Improving Visual Quality and Transferability of Adversarial Attacks on Face Recognition Simultaneously with Adversarial Restoration
ICASSP 2024 - 2024 IEEE International Conference on Acoustics, Speech and Signal Processing (ICASSP)(2023)
摘要
Adversarial face examples possess two critical properties: Visual Quality and
Transferability. However, existing approaches rarely address these properties
simultaneously, leading to subpar results. To address this issue, we propose a
novel adversarial attack technique known as Adversarial Restoration
(AdvRestore), which enhances both visual quality and transferability of
adversarial face examples by leveraging a face restoration prior. In our
approach, we initially train a Restoration Latent Diffusion Model (RLDM)
designed for face restoration. Subsequently, we employ the inference process of
RLDM to generate adversarial face examples. The adversarial perturbations are
applied to the intermediate features of RLDM. Additionally, by treating RLDM
face restoration as a sibling task, the transferability of the generated
adversarial face examples is further improved. Our experimental results
validate the effectiveness of the proposed attack method.
更多查看译文
AI 理解论文
溯源树
样例
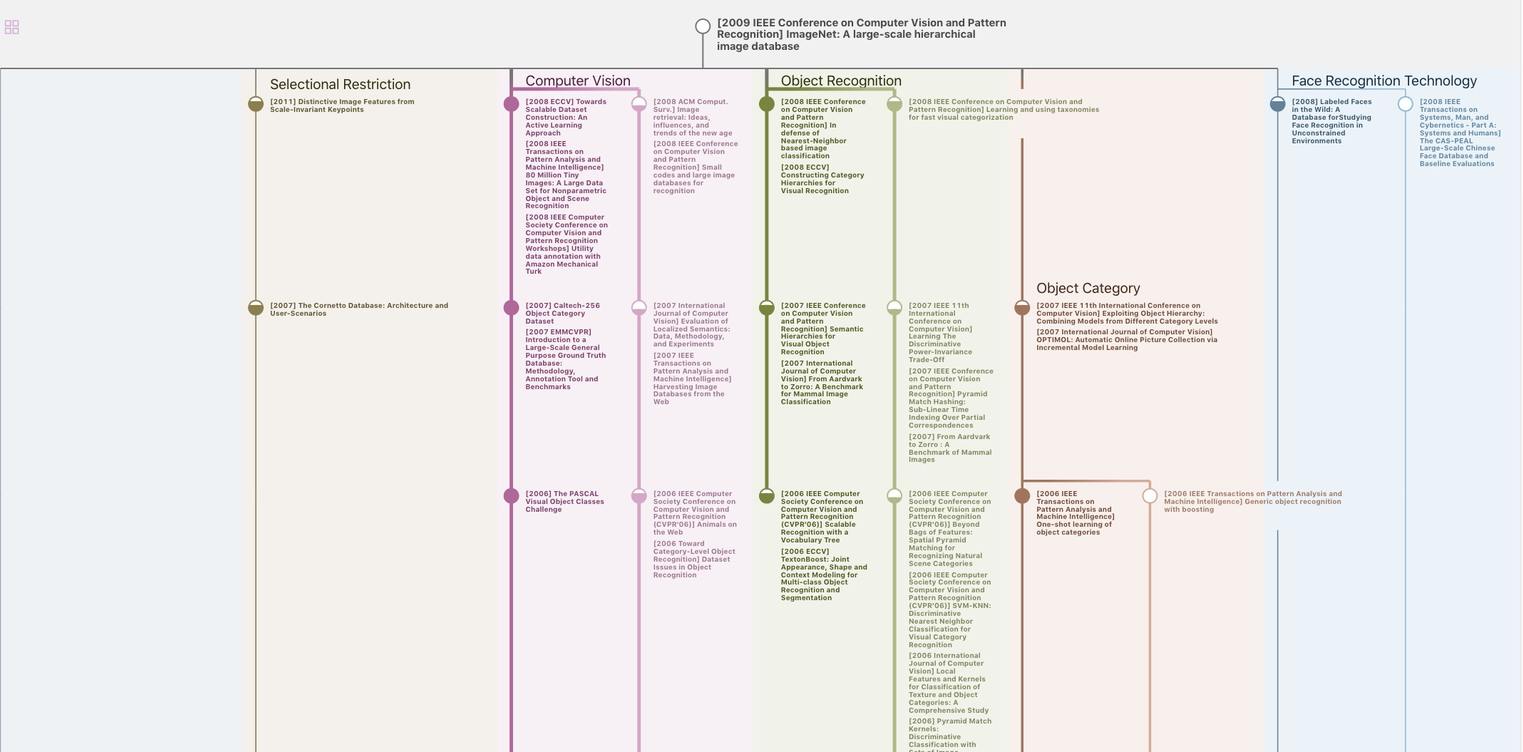
生成溯源树,研究论文发展脉络
Chat Paper
正在生成论文摘要