Selecting Information Fusion Generative Adversarial Network for Remote-Sensing Image Cloud Removal
IEEE Geosci. Remote. Sens. Lett.(2023)
Abstract
The multitemporal remote-sensing cloud removal method has improved performance, but it lacks a screening mechanism during feature fusion, simply summing and fusing features from different temporal states. This results in the inclusion of unwanted clouds and redundant feature information, hindering the restoration of the landscape under the clouds. To address this, we propose a selective information fusion generative adversarial network (SIF-GAN) for remote-sensing image cloud removal. SIF-GAN incorporates channel attention during feature extraction to capture important information in different channels and uses the selective information fusion network to assign weights to the feature information from other temporal states, selecting the crucial features for fusion. The feature of cloud-free regions in different temporal states is utilized maximally by the selection process to recover the image features under clouds. The results of the experiments show that SIF-GAN achieves superior cloud removal performance compared to other methods.
MoreTranslated text
Key words
Channel attention mechanism,generative adversarial network,remotesensing images,selective information fusion
AI Read Science
Must-Reading Tree
Example
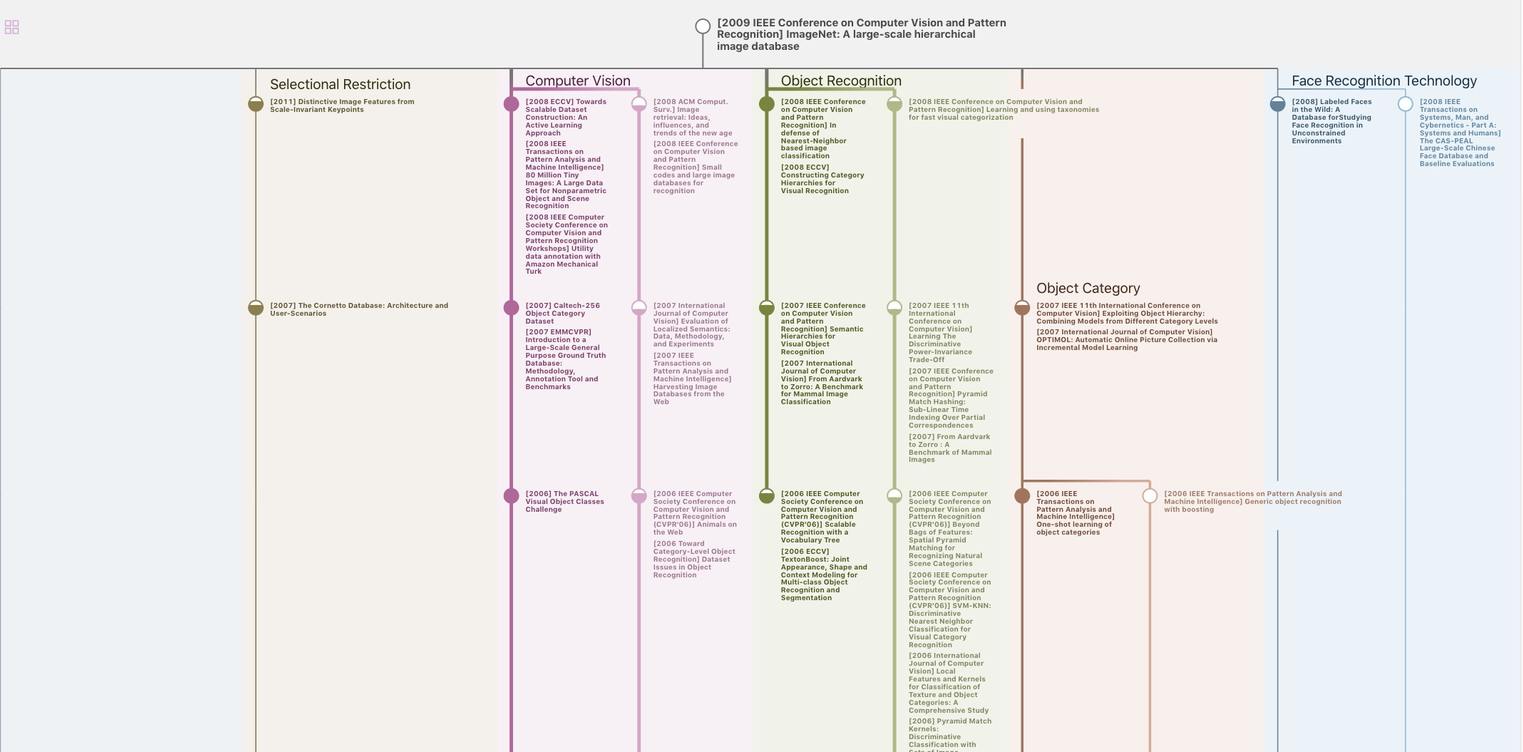
Generate MRT to find the research sequence of this paper
Chat Paper
Summary is being generated by the instructions you defined