Adversarial Learning Aided Malicious Network Traffic Detection Based on EDE Framework
2023 IEEE/CIC International Conference on Communications in China (ICCC)(2023)
摘要
With the increasing number of new malicious software and network attacks, anomalous network traffic detection technology needs to continuously identify unknown attacks in order to adapt to complex network environments. In this paper, we propose a novel adversarial learning aided malicious network traffic detection (AMD) method based on encoder-decoder-encoder (EDE) framework. The proposed AMD method detects unknown anomalies in a single type of semi-supervised form and converts network traffic data into pictures for later processing. First, an anomalous network traffic detection model consisting of two encoders, a decoder and a discriminator is introduced. Second, by using the visibility of normal samples and the unrecognizability of unknown anomaly samples, the difference between the two samples is enlarged to facilitate effective sample detection. Finally, experiments show that the proposed model detects anomalies in targeted space and is robust to complex and noisy network environments. At the same time, compared to traditional machine learning methods, AMD’s shows performance improvement of up to 40%.
更多查看译文
关键词
Anomaly detection,adversarial learning,malicious network traffic,semi-supervised learning
AI 理解论文
溯源树
样例
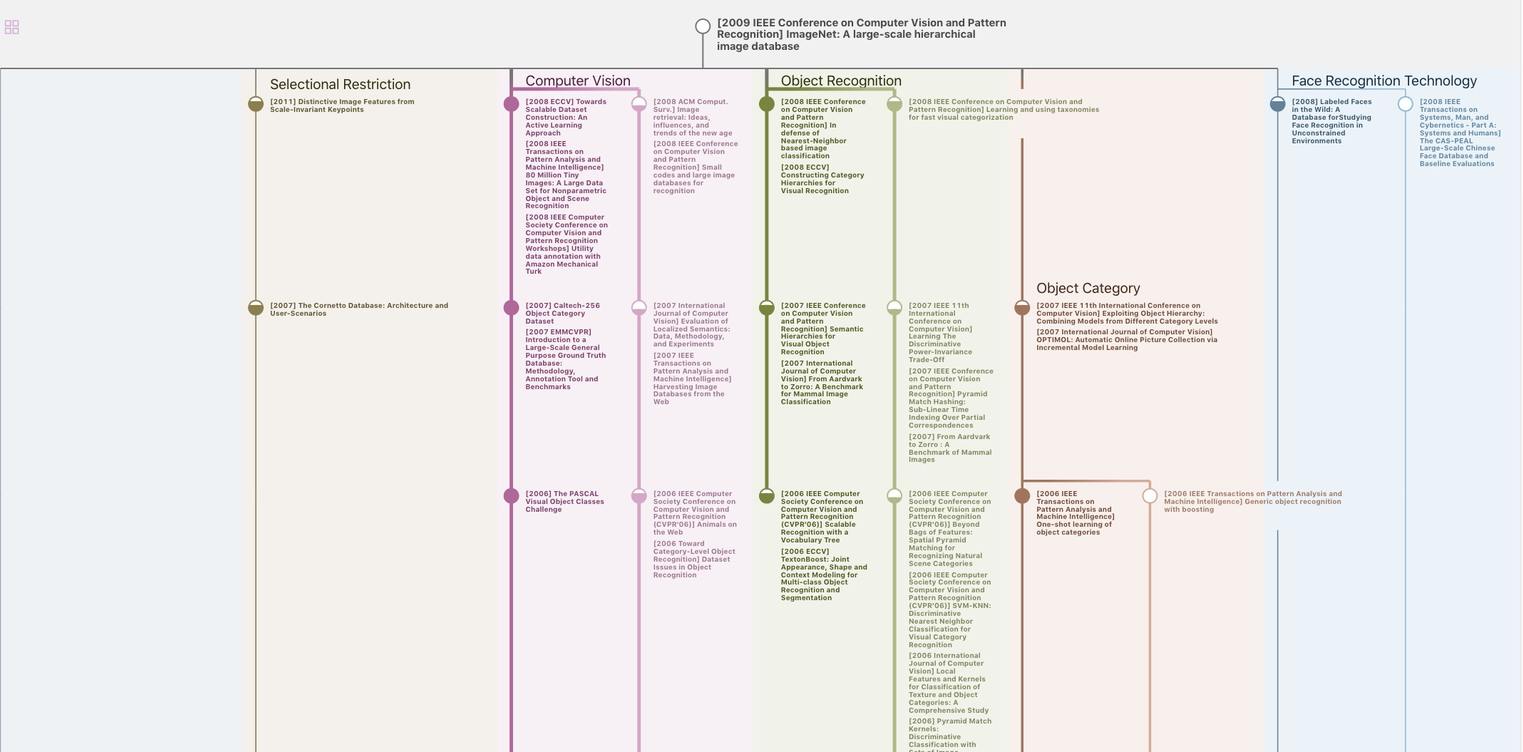
生成溯源树,研究论文发展脉络
Chat Paper
正在生成论文摘要