FasterNet-SSD: a small object detection method based on SSD model
SIGNAL IMAGE AND VIDEO PROCESSING(2023)
Abstract
In the Single Shot MultiBox Detector (SSD) model, a significant limitation arises due to the small size of many objects, leading to the extraction of limited feature information, which has significant constraints for the identification of such objects. To address this issue and enhance the model's capability in detecting small objects, we propose a novel object detection framework called FasterNet-SSD. Instead of using the VGG16 backbone network of the original SSD model, we employ the FasterNet network, which is built on partial convolution (PConv). This modification reduces computational complexity while improving the model's characterization capabilities. Furthermore, we integrate high-level features through amulti-scale fusion network to facilitate information interaction. Additionally, the feature improvement module is incorporated to enhance the representation capability and receptive field of the lower-level feature information. Experimental results demonstrate that our model achieves an impressive mean average precision (mAP) of 80.38% on the PASCAL VOC2007+2012 test set, with an input image size of 320x320. Notably, even when replacing only the backbone, our model (FasterNet-SSD-S) attains a competitive mAP of 77.96% on the PASCAL VOC2007+2012 dataset, while requiring only half of the computational complexity of the original model.
MoreTranslated text
Key words
Single Shot MultiBox Detector(SSD),Small object,Backbone network,Feature fusion
AI Read Science
Must-Reading Tree
Example
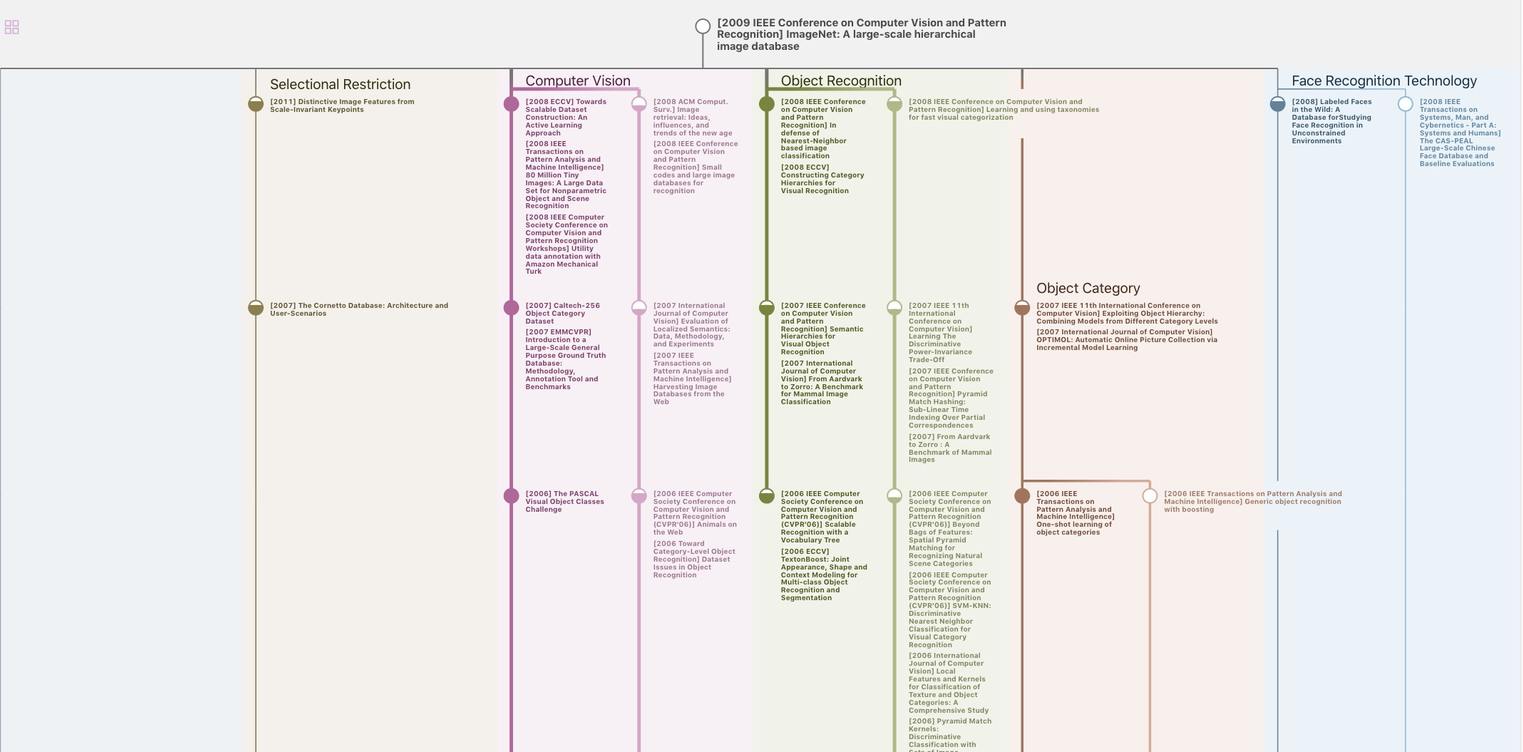
Generate MRT to find the research sequence of this paper
Chat Paper
Summary is being generated by the instructions you defined