Dynamic Hypergraph Convolution and Recursive Gated Convolution Fusion Network for Hyperspectral Image Classification.
IEEE Geosci. Remote. Sens. Lett.(2023)
摘要
Recently, convolutional neural network (CNN) and graph convolutional network (GCN) have been used widely for hyperspectral image (HSI) classification which, respectively, specialize in characterizing the local receptive feature and structure feature. However, the existing CNN-based methods cannot learn the higher-order interactions of different spectral bands. The GCN-based methods mostly used the fixed or simple graph model for feature learning. To solve the problems, we propose the dynamic hypergraph convolution and recursive gated convolution fusion network (DHCRGCFN) for HSI classification. To learn the hidden and important relations represented in the HSI data, the dynamic hypergraph convolution network (DHCN) is designed which dynamically updates the hypergraph model and captures the global spatial information of HSI. To efficiently model the high-order interactions among the high spectral dimension, the recursive gated convolution network (RGCN) is developed for progressively capturing the interactions of spectral feature. The features extracted by the two branches are adaptively fused to achieve the complementary advantages. Extensive experiments are conducted on two public HSI datasets to demonstrate the effectiveness of the proposed DHCRGCFN.
更多查看译文
关键词
Dynamic hypergraph construction,hypergraph convolution,hyperspectral image (HSI) classification,recursive gated convolution (gⁿConv)
AI 理解论文
溯源树
样例
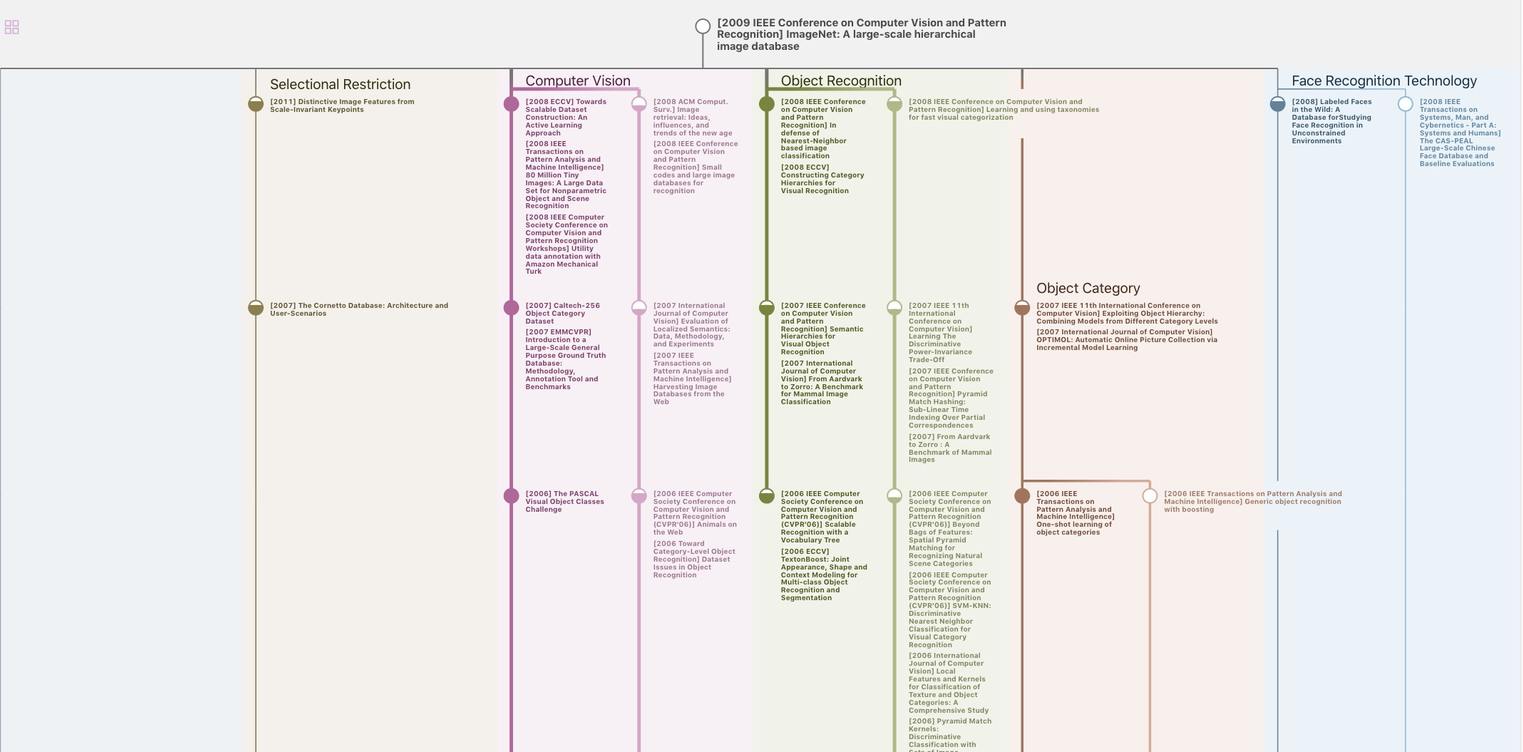
生成溯源树,研究论文发展脉络
Chat Paper
正在生成论文摘要