Label propagation-based unsupervised domain adaptation for intelligent fault diagnosis
JOURNAL OF INTELLIGENT MANUFACTURING(2023)
摘要
Current unsupervised domain adaptation methods for intelligent fault diagnosis focus on learning domain-invariant representations under covariate shift assumption. However, the covariate shift assumption is usually unsatisfied when each fault class in different domains consists of multiple modes with skewed proportions, which is common in industrial scenarios. Imbalanced data from multiple modes cause the presence of within-domain class imbalance and between-domain label distributional shift. This paper introduces a novel subpopulation shift that further considers the domain shift from a subpopulation perspective, i.e., that covariate shift and label distributional shift across domains are caused by shifts in the multiple modes. To address this issue, a label propagation-based unsupervised domain adaptation is proposed based on a realistic expansion assumption. We apply the theoretical analysis of the proposed method with a bi-level optimization strategy adapted from meta-learning. Using joint optimization of a teacher model and a student model, the label propagation-based model-agnostic meta-learning (LPMAML) not only propagates supervision information from the source to the target but also adjusts the teacher’s strategy throughout the student’s learning process. To alleviate the noise caused by label distributional shift, we integrate a sampling-based alignment method that aligns the empirical label distributions across the two domains into LPMAML. Experimental results on three bearing datasets show that the proposed method has impressive generalization ability under covariate, label distributional, and subpopulation shifts. The proposed method offers consistent improvements to unsupervised domain adaptation (UDA) methods. Compared with the vanilla UDA methods, the average diagnosis accuracies of the proposed method on the label distributional shift benchmark and subpopulation shift benchmark are improved by 8.21% and 7.63%, respectively.
更多查看译文
关键词
Intelligent diagnosis,Rotating machinery,Label propagation,Subpopulation shift,Unsupervised domain adaptation
AI 理解论文
溯源树
样例
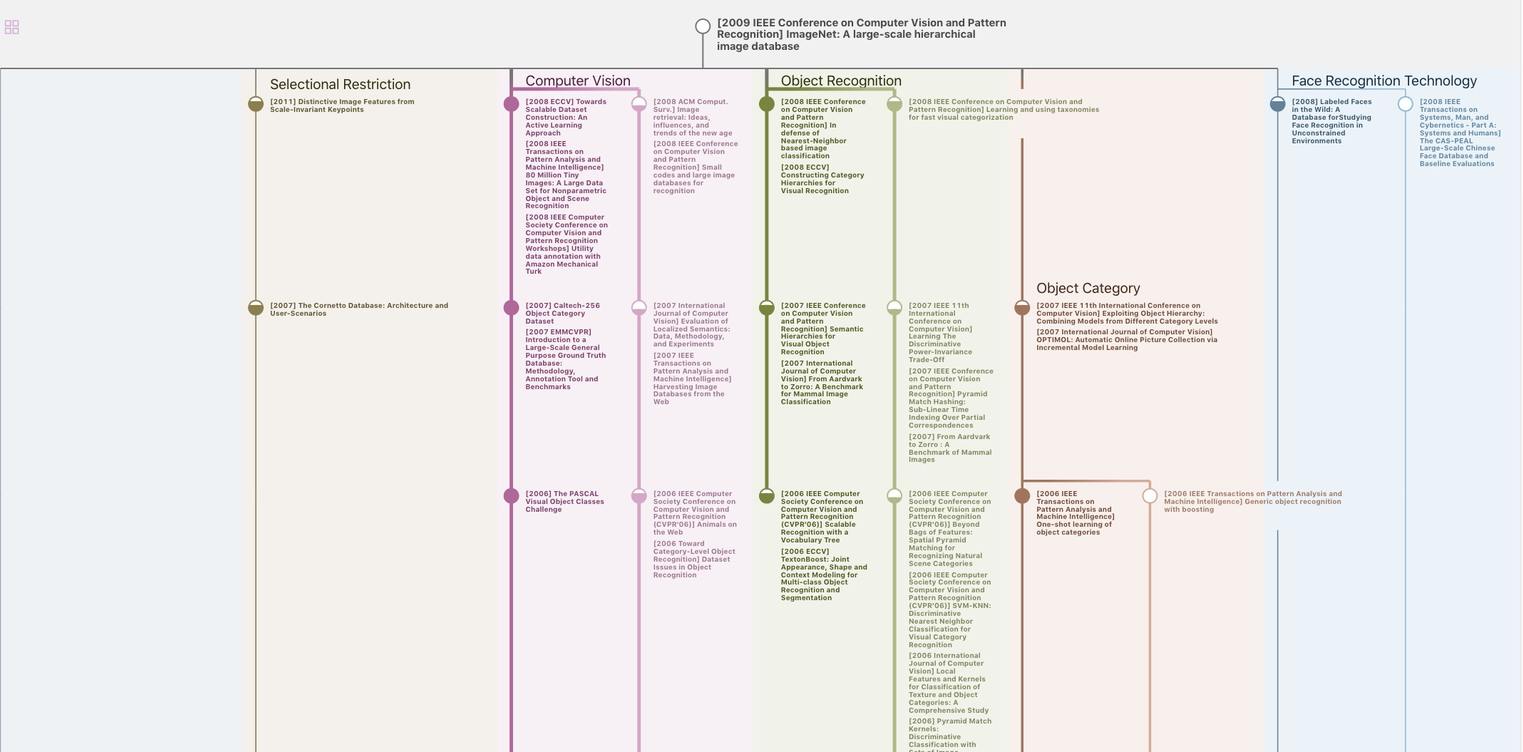
生成溯源树,研究论文发展脉络
Chat Paper
正在生成论文摘要