is the process of determining the set of reactant molecules
that can react to form a desired product. Semi-template-based retrosynthesis
methods, which imitate the reverse logic of synthesis reactions, first predict
the reaction centers in the products, and then complete the resulting synthons
back into reactants. We develop a new offline-online reinforcement learning
method RLSynC for synthon completion in semi-template-based methods. RLSynC
assigns one agent to each synthon, all of which complete the synthons by
conducting actions step by step in a synchronized fashion. RLSynC learns the
policy from both offline training episodes and online interactions, which
allows RLSynC to explore new reaction spaces. RLSynC uses a standalone forward
synthesis model to evaluate the likelihood of the predicted reactants in
synthesizing a product, and thus guides the action search. Our results
demonstrate that RLSynC can outperform state-of-the-art synthon completion
methods with improvements as high as 14.9
synthesis planning.
更多查看译文
AI 理解论文
溯源树
样例
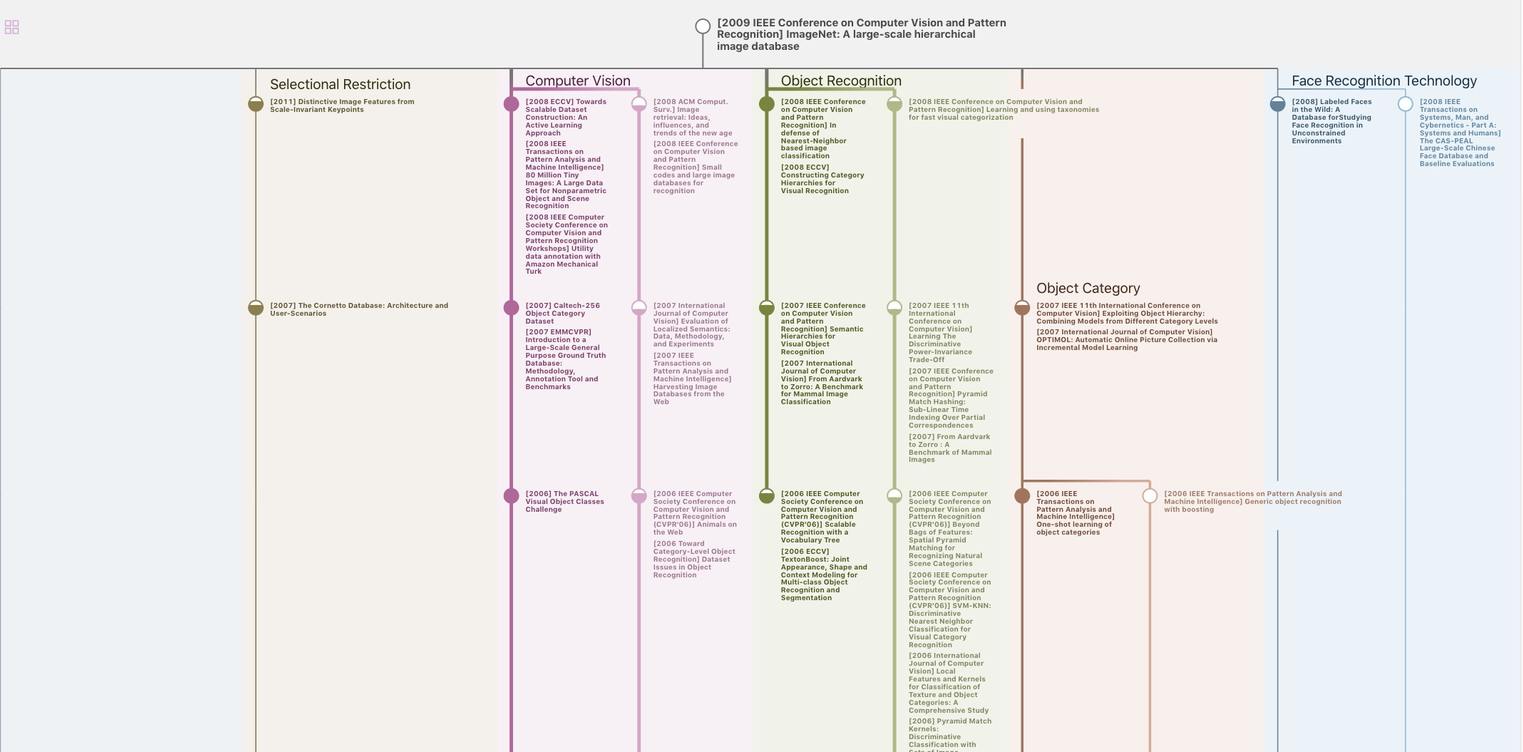
生成溯源树,研究论文发展脉络
Chat Paper
正在生成论文摘要