Robust Principles: Architectural Design Principles for Adversarially Robust CNNs
CoRR(2023)
摘要
Our research aims to unify existing works' diverging opinions on how architectural components affect the adversarial robustness of CNNs. To accomplish our goal, we synthesize a suite of three generalizable robust architectural design principles: (a) optimal range for depth and width configurations, (b) preferring convolutional over patchify stem stage, and (c) robust residual block design through adopting squeeze and excitation blocks and non-parametric smooth activation functions. Through extensive experiments across a wide spectrum of dataset scales, adversarial training methods, model parameters, and network design spaces, our principles consistently and markedly improve AutoAttack accuracy: 1-3 percentage points (pp) on CIFAR-10 and CIFAR-100, and 4-9 pp on ImageNet. The code is publicly available at https://github.com/poloclub/robust-principles.
更多查看译文
关键词
robust principles,architectural design principles,design principles
AI 理解论文
溯源树
样例
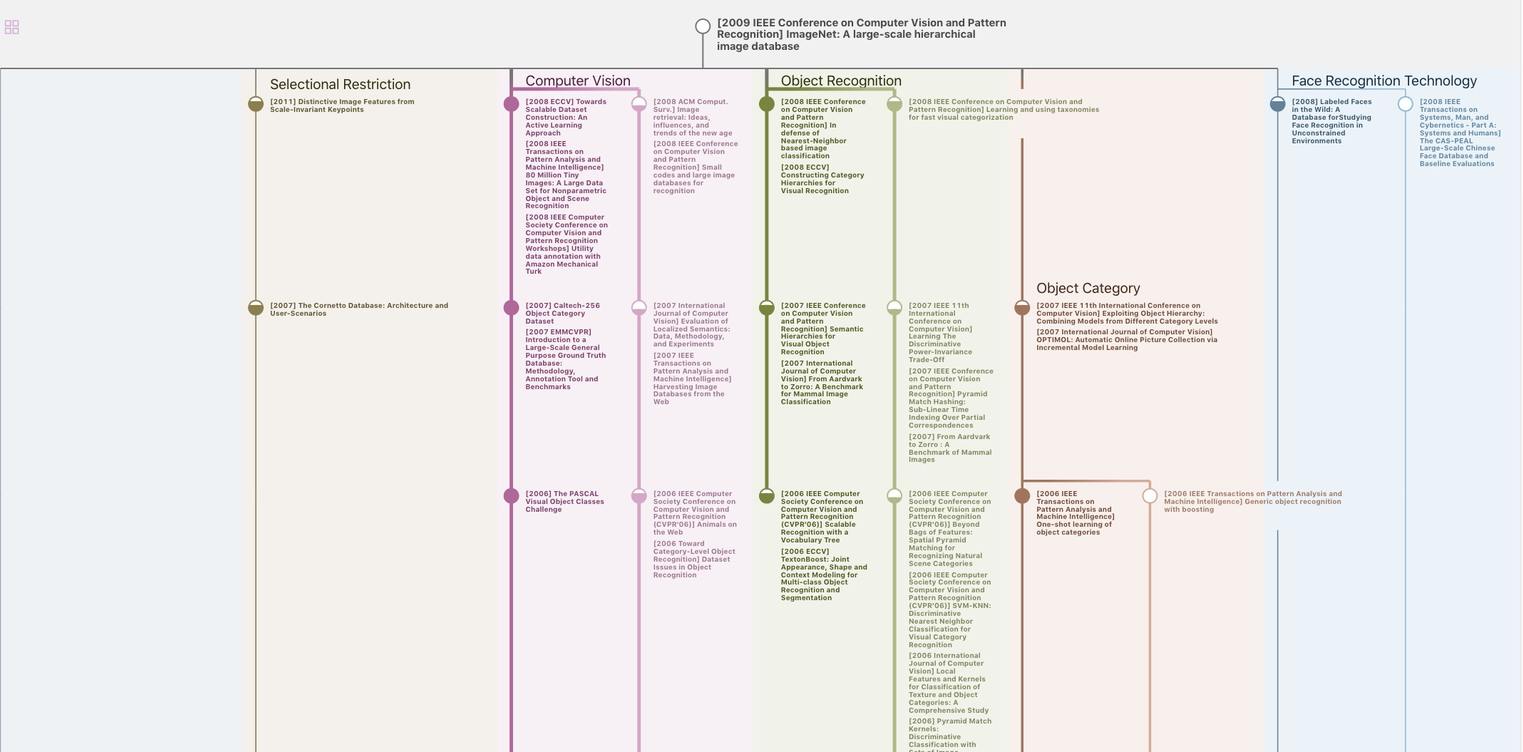
生成溯源树,研究论文发展脉络
Chat Paper
正在生成论文摘要