Bi-objective parameter setting problem of a genetic algorithm: an empirical study on traveling salesperson problem
APPLIED INTELLIGENCE(2023)
摘要
Genetic Algorithm (GA) is a widely used metaheuristic for addressing challenging optimization problems. Selecting suitable settings for GA parameters can lead to a considerable improvement in its performance, but this is often difficult due to the larger number of alternatives available and variable performance depending on the problem solved. Furthermore, consideration of multiple performance criteria in parameter selection adds an additional layer of complexity. Therefore, practitioners often rely on their previous experiences or perform trial-and-error experiments when determining suitable parameter settings. In this study, we define the problem of finding suitable settings for GAs as a bi-objective optimization problem with an aim to find efficient settings that will maximize the approximation quality and minimize the run time of the GA. We conduct an empirical study on a GA that solves Traveling Salesperson Problem (TSP). Two evolutionary algorithms are utilized in a nested manner to address the bi-objective parameter setting problem: a GA to solve the TSP instances and a multi-objective evolutionary algorithm to find the bi-objective efficient settings of the GA. We find efficient settings for each instance through an empirical study with 31 TSP instances and identify settings that perform well regardless of the instance solved. The results provide valuable insights into the behavior of efficient settings, demonstrating that some operators and parameters are robust to changes in problem size, while others require adjustment.
更多查看译文
关键词
Genetic algorithm,Parameter setting problem,Multi-objective optimization,Traveling salesperson problem
AI 理解论文
溯源树
样例
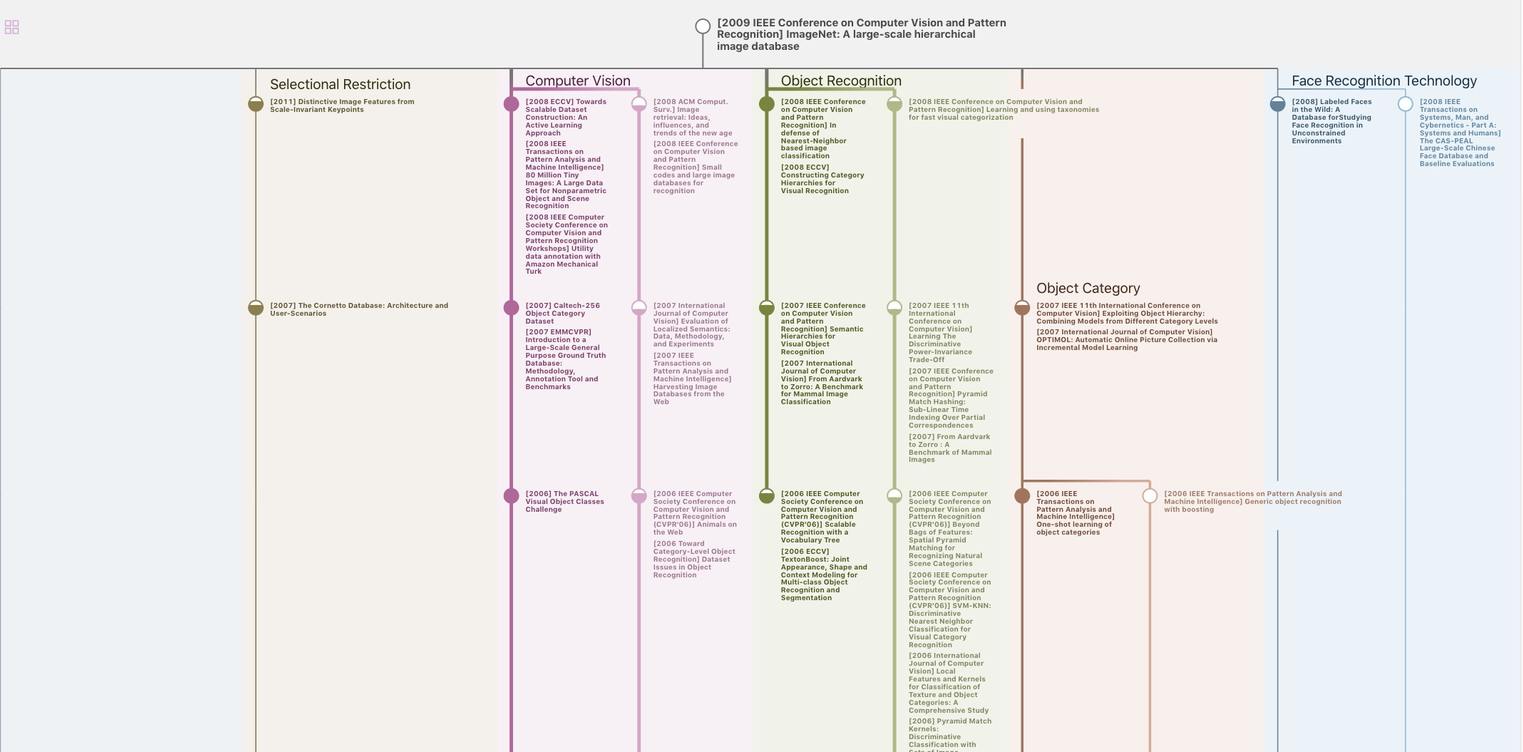
生成溯源树,研究论文发展脉络
Chat Paper
正在生成论文摘要