Optimization tools for Twin-in-the-Loop vehicle control design: analysis and yaw-rate tracking case study
arxiv(2023)
摘要
Given the urgent need of simplifying the end-of-line tuning of complex
vehicle dynamics controllers, the Twin-in-the-Loop Control (TiL-C) approach was
recently proposed in the automotive field. In TiL-C, a digital twin is run
on-board to compute a nominal control action in run-time and an additional
block C_delta is used to compensate for the mismatch between the simulator and
the real vehicle. As the digital twin is assumed to be the best replica
available of the real plant, the key issue in TiL-C becomes the tuning of the
compensator, which must be performed relying on data only. In this paper, we
investigate the use of different black-box optimization techniques for the
calibration of C_delta. More specifically, we compare the originally proposed
Bayesian Optimization (BO) approach with the recently developed Set Membership
Global Optimization (SMGO) and Virtual Reference Feedback Tuning (VRFT), a
one-shot direct data-driven design method. The analysis will be carried out
within a professional multibody simulation environment on a novel TiL-C
application case study – the yaw-rate tracking problem – so as to further
prove the TiL-C effctiveness on a challenging problem. Simulations will show
that the VRFT approach is capable of providing a well tuned controller after a
single iteration, while 10 to 15 iterations are necessary for refining it with
global optimizers. Also, SMGO is shown to significantly reduce the
computational effort required by BO.
更多查看译文
AI 理解论文
溯源树
样例
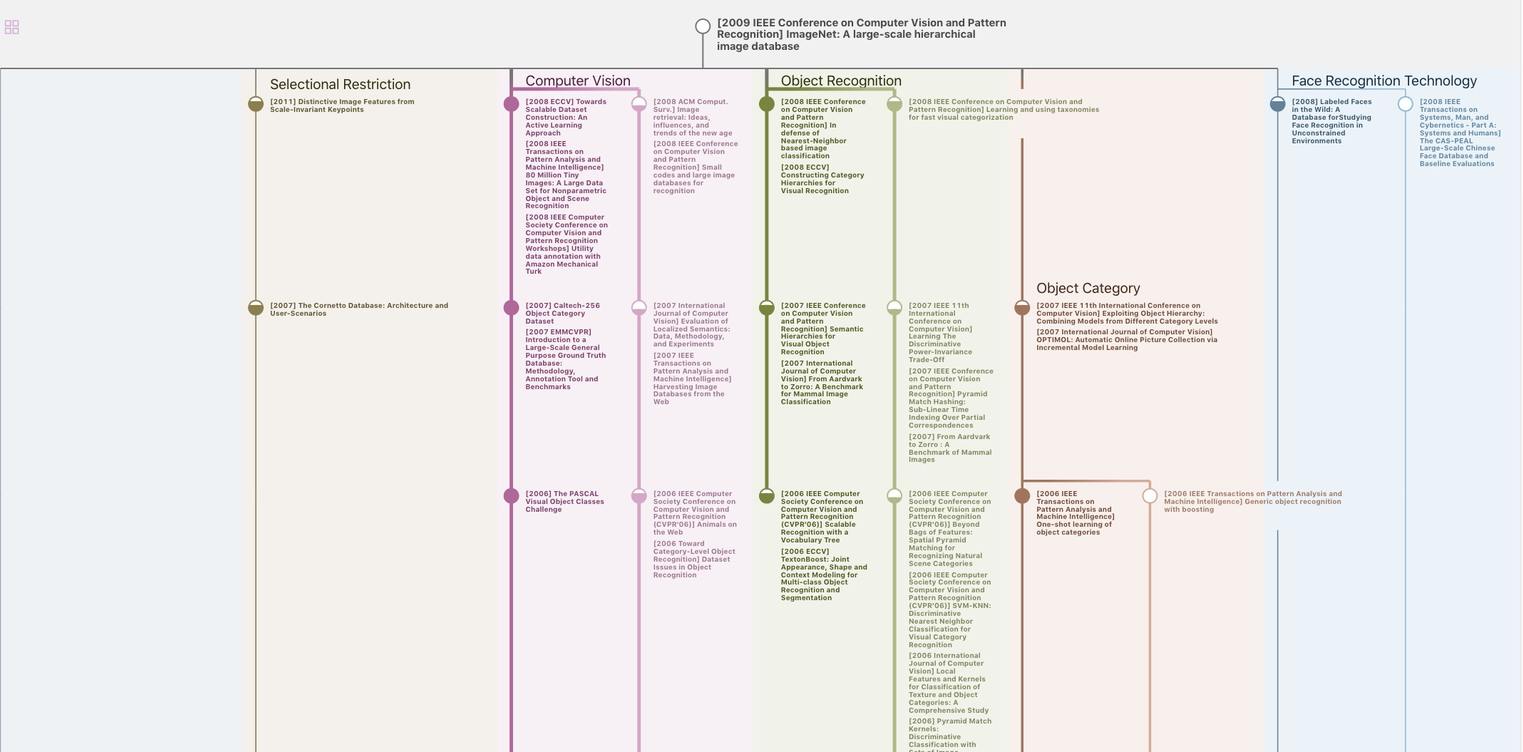
生成溯源树,研究论文发展脉络
Chat Paper
正在生成论文摘要