Robust Least Squares Projection Twin SVM and its Sparse Solution
Journal of Systems Engineering and Electronics(2023)
摘要
Least squares projection twin support vector machine (LSPTSVM) has faster computing speed than classical least squares support vector machine (LSSVM). However, LSPTSVM is sensitive to outliers and its solution lacks sparsity. Therefore, it is difficult for LSPTSVM to process large-scale datasets with outliers. In this paper, we propose a robust LSPTSVM model (called R-LSPTSVM) by applying truncated least squares loss function. The robustness of R-LSPTSVM is proved from a weighted perspective. Furthermore, we obtain the sparse solution of R-LSPTSVM by using the pivoting Cholesky factorization method in primal space. Finally, the sparse R-LSPTSVM algorithm (SR-LSPTSVM) is proposed. Experimental results show that SR-LSPTSVM is insensitive to outliers and can deal with large-scale datasets fastly.
更多查看译文
关键词
twin
AI 理解论文
溯源树
样例
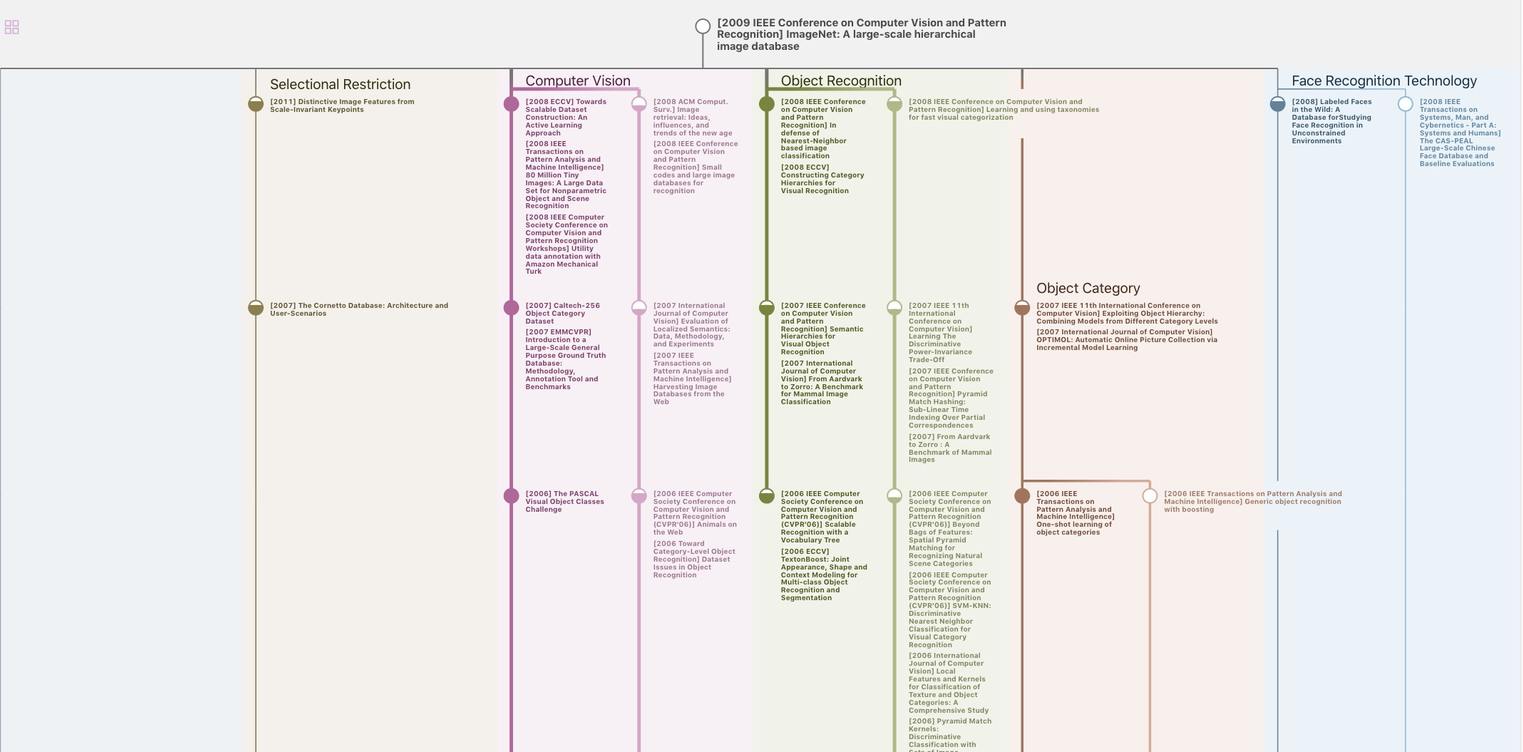
生成溯源树,研究论文发展脉络
Chat Paper
正在生成论文摘要