Domain Generalization via Balancing Training Difficulty and Model Capability
2023 IEEE/CVF International Conference on Computer Vision (ICCV)(2023)
摘要
Domain generalization (DG) aims to learn domain-generalizable models from one or multiple source domains that can perform well in unseen target domains. Despite its recent progress, most existing work suffers from the misalignment between the difficulty level of training samples and the capability of contemporarily trained models, leading to over-fitting or under-fitting in the trained generalization model. We design MoDify, a Momentum Difficulty framework that tackles the misalignment by balancing the seesaw between the model's capability and the samples' difficulties along the training process. MoDify consists of two novel designs that collaborate to fight against the misalignment while learning domain-generalizable models. The first is MoDify-based Data Augmentation which exploits an RGB Shuffle technique to generate difficulty-aware training samples on the fly. The second is MoDify-based Network Optimization which dynamically schedules the training samples for balanced and smooth learning with appropriate difficulty. Without bells and whistles, a simple implementation of MoDify achieves superior performance across multiple benchmarks. In addition, MoDify can complement existing methods as a plug-in, and it is generic and can work for different visual recognition tasks.
更多查看译文
关键词
generalization,domain,training difficulty
AI 理解论文
溯源树
样例
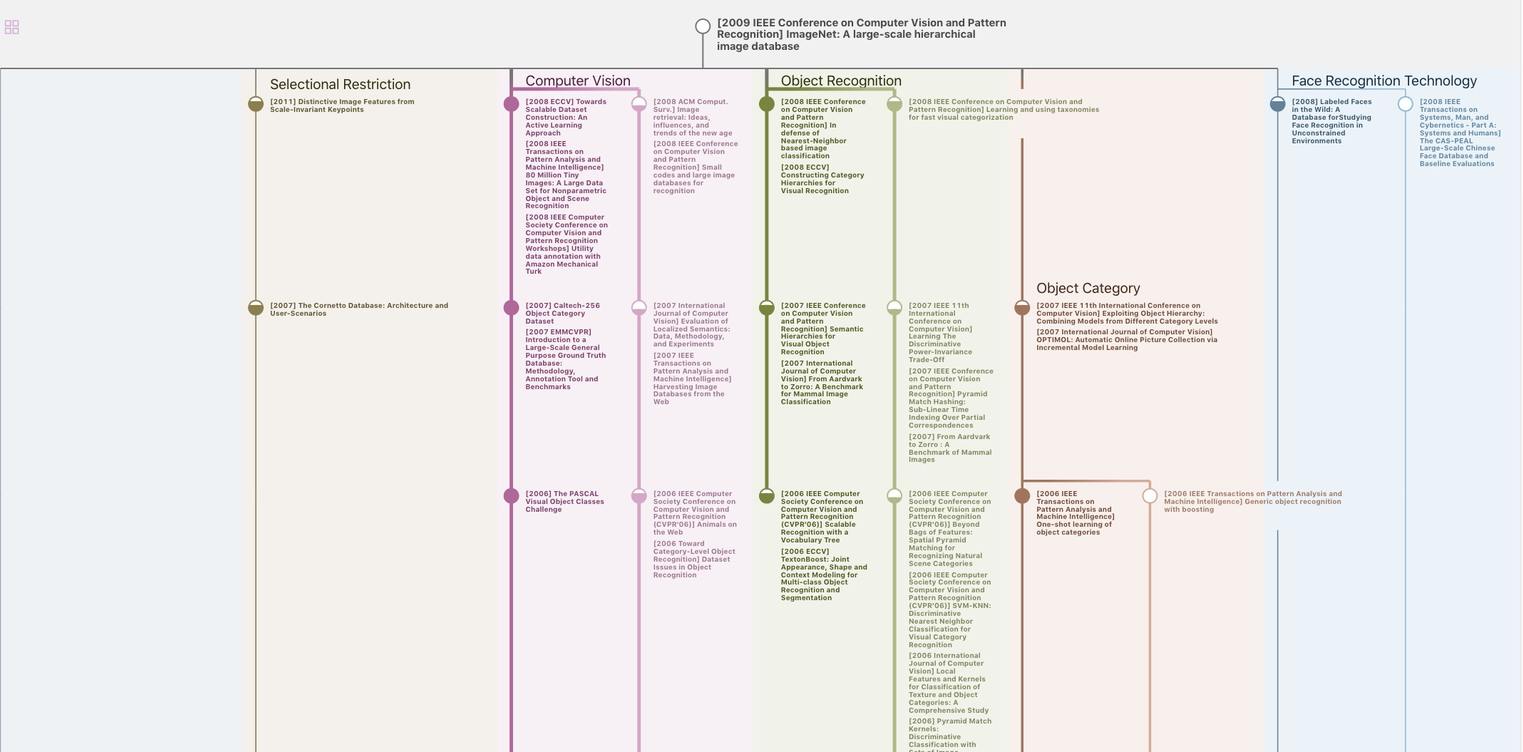
生成溯源树,研究论文发展脉络
Chat Paper
正在生成论文摘要