CT Synthesis from MRI Using Generative Adversarial Network with Frequency-Aware Discriminator
JOURNAL OF ELECTRICAL ENGINEERING & TECHNOLOGY(2024)
摘要
The pursuit of generating computed tomography (CT) from magnetic resonance imaging (MRI) remains a key area of research with the goal of advancing modern radiation therapy. There has been an increased emphasis on leveraging deep learning methodologies, particularly the generative adversarial network (GAN), to convert MRI into CT. The effectiveness of GAN training hinges on the capacity of its discriminator model to identify and rectify flaws in the synthetic CT, providing valuable feedback to the generator model. Acknowledging the multi-scale complexity of human anatomy, this study introduces an innovative discriminator model, designed to assess the synthetic performance across varying scales and frequencies of tissues and organs. We evaluated the significance of this frequency-aware discriminator by contrasting it with two commonly used discriminator models: the convolutional neural network discriminator and PatchGAN. We conducted our testing within three existing GAN frameworks on a dataset of 78 nasopharyngeal carcinoma patients. The experimental outcomes revealed that our model managed to decrease the mean absolute error between synthetic and actual CT by an average of 0.18–1.55 Hounsfield Units within these frameworks. Additionally, it enhanced the visual quality of synthetic CT, offering superior local structures and patterns. These findings suggest that our newly developed discriminator can offer comprehensive guidance to the generator, thereby enhancing CT synthetic performance.
更多查看译文
关键词
CT synthesis,Deep learning,Generative adversarial network,Frequency-aware discriminator
AI 理解论文
溯源树
样例
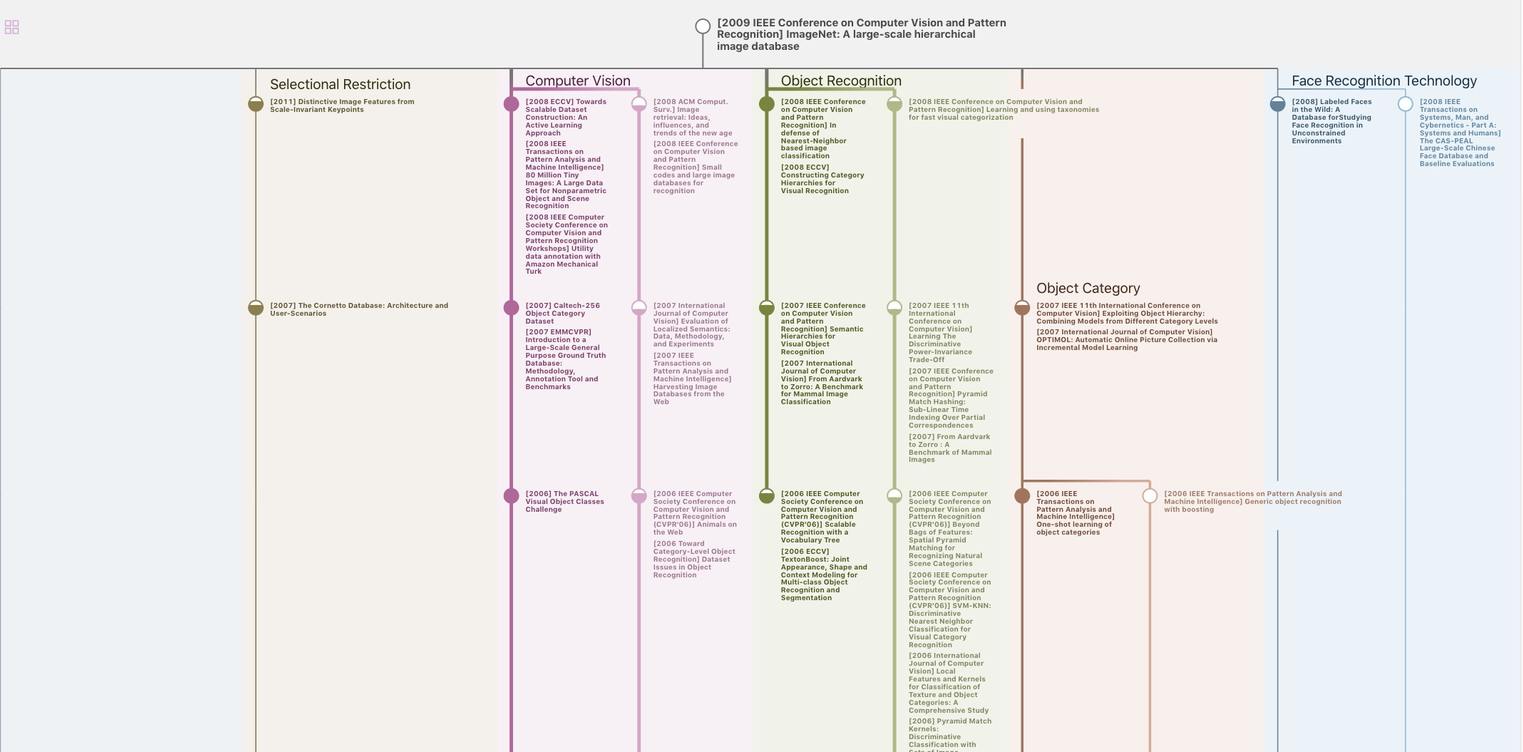
生成溯源树,研究论文发展脉络
Chat Paper
正在生成论文摘要