Directionality-Aware Mixture Model Parallel Sampling for Efficient Linear Parameter Varying Dynamical System Learning
IEEE ROBOTICS AND AUTOMATION LETTERS(2024)
Abstract
The Linear Parameter Varying Dynamical System (LPV-DS) is an effective approach that learns stable, time-invariant motion policies using statistical modeling and semi-definite optimization to encode complex motions for reactive robot control. Despite its strengths, the LPV-DS learning approach faces challenges in achieving high model accuracy without compromising computational efficiency. To address this, we introduce the Directionality-Aware Mixture Model (DAMM), a novel statistical model that applies the Riemannian metric on the n-sphere to efficiently blend non-Euclidean directional data with Euclidean states. Additionally, we develop a hybrid Markov chain Monte Carlo technique that combines Gibbs Sampling with Split/Merge Proposal, allowing for parallel computation to speed up inference. Extensive empirical tests demonstrate that LPV-DS integrated with DAMM achieves higher reproduction accuracy, better computational efficiency, and near real-time/online learning compared to standard estimation methods on various datasets. Lastly, we demonstrate its suitability for incrementally learning multi-behavior policies in real-world robot experiments.
MoreTranslated text
Key words
Manifolds,Trajectory,Measurement,Mixture models,Computational modeling,Vectors,Dynamical systems,Learning from demonstration,imitation learning,machine learning for robot control
AI Read Science
Must-Reading Tree
Example
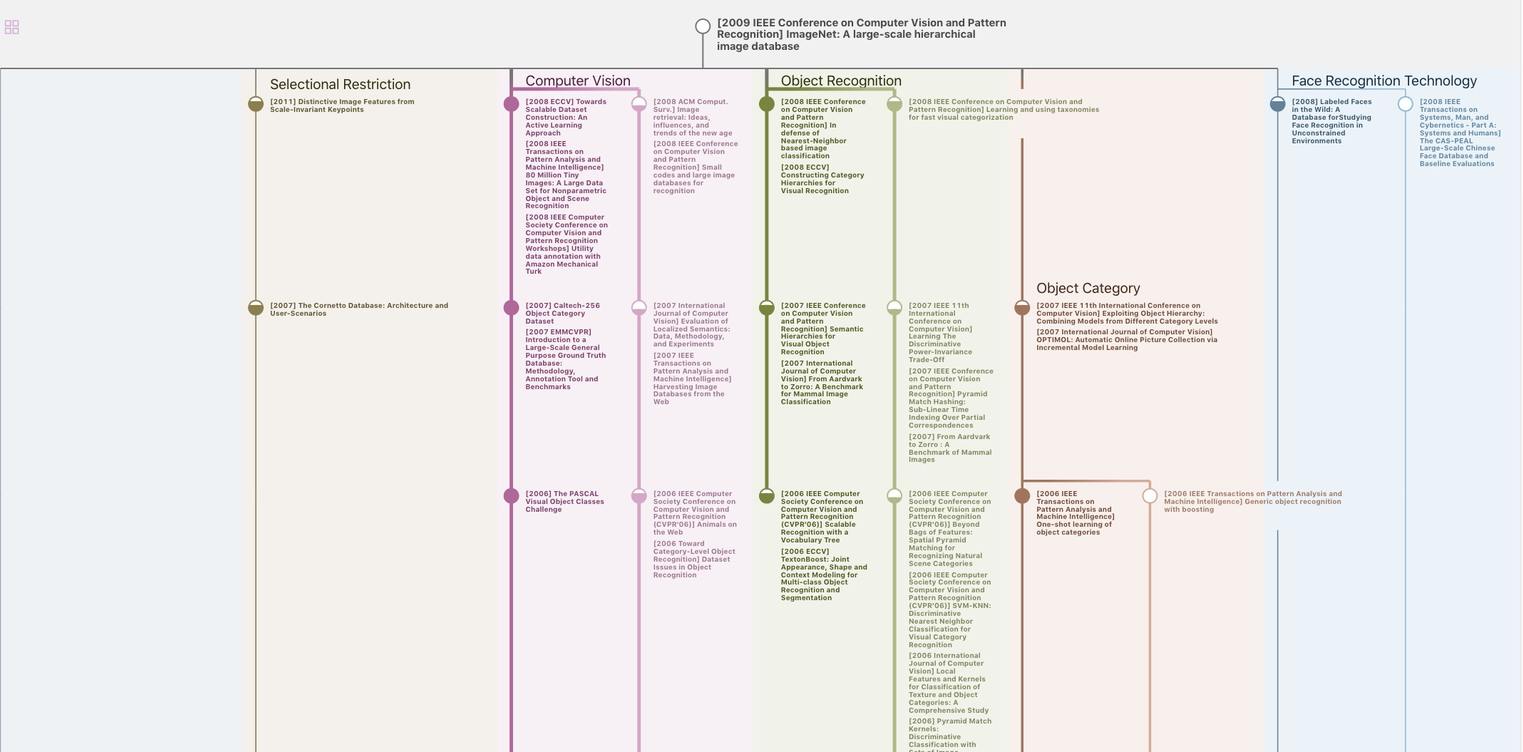
Generate MRT to find the research sequence of this paper
Chat Paper
Summary is being generated by the instructions you defined