Baseline Defenses for Adversarial Attacks Against Aligned Language Models
CoRR(2023)
摘要
As Large Language Models quickly become ubiquitous, their security vulnerabilities are critical to understand. Recent work shows that text optimizers can produce jailbreaking prompts that bypass moderation and alignment. Drawing from the rich body of work on adversarial machine learning, we approach these attacks with three questions: What threat models are practically useful in this domain? How do baseline defense techniques perform in this new domain? How does LLM security differ from computer vision? We evaluate several baseline defense strategies against leading adversarial attacks on LLMs, discussing the various settings in which each is feasible and effective. Particularly, we look at three types of defenses: detection (perplexity based), input preprocessing (paraphrase and retokenization), and adversarial training. We discuss white-box and gray-box settings and discuss the robustness-performance trade-off for each of the defenses considered. Surprisingly, we find much more success with filtering and preprocessing than we would expect from other domains, such as vision, providing a first indication that the relative strengths of these defenses may be weighed differently in these domains.
更多查看译文
关键词
adversarial attacks,aligned language
AI 理解论文
溯源树
样例
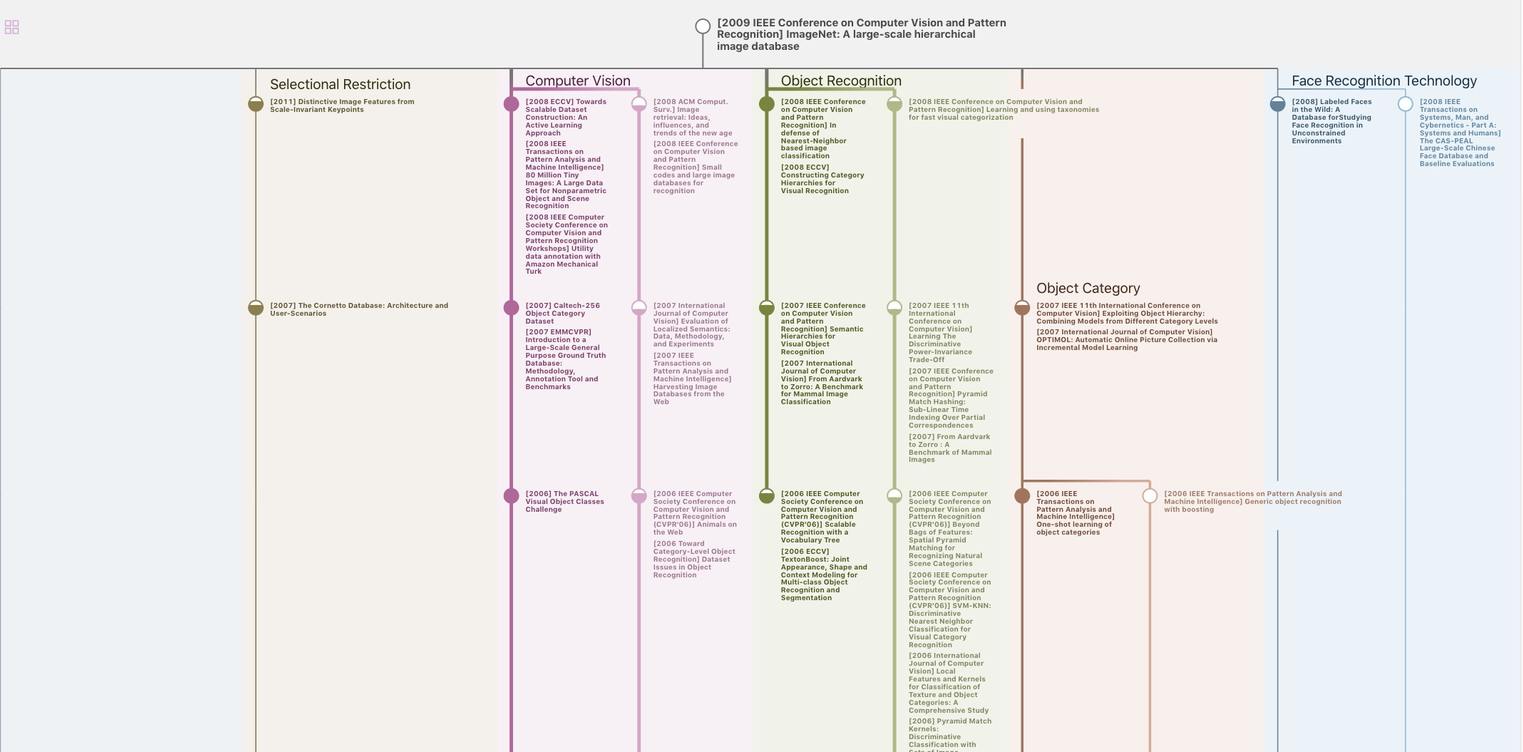
生成溯源树,研究论文发展脉络
Chat Paper
正在生成论文摘要