A decision support system in precision medicine: contrastive multimodal learning for patient stratification
Annals of Operations Research(2023)
摘要
Precision medicine aims to provide personalized healthcare for patients by stratifying them into subgroups based on their health conditions, enabling the development of tailored medical management. Various decision support systems (DSSs) are increasingly developed in this field, where the performance is limited to their capability of handling big amounts of heterogeneous and high-dimensional electronic health records (EHRs). In this paper, we focus on developing a deep learning model for patient stratification that can identify and explain patient subgroups from multimodal EHRs. The primary challenge is to effectively align and unify heterogeneous information from various modalities, which includes both unstructured and structured data. Here, we develop a Con trastive M ultimodal learning model for EHR (ConMEHR) based on topic modelling. In ConMEHR, modality-level and topic-level contrastive learning (CL) mechanisms are adopted to obtain a unified representation space and diversify patient subgroups, respectively. The performance of ConMEHR will be evaluated on two real-world EHR datasets and the results show that our model outperforms other baseline methods.
更多查看译文
关键词
Modelling unstructured and structured patient data,Application of EHRs in precision medicine,Deep learning model for patient stratification,Multimodal contrastive learning
AI 理解论文
溯源树
样例
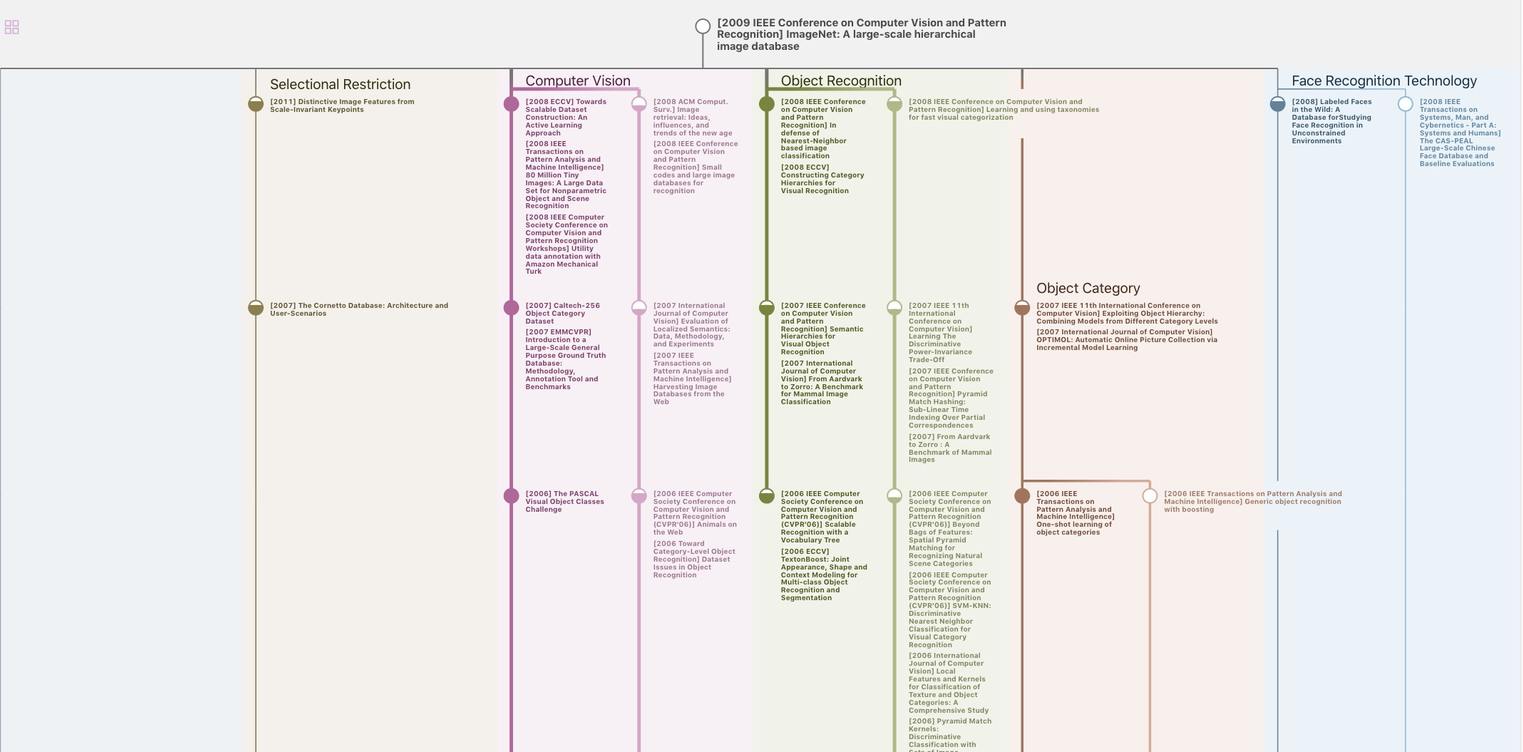
生成溯源树,研究论文发展脉络
Chat Paper
正在生成论文摘要