Towards Frugal Unsupervised Detection of Subtle Abnormalities in Medical Imaging
MEDICAL IMAGE COMPUTING AND COMPUTER ASSISTED INTERVENTION, MICCAI 2023, PT III(2023)
摘要
Anomaly detection in medical imaging is a challenging task in contexts where abnormalities are not annotated. This problem can be addressed through unsupervised anomaly detection (UAD) methods, which identify features that do not match with a reference model of normal profiles. Artificial neural networks have been extensively used for UAD but they do not generally achieve an optimal trade-off between accuracy and computational demand. As an alternative, we investigate mixtures of probability distributions whose versatility has been widely recognized for a variety of data and tasks, while not requiring excessive design effort or tuning. Their expressivity makes them good candidates to account for complex multivariate reference models. Their much smaller number of parameters makes them more amenable to interpretation and efficient learning. However, standard estimation procedures, such as the Expectation-Maximization algorithm, do not scale well to large data volumes as they require high memory usage. To address this issue, we propose to incrementally compute inferential quantities. This online approach is illustrated on the challenging detection of subtle abnormalities in MR brain scans for the follow-up of newly diagnosed Parkinsonian patients. The identified structural abnormalities are consistent with the disease progression, as accounted by the Hoehn and Yahr scale.
更多查看译文
关键词
Frugal computing,Online EM algorithm,Gaussian scale mixture,Unsupervised anomaly detection,Parkinson's Disease
AI 理解论文
溯源树
样例
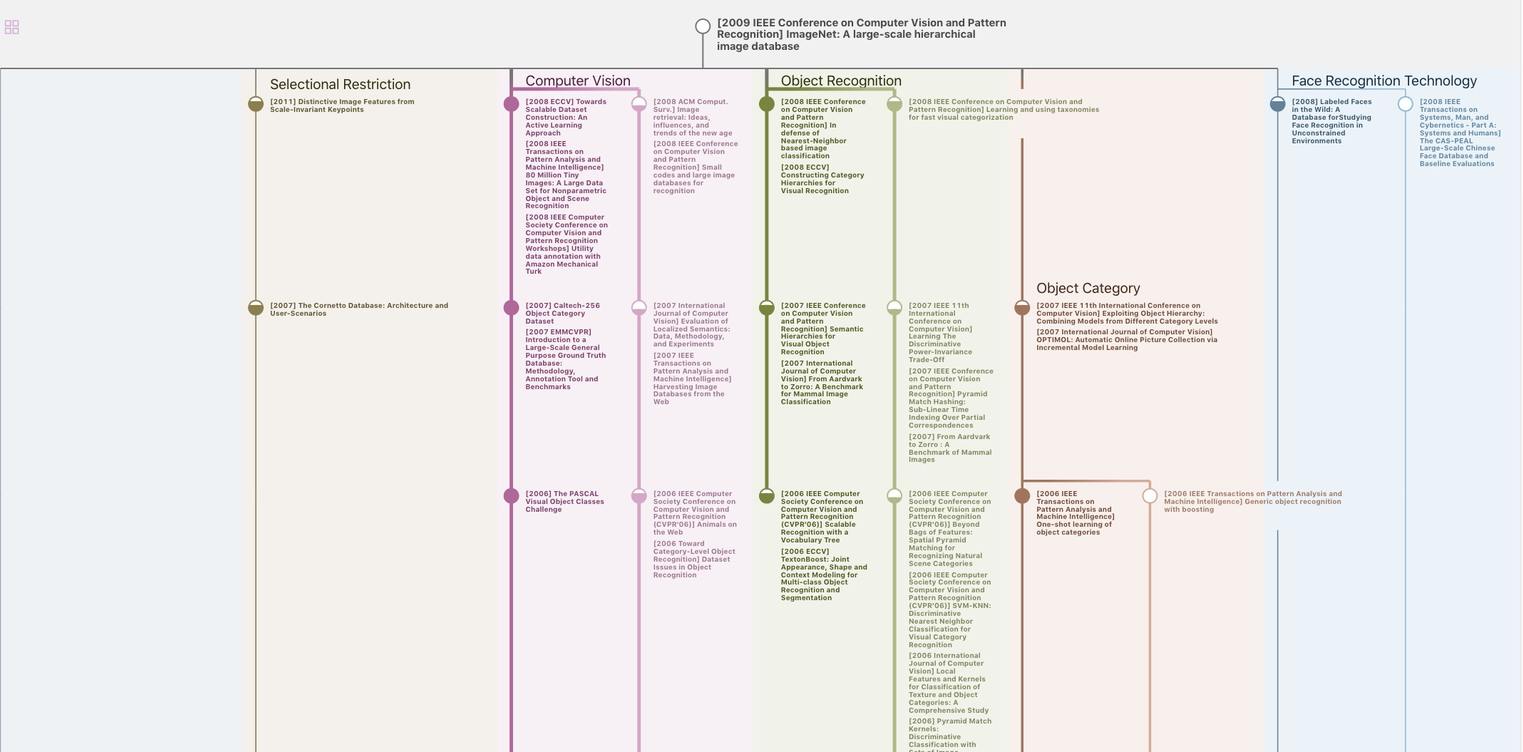
生成溯源树,研究论文发展脉络
Chat Paper
正在生成论文摘要