Blind Biological Sequence Denoising with Self-Supervised Set Learning
CoRR(2023)
Abstract
Biological sequence analysis relies on the ability to denoise the imprecise output of sequencing platforms. We consider a common setting where a short sequence is read out repeatedly using a high-throughput long-read platform to generate multiple subreads, or noisy observations of the same sequence. Denoising these subreads with alignment-based approaches often fails when too few subreads are available or error rates are too high. In this paper, we propose a novel method for blindly denoising sets of sequences without directly observing clean source sequence labels. Our method, Self-Supervised Set Learning (SSSL), gathers subreads together in an embedding space and estimates a single set embedding as the midpoint of the subreads in both the latent and sequence spaces. This set embedding represents the "average" of the subreads and can be decoded into a prediction of the clean sequence. In experiments on simulated long-read DNA data, SSSL methods denoise small reads of $\leq 6$ subreads with 17% fewer errors and large reads of $>6$ subreads with 8% fewer errors compared to the best baseline. On a real dataset of antibody sequences, SSSL improves over baselines on two self-supervised metrics, with a significant improvement on difficult small reads that comprise over 60% of the test set. By accurately denoising these reads, SSSL promises to better realize the potential of high-throughput DNA sequencing data for downstream scientific applications.
MoreTranslated text
Key words
learning,set,self-supervised
AI Read Science
Must-Reading Tree
Example
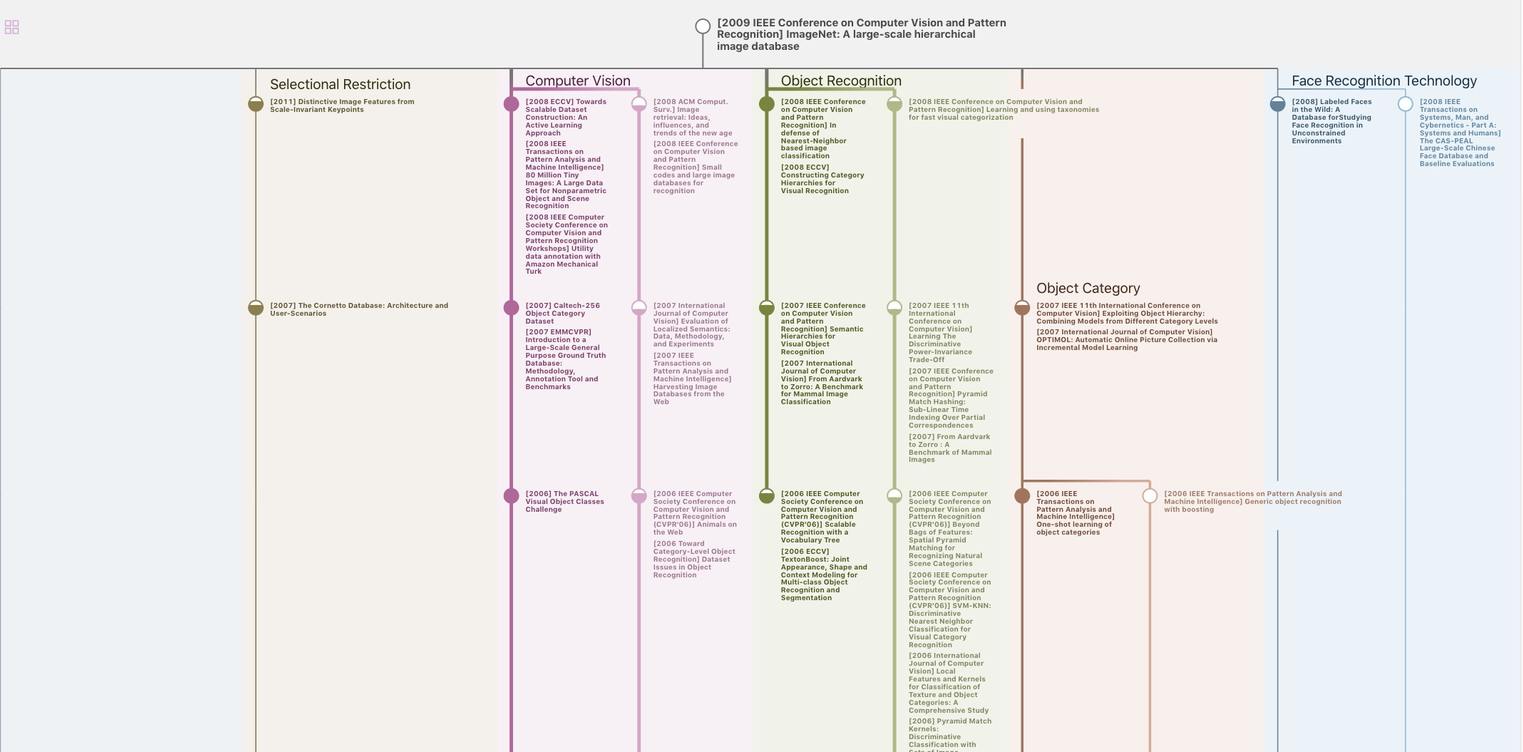
Generate MRT to find the research sequence of this paper
Chat Paper
Summary is being generated by the instructions you defined