Learning Observation Models with Incremental Non-Differentiable Graph Optimizers in the Loop for Robotics State Estimation
CoRR(2023)
摘要
We consider the problem of learning observation models for robot state estimation with incremental non-differentiable optimizers in the loop. Convergence to the correct belief over the robot state is heavily dependent on a proper tuning of observation models which serve as input to the optimizer. We propose a gradient-based learning method which converges much quicker to model estimates that lead to solutions of much better quality compared to an existing state-of-the-art method as measured by the tracking accuracy over unseen robot test trajectories.
更多查看译文
关键词
observation models,optimizers,learning,non-differentiable
AI 理解论文
溯源树
样例
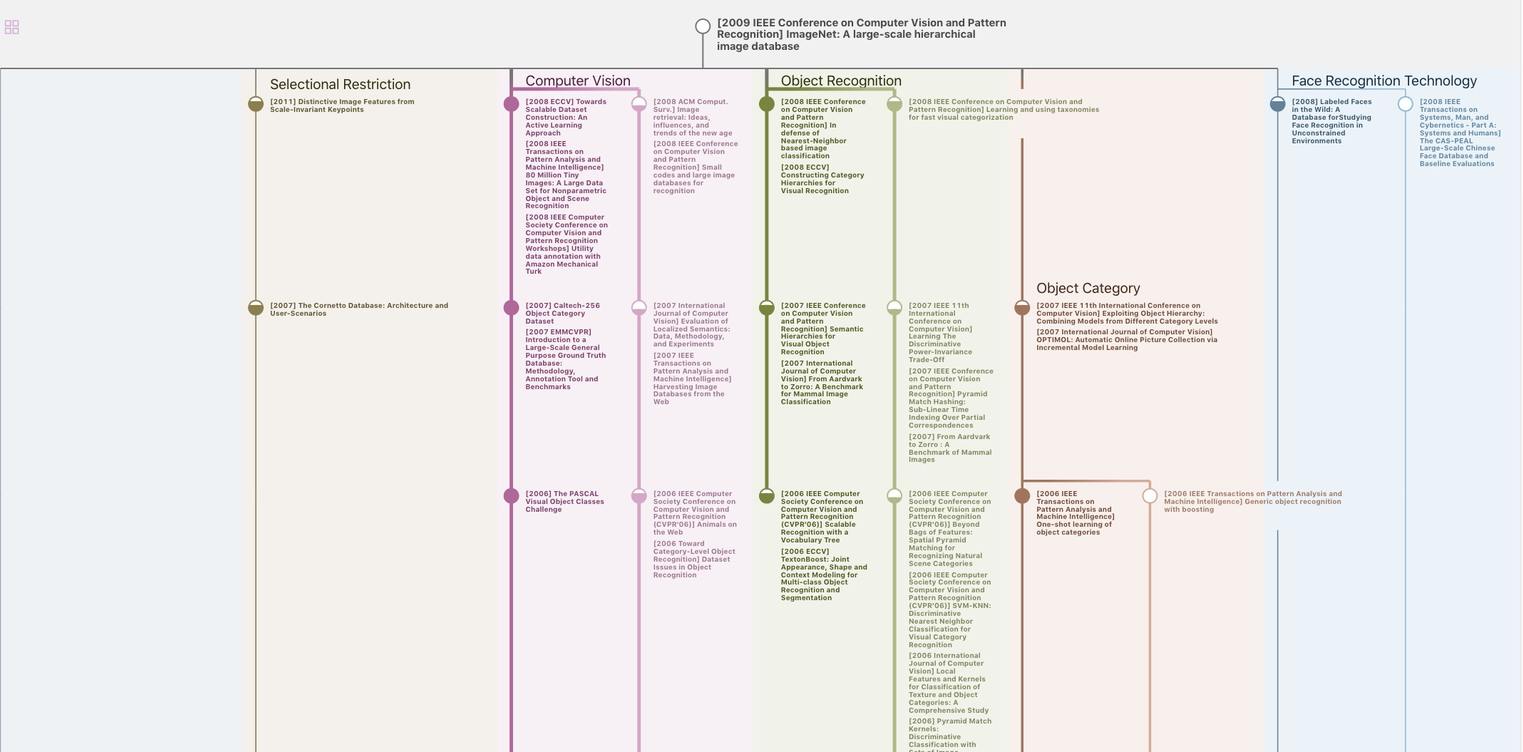
生成溯源树,研究论文发展脉络
Chat Paper
正在生成论文摘要