Heuristically Assisted Multiagent RL-Based Framework for Computation Offloading and Resource Allocation of Mobile-Edge Computing.
IEEE Internet Things J.(2023)
摘要
Mobile-edge computing (MEC) as a promising technology enables it to satisfy ever-increasing demands for low-latency and ultrareliable services. However, due to the limitations of computing capability and the dynamic network environment, it is challenging to process massive data with low latency. In this article, we consider a dynamic MEC network with a high-performance edge server, multiple time-varying channels, and multiple mobile devices. We aim to find a policy that can maximize the processing success rate of computational tasks and the fairness index of the system while minimizing the process delays. To this end, we propose a heuristic-assisted multi-agent reinforcement learning (RL)-based framework to realize the joint optimization of computation offloading and resource allocation. On the one hand, heuristic search is introduced in this framework to find a better resource allocation policy in edge servers and further assist the multiagent RL algorithm to determine offloading policy in mobile devices. On the other hand, a novel parameterized multiagent RL algorithm based on soft actor-critic (SAC) is also proposed to broaden the effectiveness and availability of the proposed framework. Simulation results of the average cumulative reward, success rate, processing delay, and fairness index fully verify the superiority of the proposed framework and algorithm for supporting this problem.
更多查看译文
关键词
mobile edge computing,computation offloading,resource allocation,rl-based
AI 理解论文
溯源树
样例
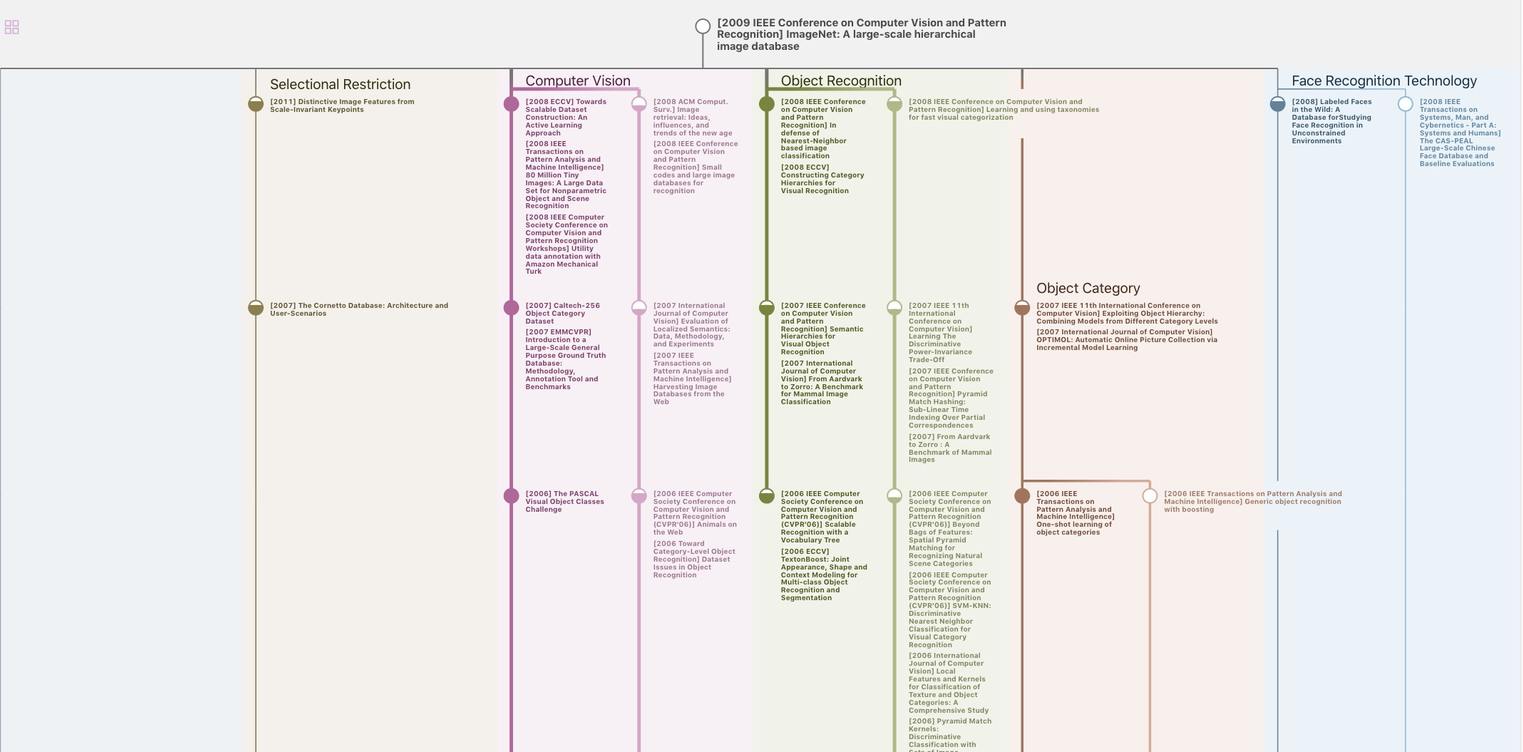
生成溯源树,研究论文发展脉络
Chat Paper
正在生成论文摘要