Prompted and integrated textual information enhancing aspect-based sentiment analysis
Journal of Intelligent Information Systems(2024)
摘要
Aspect-based Sentiment Analysis (ABSA) aims to automatically predict the sentiment polarity of the written text based on the analysis of specific aspects. By applying various pre-trained language encoders, recent studies have achieved great success in modeling aspect and context features and measuring the word-level correlations. However, the pre-trained language models (PLM) were usually employed as the feature representations generator without any task-oriented guidance. And the syntax dependency tree is also not fully utilized. Besides, simply concatenating usually fails to exploit deep semantic features from multi-source spaces and weakens the representation of context features. In this study, we propose a novel model, namely PRoGCN (Prompted RoBERTa & Graph Convolution Network), which directly tells RoBERTa the goal of the present task by inserting the task-oriented specific prompting word to the raw text. Moreover, the prompted feature representation is also utilized to help generate textual knowledge graph, and strongly enhances the syntactic feature representation. In addition, we first introduce cross attention into our study to integrate semantic representation and syntactic representation, which has been proven to be successful in implementing and fusing multi-source information. Experimental results on five publicly available ABSA datasets validate the effectiveness of our method, and the proposed method achieves state-of-the-art performance on mentioned ABSA benchmarks.
更多查看译文
关键词
Aspect-based sentiment analysis,RoBERTa,GCN,Prompt learning,Cross attention
AI 理解论文
溯源树
样例
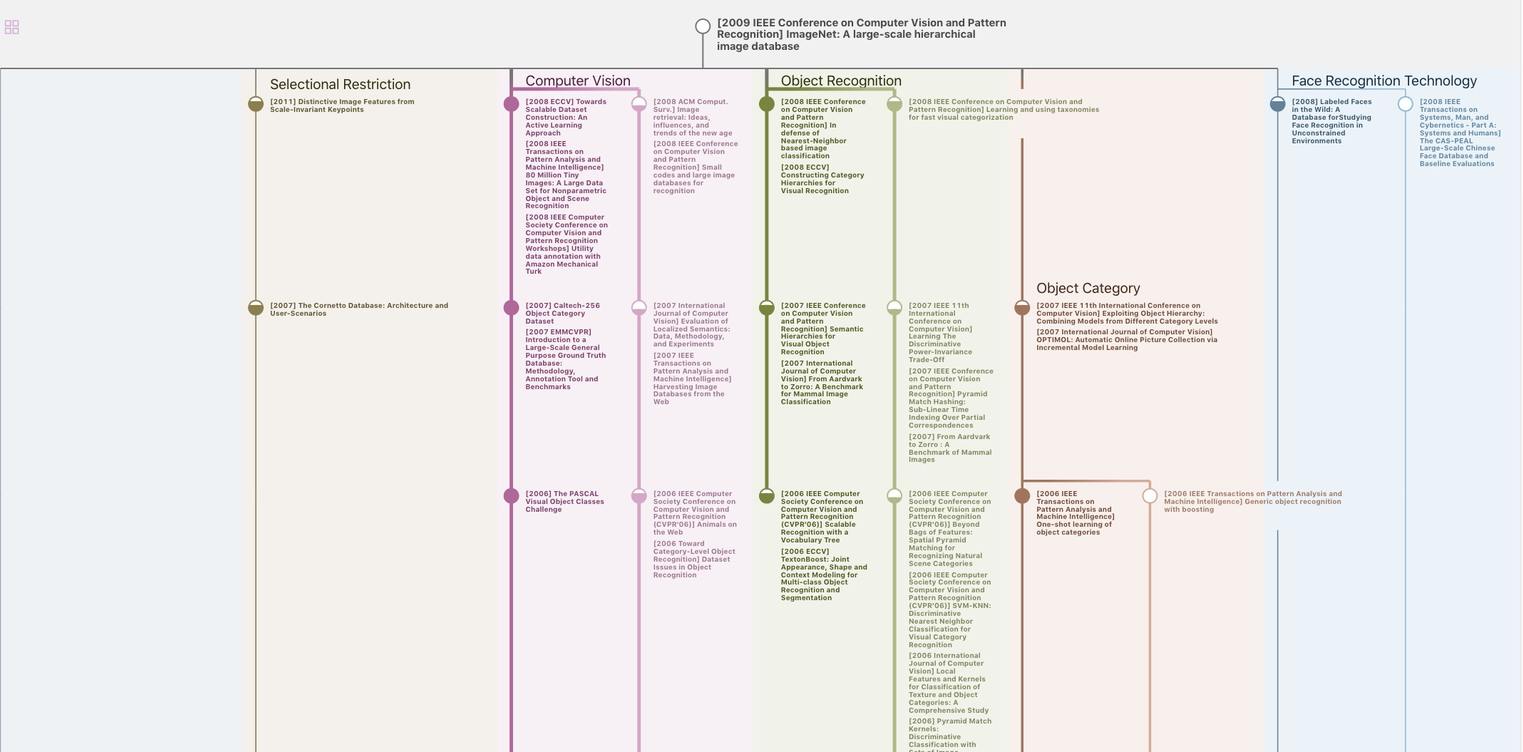
生成溯源树,研究论文发展脉络
Chat Paper
正在生成论文摘要