A benchmark dataset in chemical apparatus: recognition and detection
Multimedia Tools and Applications(2024)
摘要
Robots that perform chemical experiments autonomously have been implemented, using the same chemical apparatus as human chemists and capable of performing complex chemical experiments unmanaged. However, most robots in chemistry are still programmed and cannot adapt to diverse environments or to changes in displacement and angle of the object. To resolve this issue, we have conceived a computer vision method for identifying and detecting chemical apparatus automatically. Identifying and localizing such apparatus accurately from chemistry lab images is the most important task. We acquired 2246 images from real chemistry laboratories, with a total of 33,108 apparatus instances containing 21 classes. We demonstrate a Chemical Apparatus Benchmark Dataset (CABD) containing a chemical apparatus image recognition dataset and a chemical apparatus object detection dataset. We evaluated five excellent image recognition models: AlexNet, VGG16, GoogLeNet, ResNet50, MobileNetV2 and four state-of-the-art object detection methods: Faster R-CNN (3 backbones), Single Shot MultiBox Detector (SSD), YOLOv3-SPP and YOLOv5, respectively, on the CABD dataset. The results can serve as a baseline for future research. Experiments show that ResNet50 has the highest accuracy (99.9%) in the chemical apparatus image recognition dataset; Faster R-CNN (ResNet50-fpn) and YOLOv5 performed the best in terms of mAP (99.0%) and AR (94.5%) in the chemical apparatus object detection dataset.
更多查看译文
关键词
Deep learning,Chemical apparatus,Object detection,Image recognition,Benchmark dataset
AI 理解论文
溯源树
样例
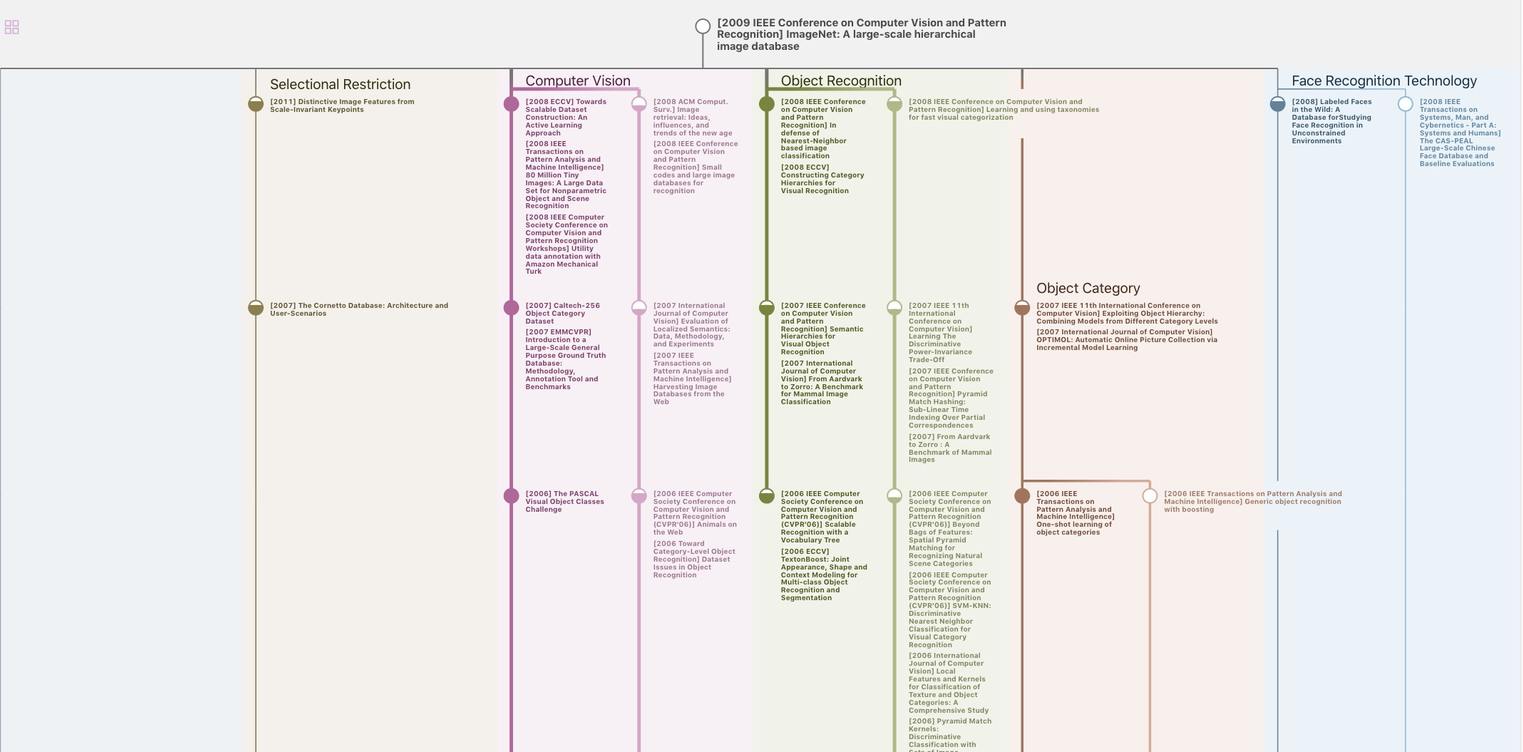
生成溯源树,研究论文发展脉络
Chat Paper
正在生成论文摘要