CONFIDERAI: a novel CONFormal Interpretable-by-Design score function for Explainable and Reliable Artificial Intelligence
arXiv (Cornell University)(2023)
Abstract
Everyday life is increasingly influenced by artificial intelligence, and
there is no question that machine learning algorithms must be designed to be
reliable and trustworthy for everyone. Specifically, computer scientists
consider an artificial intelligence system safe and trustworthy if it fulfills
five pillars: explainability, robustness, transparency, fairness, and privacy.
In addition to these five, we propose a sixth fundamental aspect: conformity,
that is, the probabilistic assurance that the system will behave as the machine
learner expects. In this paper, we present a methodology to link conformal
prediction with explainable machine learning by defining a new score function
for rule-based classifiers that leverages rules predictive ability, the
geometrical position of points within rules boundaries and the overlaps among
rules as well, thanks to the definition of a geometrical rule similarity term.
Furthermore, we address the problem of defining regions in the feature space
where conformal guarantees are satisfied, by exploiting the definition of
conformal critical set and showing how this set can be used to achieve new
rules with improved performance on the target class. The overall methodology is
tested with promising results on several datasets of real-world interest, such
as domain name server tunneling detection or cardiovascular disease prediction.
MoreTranslated text
Key words
reliable artificial intelligence,artificial intelligence,confiderai,interpretable-by-design
AI Read Science
Must-Reading Tree
Example
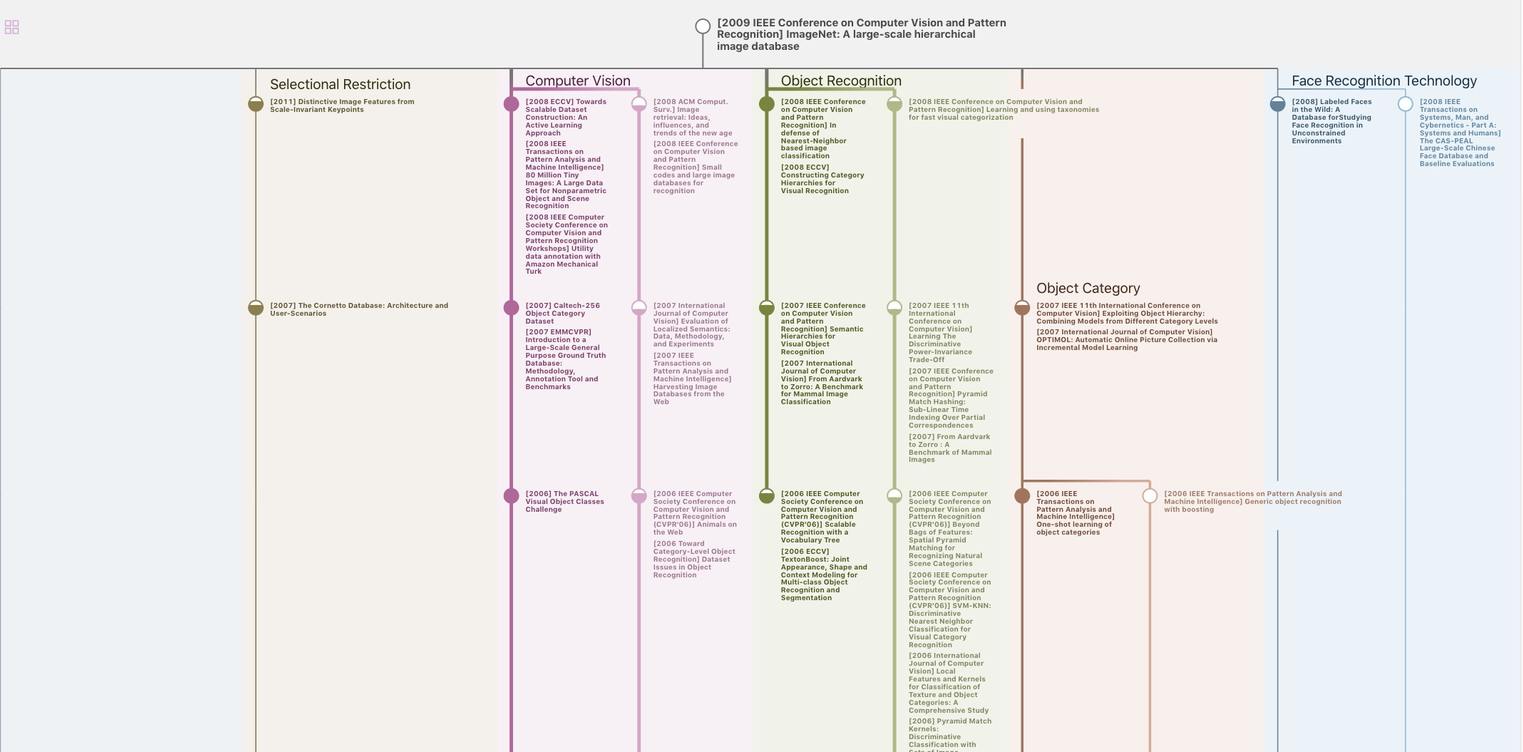
Generate MRT to find the research sequence of this paper
Chat Paper
Summary is being generated by the instructions you defined