Fast and Resource-Efficient Object Tracking on Edge Devices: A Measurement Study
CoRR(2023)
摘要
Object tracking is an important functionality of edge video analytic systems and services. Multi-object tracking (MOT) detects the moving objects and tracks their locations frame by frame as real scenes are being captured into a video. However, it is well known that real time object tracking on the edge poses critical technical challenges, especially with edge devices of heterogeneous computing resources. This paper examines the performance issues and edge-specific optimization opportunities for object tracking. We will show that even the well trained and optimized MOT model may still suffer from random frame dropping problems when edge devices have insufficient computation resources. We present several edge specific performance optimization strategies, collectively coined as EMO, to speed up the real time object tracking, ranging from window-based optimization to similarity based optimization. Extensive experiments on popular MOT benchmarks demonstrate that our EMO approach is competitive with respect to the representative methods for on-device object tracking techniques in terms of run-time performance and tracking accuracy. EMO is released on Github at https://github.com/git-disl/EMO.
更多查看译文
关键词
edge devices,tracking,resource-efficient
AI 理解论文
溯源树
样例
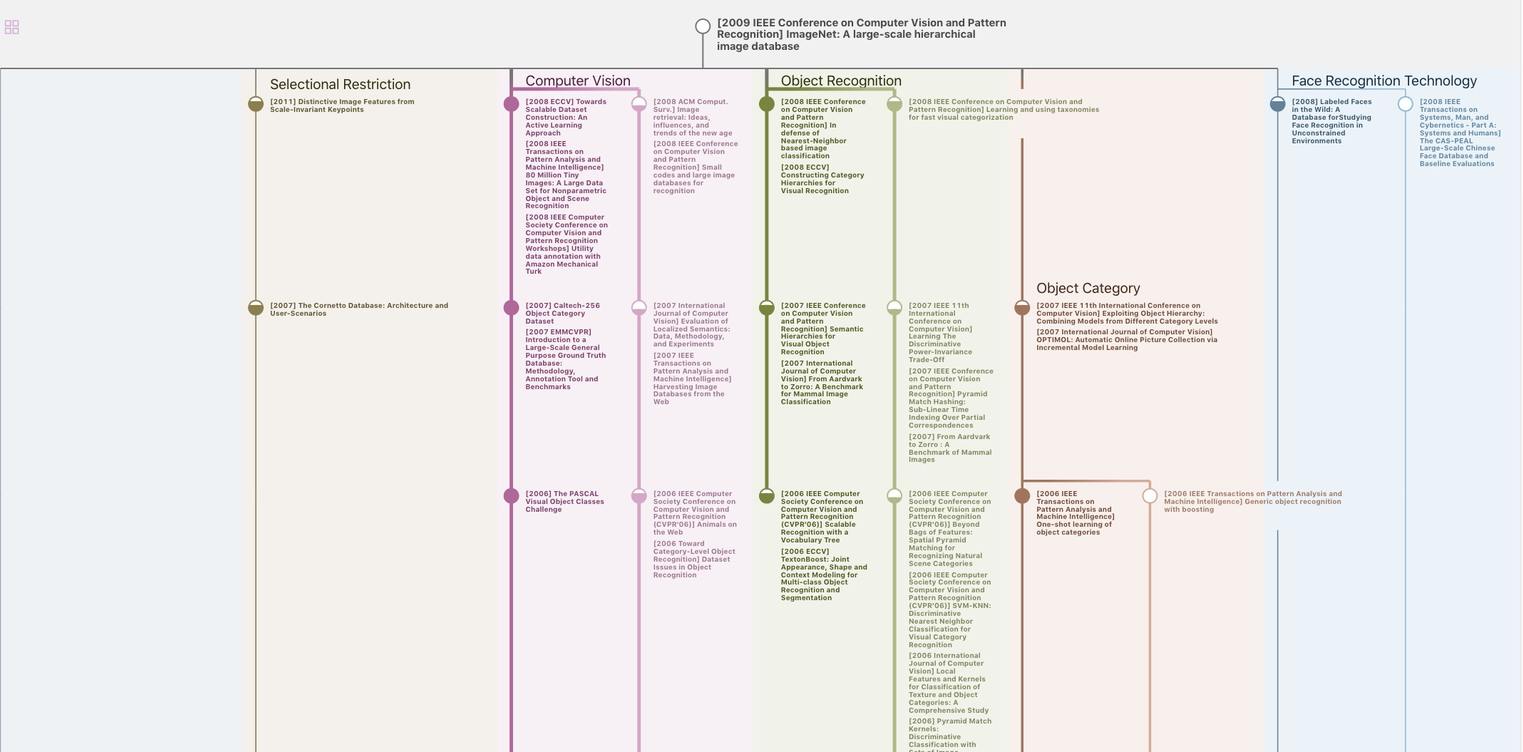
生成溯源树,研究论文发展脉络
Chat Paper
正在生成论文摘要