Cybersecurity Analysis of Data-Driven Power System Stability Assessment.
IEEE Internet Things J.(2023)
摘要
Machine learning-based intelligent systems enhanced with Internet of Things (IoT) technologies have been widely developed and exploited to enable the real-time stability assessment of a large-scale electricity grid. However, it has been extensively recognized that the IoT-enabled communication network of power systems is vulnerable to cyberattacks. In particular, system operating states, critical attributes that act as input to the data-driven stability assessment, can be manipulated by malicious actors to mislead the system operator into making disastrous decisions and thus cause major blackouts and cascading events. In this article, we explore the vulnerability of the data-driven power system stability assessment, with a special emphasis on decision tree-based stability assessment (DTSA) approaches, and investigate the feasibility of constructing a physics-constrained adversarial attack (PCAA) to undermine the DTSA. The PCAA is formulated as a nonlinear programming problem considering the misclassification constraint, power limits, and bad data detection, computing potential adversarial perturbations that reverse the "stable/unstable" prediction of the real-time input while remaining invisible/stealthy. Extensive experiments based on the IEEE 68-bus system are conducted to evaluate the impact of PCAAs on predictions of DTSA and their transferability.
更多查看译文
关键词
Adversarial examples, cyber security, decision trees (DTs), physics-constrained machine learning (ML), transient stability assessment (TSA)
AI 理解论文
溯源树
样例
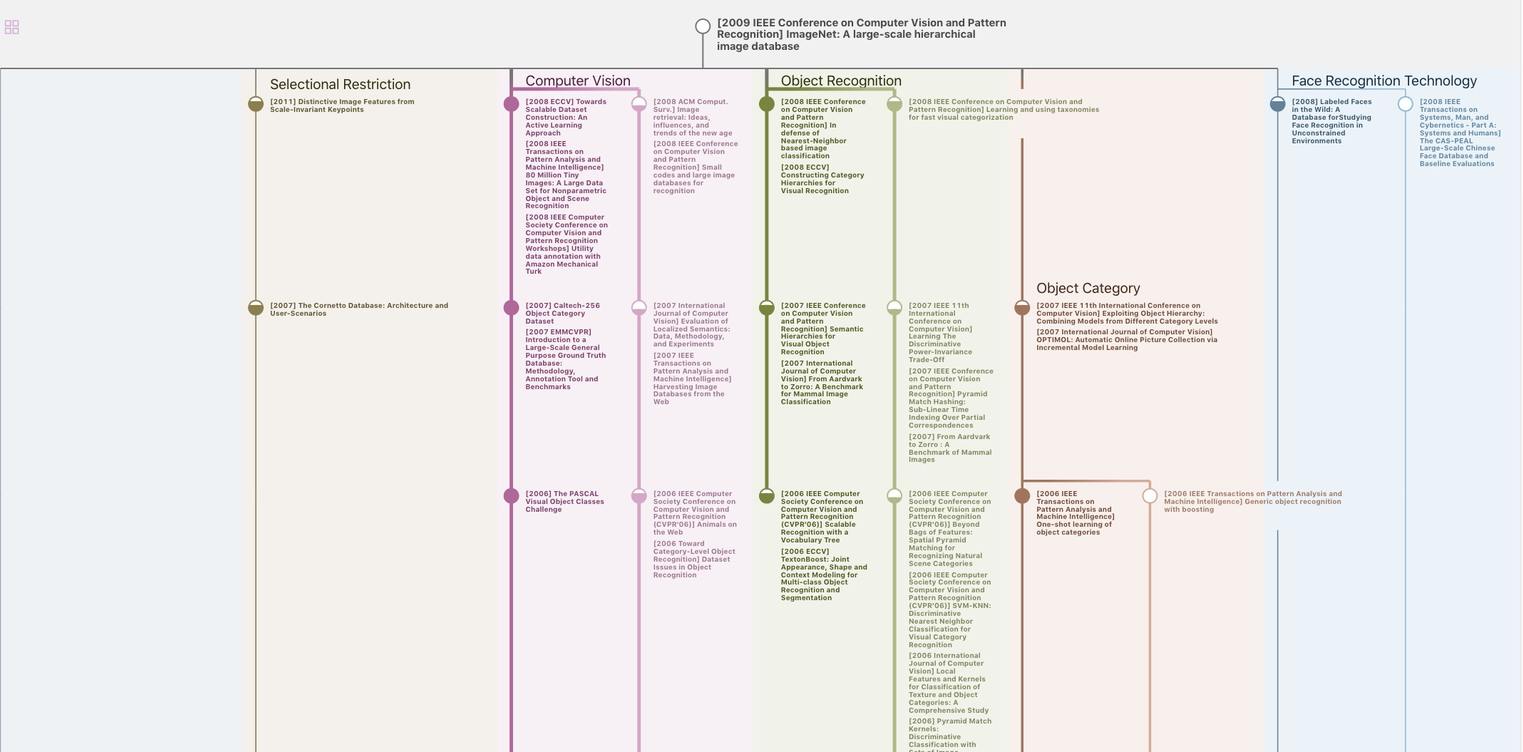
生成溯源树,研究论文发展脉络
Chat Paper
正在生成论文摘要