Evaluation of forecasts by a global data-driven weather model with and without probabilistic post-processing at Norwegian stations
arXiv (Cornell University)(2023)
摘要
During the last two years, tremendous progress in global data-driven weather models trained on numerical weather prediction (NWP) re-analysis data has been made. The most recent models trained on the ERA5 at 0.25{\deg} resolution demonstrate forecast quality on par with ECMWF's high-resolution model with respect to a wide selection of verification metrics. In this study, one of these models, the Pangu-Weather, is compared to several NWP models with and without probabilistic post-processing for 2-meter temperature and 10-meter wind speed forecasting at 183 Norwegian SYNOP stations up to +60 hours ahead. The NWP models included are the ECMWF HRES, ECMWF ENS and the Harmonie-AROME ensemble model MEPS with 2.5 km spatial resolution. Results show that the performances of the global models are on the same level with Pangu-Weather being slightly better than the ECMWF models for temperature and slightly worse for wind speed. The MEPS model clearly provided the best forecasts for both parameters. The post-processing improved the forecast quality considerably for all models, but to a larger extent for the coarse-resolution global models due to stronger systematic deficiencies in these. Apart from this, the main characteristics in the scores were more or less the same with and without post-processing. Our results thus confirm the conclusions from other studies that global data-driven models are promising for operational weather forecasting.
更多查看译文
关键词
weather model,forecasts,data-driven,post-processing
AI 理解论文
溯源树
样例
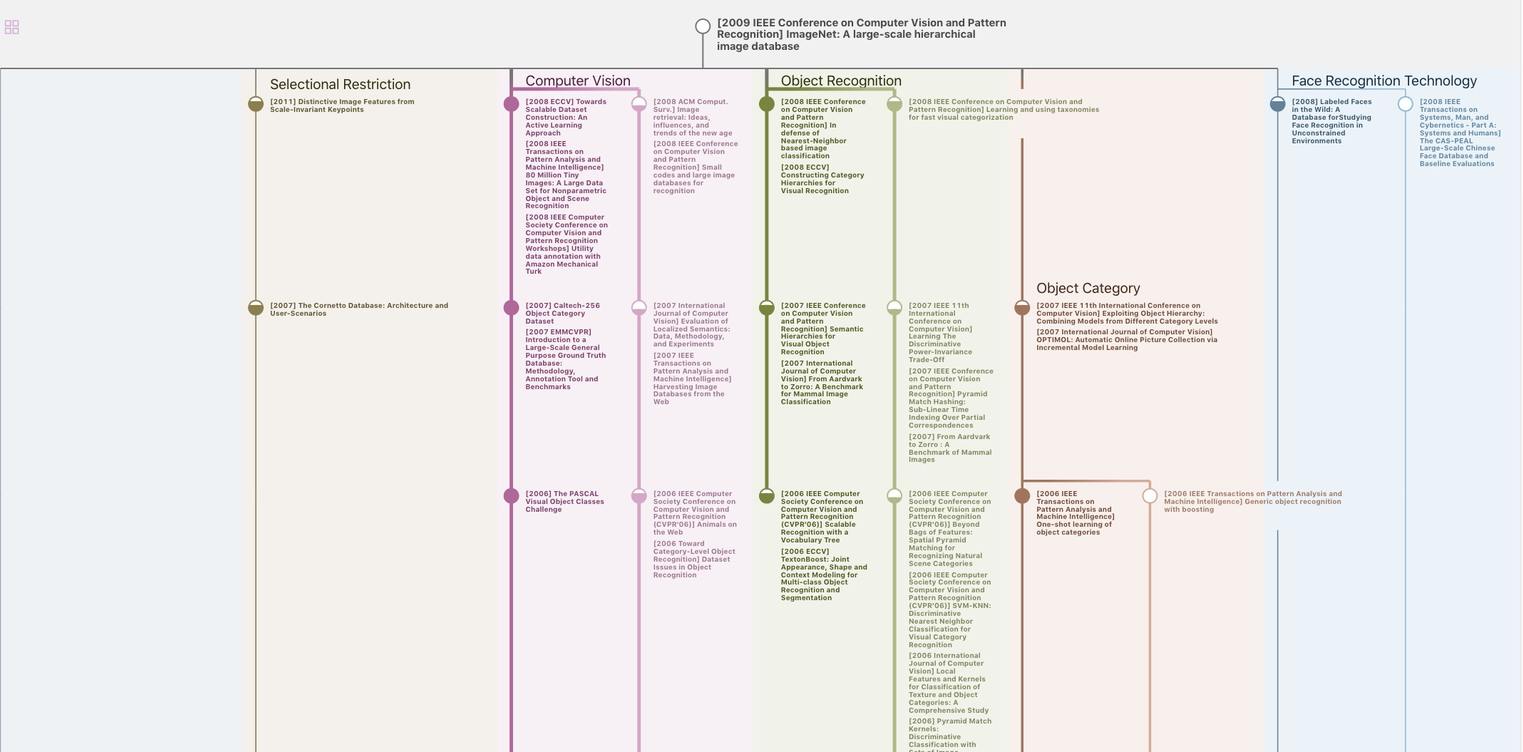
生成溯源树,研究论文发展脉络
Chat Paper
正在生成论文摘要