Using full-scale feature fusion for self-supervised indoor depth estimation
Multimedia Tools and Applications(2024)
摘要
Monocular depth estimation is a crucial task in computer vision, and self-supervised algorithms are gaining popularity due to their independence from expensive ground truth supervision. However, current self-supervised algorithms may not provide accurate estimation and may suffer from distorted boundaries when applied to indoor scenes. Combining multi-scale features is an important research direction in image segmentation to achieve accurate estimation and resolve boundary distortion. However, there are few studies on indoor self-supervised algorithms in this regard. To solve this issue, we propose a novel full-scale feature information fusion approach that includes a full-scale skip-connection and a full-scale feature fusion block. This approach can aggregate the high-level and low-level information of all scale feature maps during the network's encoding and decoding process to compensate for the network's loss of cross-layer feature information. The proposed full-scale feature fusion improves accuracy and reduces the decoder parameters. To fully exploit the superiority of the full-scale feature fusion module, we replace the encoder backbone from ResNet with the more advanced ResNeSt. Combining these two methods results in a significant improvement in prediction accuracy. We have extensively evaluated our approach on the indoor benchmark datasets NYU Depth V2 and ScanNet. Our experimental results demonstrate that our method outperforms existing algorithms, particularly on NYU Depth V2, where our precision is raised to 83.8%.
更多查看译文
关键词
Monocular depth estimation,Feature fusion,Self-supervised,Indoor scenes,ResNeSt
AI 理解论文
溯源树
样例
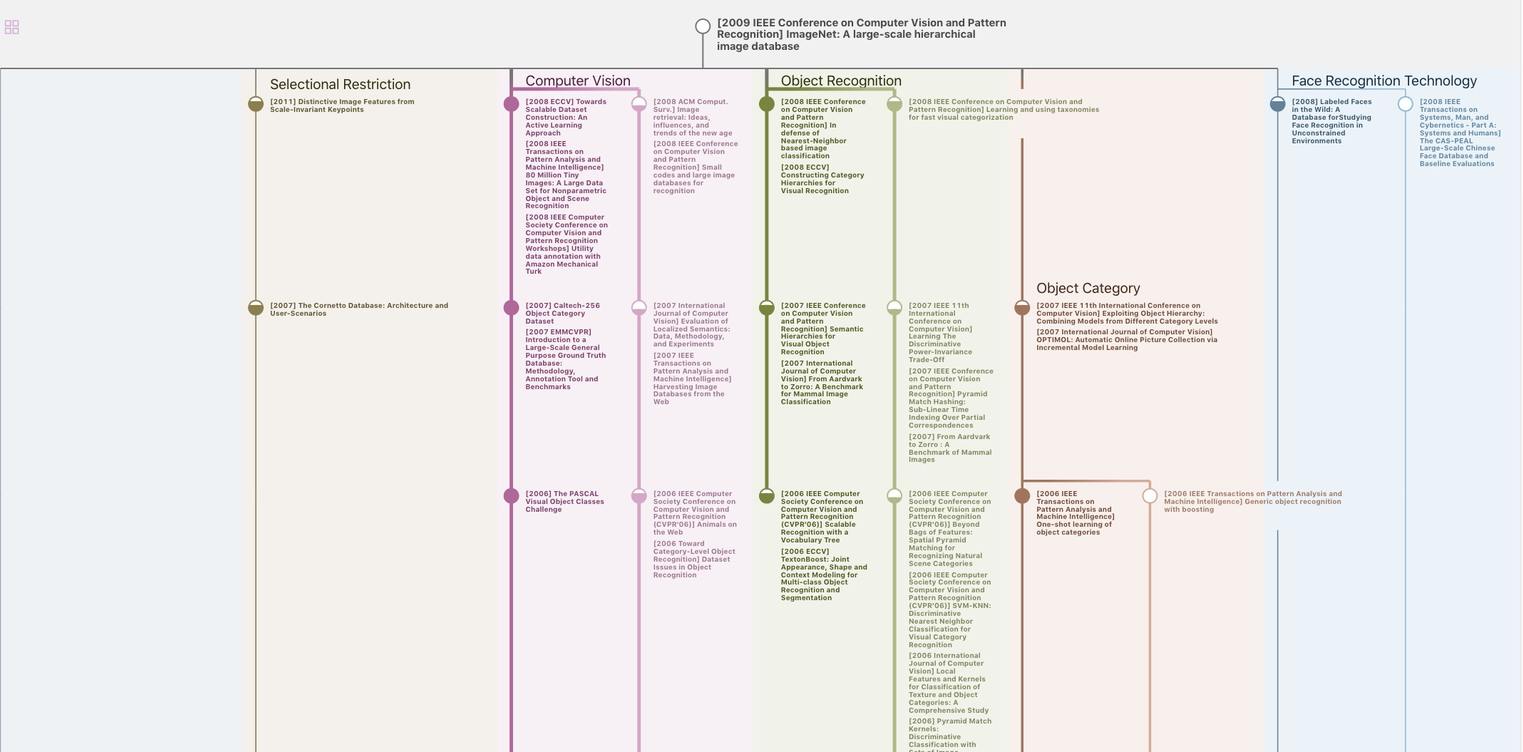
生成溯源树,研究论文发展脉络
Chat Paper
正在生成论文摘要