A Likelihood Approach to Incorporating Self-Report Data in HIV Recency Classification
arXiv (Cornell University)(2023)
摘要
Estimating new HIV infections is significant yet challenging due to the difficulty in distinguishing between recent and long-term infections. We demonstrate that HIV recency status (recent v.s. long-term) could be determined from the combination of self-report testing history and biomarkers, which are increasingly available in bio-behavioral surveys. HIV recency status is partially observed, given the self-report testing history. For example, people who tested positive for HIV over one year ago should have a long-term infection. Based on the nationally representative samples collected by the Population-based HIV Impact Assessment (PHIA) Project, we propose a likelihood-based probabilistic model for HIV recency classification. The model incorporates both labeled and unlabeled data and integrates the mechanism of how HIV recency status depends on biomarkers and the mechanism of how HIV recency status, together with the self-report time of the most recent HIV test, impacts the test results, via a set of logistic regression models. We compare our method to logistic regression and the binary classification tree (current practice) on Malawi, Zimbabwe, and Zambia PHIA data, as well as on simulated data. Our model obtains more efficient and less biased parameter estimates and is relatively robust to potential reporting error and model misspecification.
更多查看译文
关键词
hiv recency
AI 理解论文
溯源树
样例
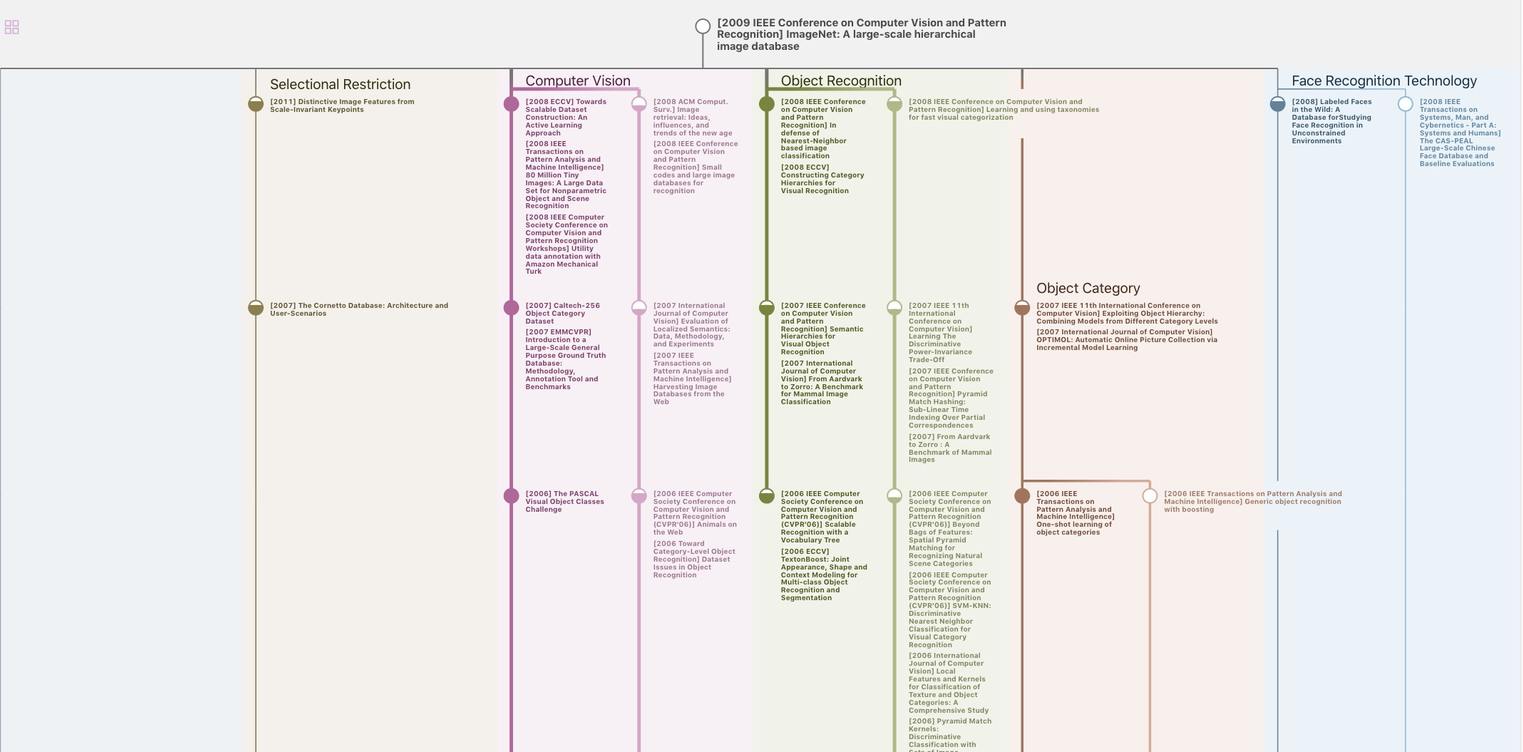
生成溯源树,研究论文发展脉络
Chat Paper
正在生成论文摘要