Data Aggregation for Hierarchical Clustering
CoRR(2023)
Abstract
Hierarchical Agglomerative Clustering (HAC) is likely the earliest and most flexible clustering method, because it can be used with many distances, similarities, and various linkage strategies. It is often used when the number of clusters the data set forms is unknown and some sort of hierarchy in the data is plausible. Most algorithms for HAC operate on a full distance matrix, and therefore require quadratic memory. The standard algorithm also has cubic runtime to produce a full hierarchy. Both memory and runtime are especially problematic in the context of embedded or otherwise very resource-constrained systems. In this section, we present how data aggregation with BETULA, a numerically stable version of the well known BIRCH data aggregation algorithm, can be used to make HAC viable on systems with constrained resources with only small losses on clustering quality, and hence allow exploratory data analysis of very large data sets.
MoreTranslated text
AI Read Science
Must-Reading Tree
Example
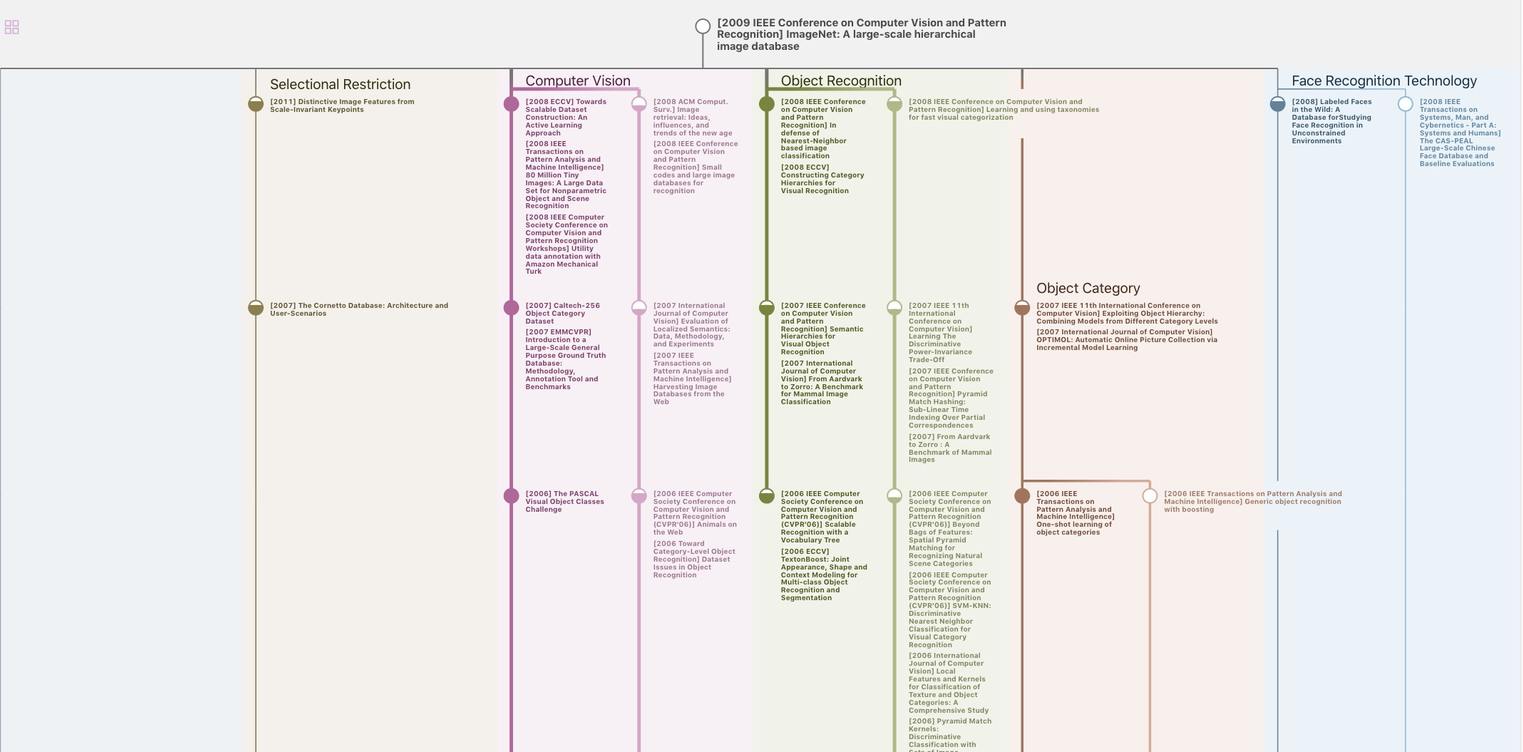
Generate MRT to find the research sequence of this paper
Chat Paper
Summary is being generated by the instructions you defined