SEPAL: Spatial Gene Expression Prediction from Local Graphs
2023 IEEE/CVF INTERNATIONAL CONFERENCE ON COMPUTER VISION WORKSHOPS, ICCVW(2023)
摘要
Spatial transcriptomics is an emerging technology that aligns histopathology images with spatially resolved gene expression profiling. It holds the potential for understanding many diseases but faces significant bottlenecks such as specialized equipment and domain expertise. In this work, we present SEPAL, a new model for predicting genetic profiles from visual tissue appearance. Our method exploits the biological biases of the problem by directly supervising relative differences with respect to mean expression, and leverages local visual context at every coordinate to make predictions using a graph neural network. This approach closes the gap between complete locality and complete globality in current methods. In addition, we propose a novel benchmark that aims to better define the task by following current best practices in transcriptomics and restricting the prediction variables to only those with clear spatial patterns. Our extensive evaluation in two different human breast cancer datasets indicates that SEPAL outperforms previous state-of-the-art methods and other mechanisms of including spatial context.
更多查看译文
关键词
spatial gene expression prediction,local graphs,gene expression
AI 理解论文
溯源树
样例
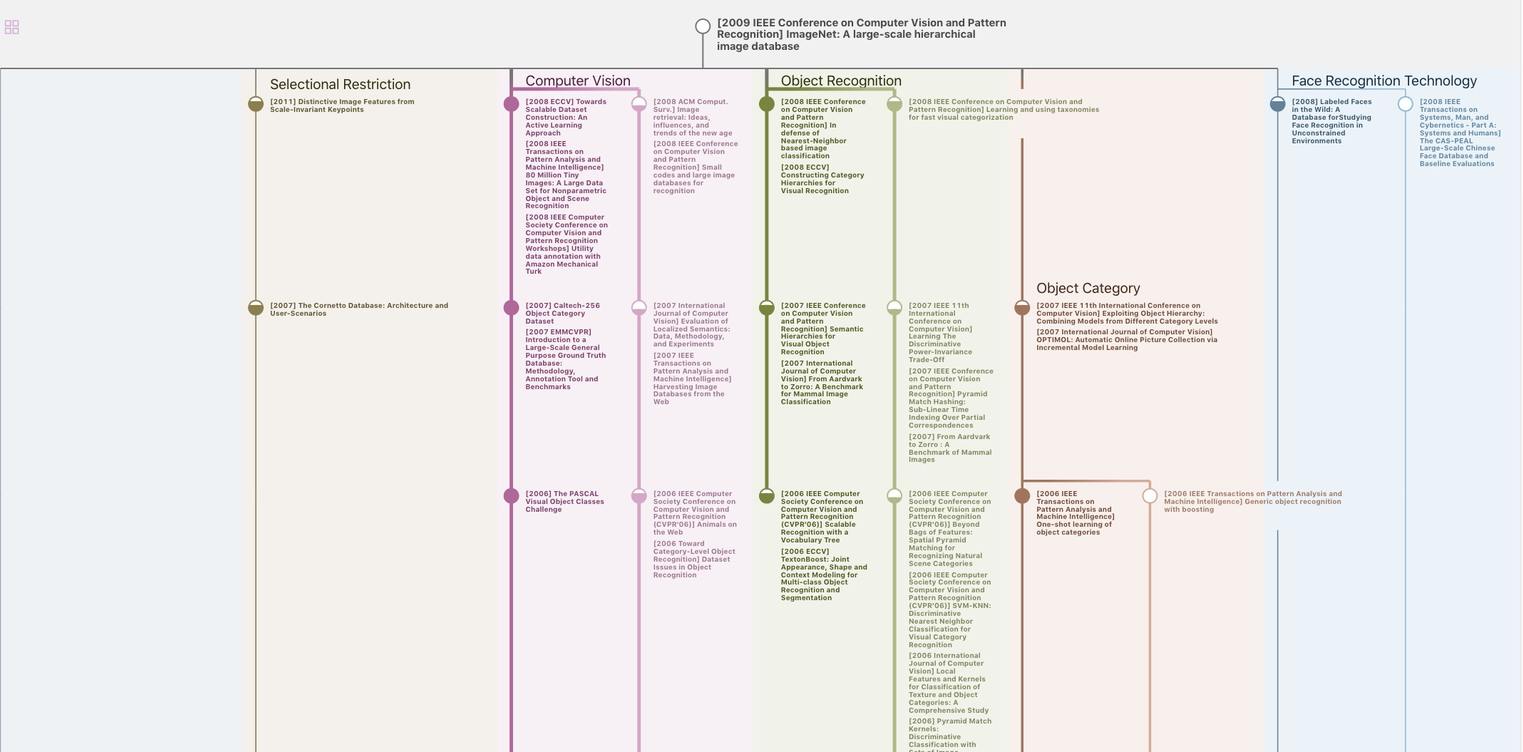
生成溯源树,研究论文发展脉络
Chat Paper
正在生成论文摘要