Single-image super-resolution via a lightweight convolutional neural network with improved shuffle learning
Signal, Image and Video Processing(2024)
Abstract
With the development of deep learning techniques, single-image super-resolution methods based on deep learning have made great progress, enabling significant improvements in image quality and detail reproduction. However, deep convolutional neural networks are often complicated and hard to be understood, and the computational cost limits the application of the models in practical situations. In order to deploy the network on mobile devices with very limited computing power, we build a refined image super-resolution model based on shuffle learning. Based on extensive experimental results on image super-resolution using three widely used datasets, our model not only achieves high scores on the peak signal-to-noise ratio/structural similarity index matrix, but also is simpler and easier to be implemented than other image super-resolution models.
MoreTranslated text
Key words
Super-resolution,Convolutional neural network,Model lightweight,Shuffle units
AI Read Science
Must-Reading Tree
Example
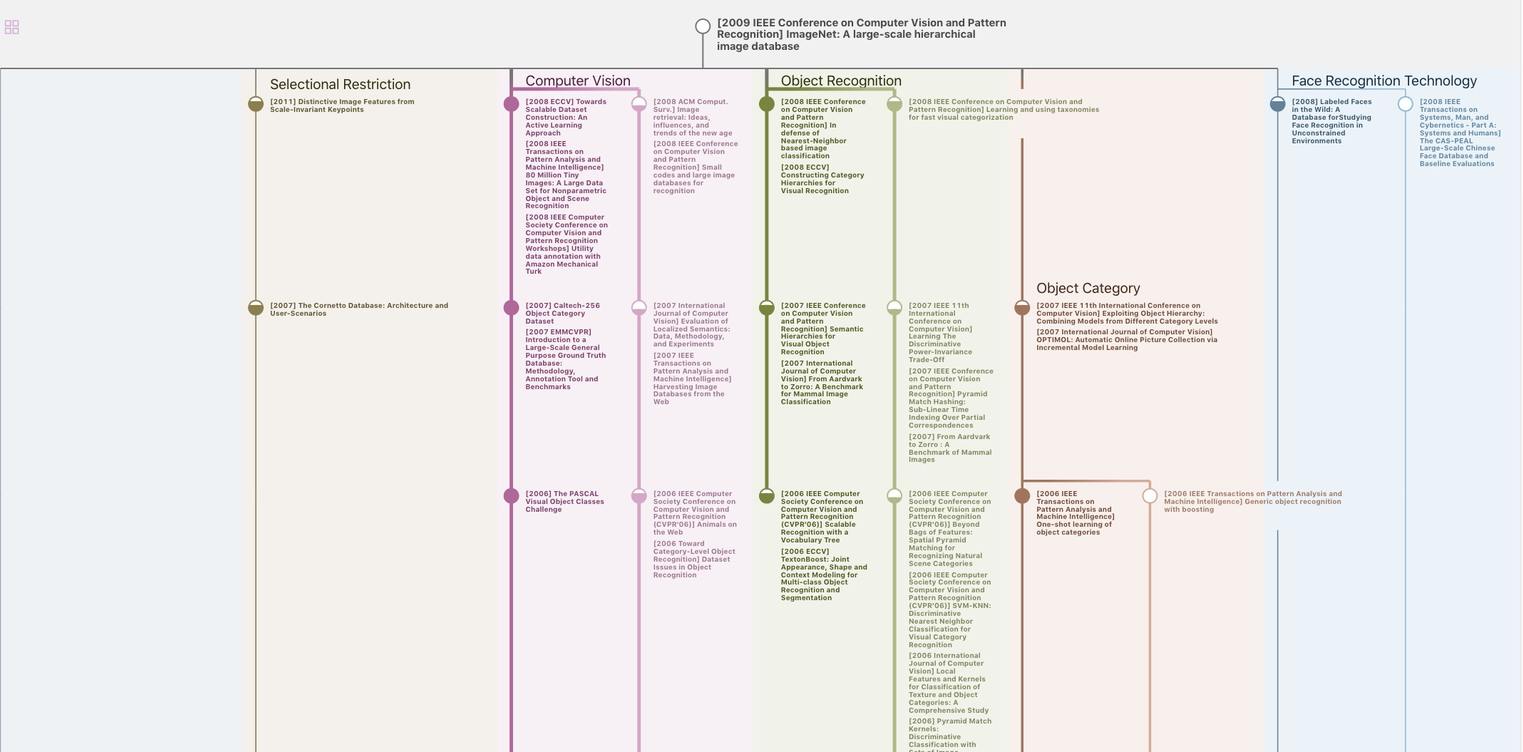
Generate MRT to find the research sequence of this paper
Chat Paper
Summary is being generated by the instructions you defined