Robust Variational Physics-Informed Neural Networks
Computer Methods in Applied Mechanics and Engineering(2024)
摘要
We introduce a Robust version of the Variational Physics-Informed Neural Networks method (RVPINNs). As in VPINNs, we define the quadratic loss functional in terms of a Petrov–Galerkin-type variational formulation of the PDE problem: the trial space is a (Deep) Neural Network (DNN) manifold, while the test space is a finite-dimensional vector space. Whereas the VPINN’s loss depends upon the selected basis functions of a given test space, herein, we minimize a loss based on the discrete dual norm of the residual. The main advantage of such a loss definition is that it provides a reliable and efficient estimator of the true error in the energy norm under the assumption of the existence of a local Fortin operator. We test the performance and robustness of our algorithm in several advection–diffusion problems. These numerical results perfectly align with our theoretical findings, showing that our estimates are sharp.
更多查看译文
关键词
Robustness,Variational Physics-Informed Neural Networks,Petrov–Galerkin formulation,Riesz representation,Minimum residual principle,A posteriori error estimation
AI 理解论文
溯源树
样例
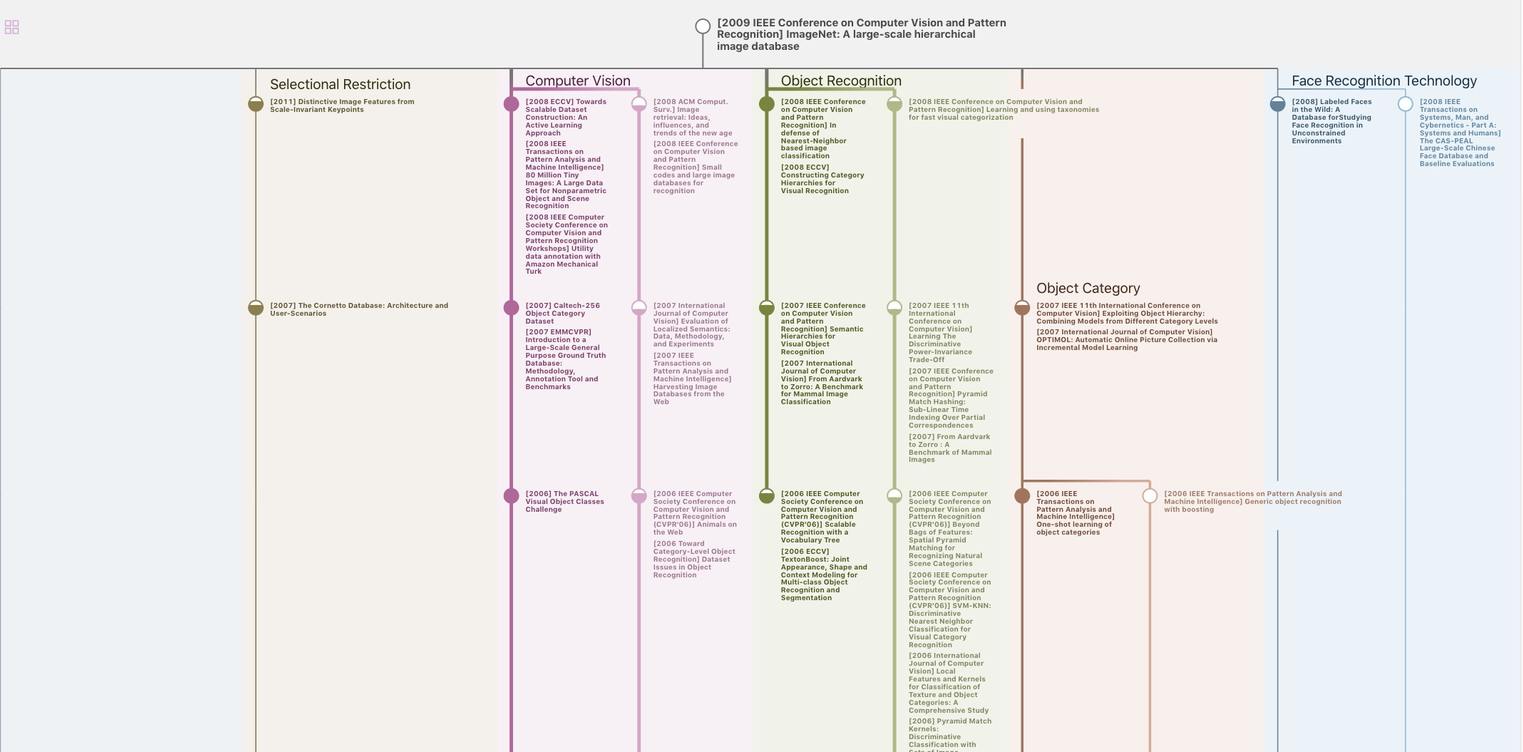
生成溯源树,研究论文发展脉络
Chat Paper
正在生成论文摘要